1. **Introduction**
Within the private equity industry, conventional investment methods are being transformed by data analytics and machine learning. Private equity firms are finding that these cutting-edge tools help them make better judgments, spot worthwhile investment possibilities, and maximize portfolio performance. Private equity experts may quickly and reliably evaluate massive volumes of data by utilizing data analytics and machine learning. This allows them to get significant insights that were previously unattainable through traditional approaches. The significant effects of machine learning and data analytics on the private equity sector are examined in this blog article.
2. **Importance of Data Analytics in Private Equity**
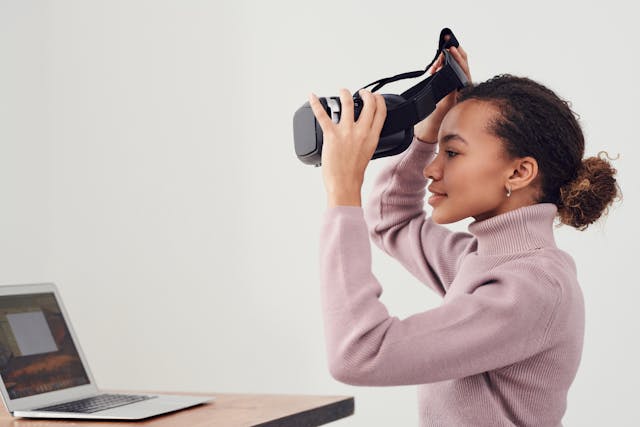
Because data analytics allows companies to use massive volumes of data to make well-informed decisions, it is essential to the transformation of the private equity industry. Using data analytics offers insightful information that informs strategic decisions in the world of private equity, where investment decisions have the power to make or break a deal. Through the utilization of data analytics tools and techniques, corporations can evaluate the performance of prospective investments, examine industry trends, and pinpoint growth and value-creation prospects inside their portfolio companies.
Beyond closing deals, data analytics is crucial to the private equity industry. Additionally, it gives businesses the ability to improve due diligence procedures, maximize operational efficiency, and successfully manage risk. Private equity professionals can find hidden patterns that could affect investment outcomes, optimize their workflows, and detect inefficiencies using data-driven research. Businesses may more properly determine the viability of possible agreements by utilizing sophisticated analytics models and algorithms, which will help them make better investment decisions and increase investor returns.
To put it simply, data analytics is essential to success in the fast-paced world of private equity. In a market that is changing quickly, its capacity to generate useful insights, reduce risks, and find new opportunities gives businesses a competitive edge. Through the strategic use of data analytics in decision-making processes, private equity firms can confidently manage unpredictable situations and direct their investments toward long-term profitability and growth.
3. **Benefits of Machine Learning for Private Equity Firms**
Private equity businesses can reap a multitude of benefits from machine learning, which has the potential to revolutionize the development and implementation of investment strategies. Its exceptional speed and accuracy in analyzing large volumes of data allow businesses to make more informed decisions, which is one of its key advantages. Machine learning uses sophisticated algorithms to find patterns and trends in information that human analysts would miss. Private equity specialists are able to acquire better insights into possible investment opportunities and hazards thanks to this comprehensive study.
Private equity organizations can benefit from machine learning by using better predictive modeling. Algorithms can more accurately predict outcomes, including shifts in market value or movements, by leveraging real-time data and past data. Businesses can proactively modify their investment strategy in response to changing market conditions because to this predictive capabilities. Routine processes like data processing and due diligence can be automated by machine learning algorithms, giving experts more time to concentrate on high-level strategic decision-making.
Through the provision of cutting-edge instruments for risk assessment and mitigation, machine learning supports risk management in the private equity industry. Algorithms can offer early alerts of possible dangers to investments by evaluating a variety of risk characteristics in real-time across markets and portfolios. Businesses can apply prompt interventions and diversification measures to safeguard their assets thanks to this proactive risk monitoring. Machine learning is being incorporated into private equity operations to improve efficiency and create a culture of data-driven decision-making, which will ultimately give the company a competitive advantage in the market.
In essence, machine learning acts as a powerful ally for private equity firms seeking to optimize their investment processes and stay ahead in an increasingly complex financial landscape.
4. **Challenges and Opportunities in Implementing Data Analytics**
Private equity firms encounter many obstacles when attempting to apply data analytics. A prevalent problem is the abundance and diversity of data sources accessible, which makes it challenging to derive significant insights. Another challenge is ensuring the quality and accuracy of the data, since inconsistent or missing data might result in inaccurate analysis and choices. The management of sensitive financial and personal data is made more difficult by privacy issues and regulatory compliance.
