1. Introduction to the concept of 'Data: When Less is More'
The idea that 'less is more' in the field of data analytics could initially seem illogical. After all, it has long been believed that greater data produces more insightful analysis and better decision-making. A rising number of experts, however, are arguing that a concentrated approach to data collecting and analysis can frequently produce more relevant results than getting lost in a sea of data. 'Data: When Less is More' is an idea that questions conventional wisdom and promotes the more intelligent and effective use of data resources. Let's investigate this fascinating concept in more detail and see how it might completely alter the way we think about data-driven processes.
2. Exploring the benefits of minimalistic data collection and analysis
When it comes to data analysis, sometimes less really is more. Although large datasets are frequently preferred, adopting minimalistic ways to data collecting and analysis can have a number of advantages. Researchers and analysts can improve efficiency, cut down on noise and superfluous information, and obtain insights more quickly by concentrating on the most important data points.
Enhanced clarity is a major benefit of minimalist data collection. By focusing on gathering only the most relevant data for their analysis, researchers can prevent information overload and unnecessary details that could skew their results. They may more readily find significant patterns and links in the data thanks to this targeted approach, which produces more understandable interpretations and useful insights.
Working with minimalist data analysis encourages a more flexible and agile process. Working with smaller datasets allows analysts to test theories effectively, iterate quickly, and make necessary improvements without being overtaken by data. Their ability to adapt quickly to evolving study inquiries and commercial requirements promotes creativity and enhances decision-making procedures.
Enhanced data quality is an additional advantage of minimalist data techniques. Organizations may guarantee that the data they collect is accurate, dependable, and pertinent to their goals by placing a higher priority on quality than quantity. By placing a strong emphasis on high-quality data, they can improve the integrity of their analyses and lower the possibility that their conclusions would contain biases or inaccuracies.
In summary, by placing an emphasis on clarity, agility, and quality in the research process, adopting a minimalist approach to data collecting and analysis can produce more effective results. Simplicity-minded researchers are better equipped to swiftly extract significant insights and make evidence-based conclusions rather than getting lost in a sea of unimportant data.
3. Case studies exemplifying successful outcomes with a lean data approach
Case Study 1: E-commerce Company An e-commerce company streamlined its data collection process by focusing only on key metrics like customer demographics, purchase history, and website behavior. By reducing unnecessary data points, they optimized their marketing campaigns and improved product recommendations. This approach led to a significant increase in conversion rates and customer engagement.😎
Case Study 2: Healthcare Provider
By combining patient data into a single database, a healthcare provider made the switch to a lean data strategy. Prioritizing pertinent treatment plans, medical histories, and billing information improved patient care coordination and reduced administrative burden. As a result, the team was able to spend more time helping patients instead of sifting through a mountain of data.
Case Study 3: Tech Startup
A digital business collected targeted user input and behavioral trends without being bogged down in unnecessary data, which transformed the company's approach to product development. Their rapid identification of areas for improvement and timely resolution led to an increase in the efficiency of their iterative design process. The company was able to keep ahead of competitors in the market by iterating on product features quickly because to its agile methodology.
Every one of these case studies demonstrates how adopting a lean data approach can result in observable advantages including better decision-making, more operational effectiveness, and greater company agility. Organizations can uncover insightful information that spurs growth and innovation by prioritizing quality over quantity when it comes to data gathering and analysis.
4. Strategies for minimizing data overload in decision-making processes
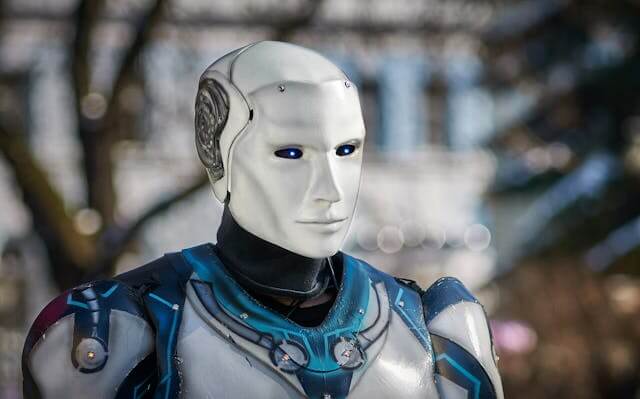
Several techniques can help to expedite the decision-making process when faced with data overload. Make sure the data is relevant first by concentrating on important metrics and details that are pertinent to the current decision. Next, formulate specific goals and inquiries to direct the gathering of data and avoid the accumulation of superfluous information. Using visual aids like graphs and charts can help make complex data sets easier to understand. Automating data processing and effectively highlighting patterns or trends can be achieved by utilizing advanced analytics technologies such as machine learning algorithms. Finally, keeping a small but useful data collection for decision-making requires regular evaluations of data sources and modifications to filtering parameters.
5. The role of artificial intelligence in optimizing limited data sets
Optimizing small data sets is a critical function of artificial intelligence. AI systems are able to extract maximum value from tiny datasets through methods like generative models, data augmentation, and transfer learning. Through transfer learning, models can perform better on smaller datasets by utilizing knowledge from bigger, pre-existing ones. By significantly altering already-existing data points, data augmentation techniques generate new ones, hence expanding the dataset's effective size. Synthetic data can be produced by generative models, like GANs, and added to real data to improve training.
AI algorithms that are skilled at managing uncertainty in small datasets include ensemble learning and Bayesian approaches. When working with sparse datasets, Bayesian techniques offer a framework for updating beliefs based on observed data and incorporating prior information. To increase accuracy and generalization, ensemble learning integrates predictions from several models. This technique is especially useful for small, noisy datasets.