Notwithstanding these obstacles, private equity firms have a lot of opportunity to use data analytics well. Businesses can obtain a competitive edge through improved risk assessment, investment appraisal, and portfolio management by utilizing sophisticated analytics technologies. Algorithms for machine learning can be used to find patterns, forecast changes in the market, and improve deal sourcing tactics. Data-driven insights can be used to automate repetitive operations and streamline workflows, resulting in increased operational efficiency and cost savings.
Based on the aforementioned information, we can conclude that although there are certain problems when applying data analytics in private equity, the potential benefits greatly exceed these difficulties. Businesses that effectively get over these obstacles stand to improve their decision-making procedures, increase returns on investments, and maintain their competitive edge in a market that is becoming more and more crowded. Through astute adoption of data analytics and machine learning technology, private equity firms can seize novel prospects for expansion and enduring prosperity amidst the dynamic market milieu.
5. **Real-Life Applications of Machine Learning in Private Equity**
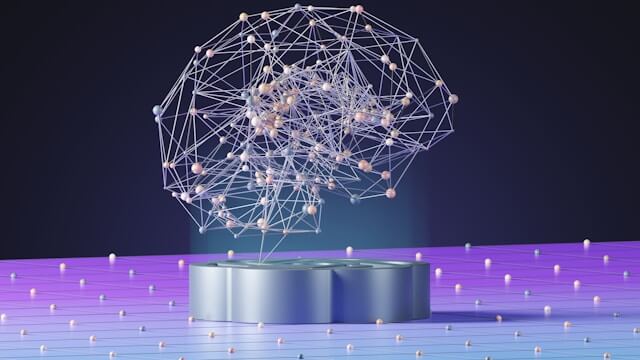
Machine learning has transformed the private equity industry by facilitating the extraction of valuable insights from large datasets, hence revolutionizing decision-making processes. Deal sourcing is one interesting use for machine learning (ML), wherein algorithms evaluate a large number of data points to identify possible investments that meet a firm's investment requirements. Private equity experts can find profitable acquisitions more quickly and with less manual labor and bias by automating this process.
Risk assessment and management are a noteworthy application of machine learning in the private equity industry. ML algorithms are capable of evaluating the possible risks associated with an investment, predicting market trends, and assessing the financial health of target organizations through advanced predictive modeling. Before completing an investment contract, this proactive method enables organizations to make well-informed judgments and minimize potential drawbacks.
A key factor in improving portfolio management in the private equity industry is machine learning. ML algorithms can optimize portfolio allocation, spot growth possibilities, and even forecast future success rates for different investments by examining past performance data and market patterns. Businesses can customize their strategies for optimal returns and minimize risks across their portfolios with this data-driven approach.ðŸ«
The way that businesses traverse the complicated world of investing has changed dramatically as a result of the incorporation of machine learning into private equity operations. Private equity professionals may achieve unprecedented levels of efficiency, accuracy, and strategic insight to improve decision-making processes and, ultimately, provide greater returns for their investors. This can be achieved by utilizing advanced analytics tools and AI-driven technology.
6. **Impact on Due Diligence Processes**
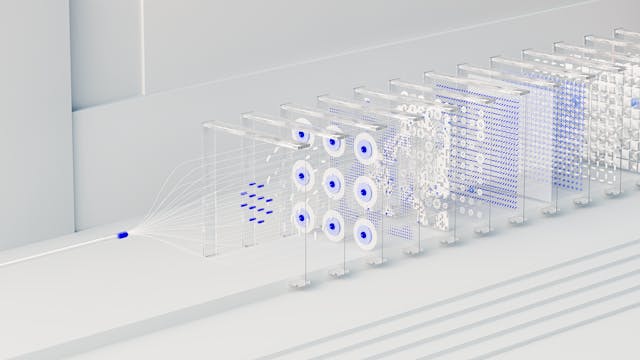
Machine learning and data analytics have had a big impact on private equity firms' due diligence procedures. Businesses can improve and streamline their due diligence processes in a number of ways by utilizing these technologies.
Data collection and analysis is one important area where data analytics has had a big influence. With the abundance of data available today, private equity firms may quickly and effectively collect, organize, and analyze data from multiple sources by utilizing sophisticated analytics tools. In addition to saving time, this enables businesses to find insightful information that might have been challenging to get with more conventional approaches.
Algorithms for machine learning are essential for increasing the precision of due diligence procedures. Businesses may more accurately analyze risks, discover patterns, and forecast outcomes by training models using historical data sets. Businesses may reduce possible risks before they have an impact on the investment and make better informed decisions about investments thanks to this predictive capability.
During the due diligence process, private equity firms can perform more thorough and in-depth investigations thanks to data analytics. Businesses can uncover patterns, correlations, and anomalies that offer important insights on the target company's performance, position in the market, and growth prospects by utilizing sophisticated statistical approaches and machine learning algorithms on huge datasets.
The method that private equity firms assess investment prospects has been completely transformed by the incorporation of data analytics and machine learning into due diligence procedures. These technologies not only increase productivity but also improve decision-making by offering more precise predictions and in-depth insights derived from thorough data analysis.
7. **Enhancing Portfolio Management with Machine Learning**
Improving Portfolio Management with Machine Learning entails using cutting-edge algorithms to streamline private equity firms' decision-making procedures. Portfolio managers can discover possible investment opportunities, accurately assess risks, and obtain insightful knowledge of market trends by utilizing machine learning (ML) approaches like risk modeling and predictive analytics.
Large volumes of data from many sources, like as financial statements, market trends, and customer behavior patterns, can be analyzed by machine learning algorithms. This gives portfolio managers the ability to base their decisions about investments on predictive analytics and real-time data, making them more knowledgeable. By finding connections between various assets and more precisely forecasting future performance, machine learning models can aid in the optimization of portfolio diversification techniques.
By helping to automate monotonous processes like data processing and analysis, machine learning enables portfolio managers to concentrate on more strategically important areas of portfolio management. Through the use of machine learning (ML) techniques, private equity companies may become more competitive in today's dynamic market by enhancing overall portfolio performance, cutting operating expenses, and making decisions more quickly.
8. **Data Security Concerns and Solutions**
Data security is a crucial consideration in the field of private equity when putting data analytics and machine learning technologies into practice. The use of large volumes of data has inherent privacy issues that need to be properly controlled to guarantee regulatory compliance and safeguard confidential data. Strong security measures must be given top priority by private equity firms in order to protect their operations from the increasing focus on data protection legislation such as the CCPA and GDPR.
Private equity businesses may use encryption techniques to safeguard private information while it's in transit and at rest in order to successfully address these privacy concerns. Putting in place access controls and routine monitoring procedures can aid in quickly identifying any illegal access or breaches. Techniques like anonymization and pseudonymization can be used to preserve personally identifiable information while enabling insightful analysis.
Creating a data security culture within the company through awareness campaigns and training initiatives is another crucial component. Best practices for managing sensitive data and upholding regulatory compliance should be taught to staff members. Frequent evaluations and audits can also assist in locating possible weak points in the current procedures and systems, allowing for early risk mitigation through corrective action.
When outsourcing some parts of the data analytics process, it is imperative to establish robust vendor management procedures. To avoid any compromise of sensitive data, private equity firms should make sure that third-party providers follow strict security standards and procedures. For vendors to be held responsible for preserving the integrity of the data they manage, contracts that specify their obligations and liabilities in regards to data security are necessary.
Furthermore, as I mentioned above, resolving data security issues in the context of private equity necessitates an all-encompassing strategy that incorporates technological advancements, efforts to comply with regulations, staff development, and vendor management procedures. Private equity firms can capitalize on actionable insights and maintain stakeholder and investor trust in this fast changing landscape by proactively minimizing risks connected with data analytics programs and emphasizing privacy protection measures.
9. **Future Trends and Predictions in the Private Equity Sector**
It is clear that data analytics and machine learning will become more important in determining the future of the private equity business and how it is shaped. The incorporation of sophisticated data analytics tools into the process of making investment decisions is a significant trend that is anticipated to persist. Through the use of these technologies, private equity companies will be able to make more intelligent investment decisions by gaining a deeper understanding of consumer behavior, market trends, and operational efficiency.
We also see substantial increase in the application of machine learning techniques to predictive modeling. With the use of these technologies, private equity investors can more successfully manage risks, predict market moves, and spot possible investment opportunities. In a dynamic market, machine learning models can offer insightful forecasts that guide strategic decision-making by utilizing both historical data and current information.
We anticipate a greater emphasis on deal sourcing and due diligence procedures that make use of AI-driven solutions. AI may improve and streamline traditional private equity procedures in a number of ways, including automated screening of target firms based on predetermined criteria, sentiment analysis on social media platforms, and natural language processing for the examination of contracts and legal documents.
Data analytics and machine learning technologies will continue to advance and be adopted at a rate that will determine the future of private equity. Businesses that adopt these technologies will find it easier to spot profitable investment opportunities, maximize portfolio performance, and eventually provide stakeholders with higher returns. We may anticipate a change in the way private equity functions as these technologies advance and become more widely available, making the sector more dynamic and effective in the future.
10. **Case Studies: Successful Implementations of Data Analytics and Machine Learning**
A number of private equity firms have effectively incorporated machine learning and data analytics to transform their decision-making and operational processes. Predictive analytics was utilized by a mid-sized private equity firm to find assets in the retail industry that were undervalued. With the help of consumer trends, competitive environments, and economic indicators, the company made well-considered investment decisions that paid off handsomely.
A noteworthy case study also concerns a sizable private equity fund that automated the examination of legal contracts as part of due diligence procedures by utilizing natural language understanding algorithms. In addition to speeding up the review process, this automation increased accuracy by highlighting possible hazards or possibilities that more typical manual assessments would have overlooked.
Machine learning algorithms were effectively used by a boutique private equity firm to enhance its portfolio management approach. The firm was able to increase overall portfolio performance and mitigate risk by proactively adjusting its exit strategy and investment allocations through the analysis of market trends, sector-specific insights, and historical performance data.
In today's competitive world, these case studies show how private equity companies may promote innovation, improve decision-making capabilities, and ultimately maximize returns by integrating data analytics and machine learning technology.
11. **Regulatory Considerations for Data-Driven Decision-Making**
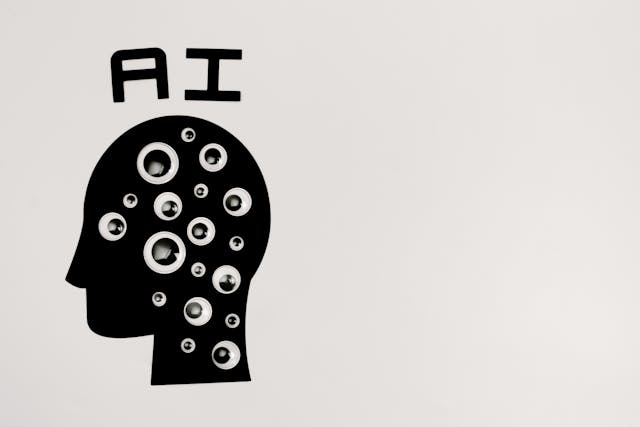
Using machine learning and data analytics gives the private equity space a revolutionary advantage. But in the midst of this revolution, it's critical to successfully negotiate the regulatory environment. When using data-driven decision-making processes in private equity investments, compliance standards are important.
Businesses need to be aware of the regulations pertaining to security, privacy, and openness of data. Handling sensitive personal and financial data necessitates compliance with laws like the CCPA and GDPR. To prevent penalties and legal ramifications, it is critical to make sure that data collecting, storage, and processing procedures adhere to these standards.
Transparency becomes critical in private equity transactions that are powered by data analytics. Regulators may mandate that companies reveal the data sources they utilize, the algorithms they employ for research, and the rationale behind their investment choices. Retaining transparent documentation and audit trails of data-driven procedures can assist companies in proving compliance and keeping stakeholders' trust.
Regulators must recognize the possible biases in these models since private equity is depending more and more on complex algorithms for decision-making. Compliance frameworks must to include methods for identifying and reducing algorithmic biases in order to guarantee impartial and moral decision-making procedures.
Additionally, as I mentioned above, handling regulatory considerations in data-driven decision-making in private equity necessitates taking proactive steps to preserve process transparency, ensure privacy regulations are followed, and effectively remove algorithmic biases. Private equity businesses can leverage technology to its full potential while reducing the risk of non-compliance by incorporating strong regulatory frameworks into their data analytics initiatives.
12.**Conclusion**
Taking into account everything that has been said thus far, we can say that the combination of machine learning and data analytics has completely changed the private equity industry. Through the provision of deeper insights, risk mitigation, and more accurate identification of profitable investment prospects, these technologies have improved decision-making processes. Private equity firms can enhance portfolio performance, streamline operations, and provide investors with greater returns by implementing data-driven methods.
In the future, data analytics and machine learning (ML) have the potential to significantly change the private equity industry. Businesses will use automation, predictive modeling, and real-time analytics as new developments push the boundaries of these tools and help them remain competitive in a changing market. Improved AI skills will make it possible to complete due diligence procedures more quickly, source deals more effectively, and implement post-acquisition value generation plans more successfully.
In a financial landscape that is changing quickly, private equity firms that want to succeed over the long term and achieve sustainable development will need to embrace data analytics and machine learning technology. These businesses can quickly adjust to market shifts, find new opportunities for value creation, and spur innovation in their investment strategies by utilizing the power of data-driven decision-making. Those who fully embrace these technology innovations will have a bright future in private equity.