Active learning is one of the AI-driven methods that gives priority to gathering the best training examples possible from a small amount of data. Active learning minimizes the requirement for enormous amounts of labeled data while optimizing the learning process and maximizing model performance by intelligently choosing which samples to label or annotate next. When data labelling is costly or time-consuming, this iterative method works very well.
To put it simply, artificial intelligence is a useful tool for gleaning important information from little amounts of data. AI can turn scarcity into an advantage by optimizing the use of existing data resources and pushing the envelope of what is possible even with restrictions on incoming information. This is done through sophisticated algorithms and creative approaches.
6. Ethical considerations surrounding reducing data usage in technological advancements
The debate over how to reduce data usage in technological breakthroughs must take ethics into account. Making sure that reducing data collecting doesn't jeopardize user privacy or provide unfavorable results is one of the main problems. Achieving equilibrium between leveraging the advantages of data-driven technologies and protecting individuals' privacy rights is crucial. Businesses need to be open and honest about how they use data, and they should make sure that cutting back on data usage doesn't unfairly harm particular groups or support discrimination.
Reducing data usage makes accountability and responsibility more difficult to understand. It could be difficult to guarantee the precision and dependability of algorithms and forecasts if there is a decrease in data collection. Businesses must take precautions to reduce any potential dangers and carefully assess how lowering data inputs could influence the integrity of their systems. The wider societal effects of reducing data usage, such as those on innovation, economic expansion, and service accessibility, are also ethically relevant.
The possible trade-off between privacy protections and tailored experiences is another ethical factor to take into account. Restricting data gathering can improve privacy, but it may also reduce the kind of individualized services and suggestions that consumers have been accustomed to. Businesses must morally strike this fine balance by investigating alternate strategies like differential privacy or anonymization techniques in order to provide individualized experiences without jeopardizing user privacy. Reducing data usage raises ethical issues that need for thoughtful planning and proactive steps to guarantee that technical innovations serve society while respecting core principles like justice and privacy.
7. Practical tips for implementing a "less is more" mentality in data-driven initiatives
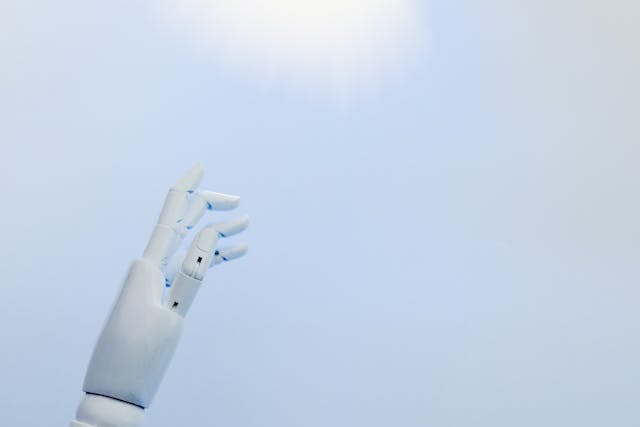
Implementing a "less is more" mentality in data-driven initiatives can lead to more focused and impactful outcomes. Here are some practical tips to help you streamline your approach:
1. Establish definite goals: Establish specific goals at the outset for your data endeavors. Pay attention to the things that really important for your business goals and refrain from gathering unnecessary data.
2. **Quality Above Quantity:** Put the quality of the data above its quantity. Focus on getting high-quality, pertinent data that immediately answers your main demands and queries rather than acquiring a ton of data.
3. **Synchronous Data Audits:** Audit your current data sources and procedures on a regular basis. To make sure you are dealing with the most recent and pertinent data, find and remove any unnecessary or out-of-date information.
4. **Simplify Data gathering:** Reduce the number of variables in your data gathering by concentrating exclusively on the key performance indicators that support your goals. Steer clear of gathering extraneous data items since they may add noise and complexity to your research.
5. Emphasize Crucial Metrics: Determine which key performance indicators are most important for gauging achievement and forming wise decisions. You can avoid becoming bogged down in a sea of superfluous or irrelevant data items by focusing on these essential indications.
6. Employ Automation Instruments: To make data gathering, processing, and analysis processes more efficient, make use of automation techniques and technology. You can ensure consistency in your data operations, save time, and minimize manual errors with automation. 😏
7. **Invest in Data Visualization:** Make use of powerful data visualization strategies to communicate important insights succinctly. Without being overtaken by details, stakeholders can more easily comprehend trends, patterns, and outliers with the use of visualizations.
8. Adopt Data Governance: Establish strong data governance procedures to guarantee that the appropriate individuals have access to the appropriate data at the appropriate moment. To keep data trustworthy and integrity, set explicit guidelines for data management, security, privacy, and compliance.
9. **Progressive Method:** Take an iterative approach to your data endeavors by putting ideas to the test, getting input, and making any adjustments to your plans after you've started small. By adopting an agile mentality, you can continuously enhance your decision-making processes based on real-time data and learn from each iteration.
You may simplify your workflow, improve results, and eventually realize the full potential of your company's data assets by heeding these helpful suggestions for applying a "less is more" mindset to your data-driven projects.
8. Discussing potential challenges and misconceptions related to minimal data usage
Regarding the use of limited data, there are a number of possible obstacles and misunderstandings that may occur. One prevalent fallacy is the idea that increased data always produces superior outcomes. Accuracy can occasionally be increased by having additional data, although this is not always the case. More significant insights can often be obtained from a smaller, carefully selected dataset than from a larger, noisier one.
The concern that by using fewer data, crucial information will be missed is another difficulty. However, this risk can be reduced with good data preprocessing and selection. When working with small datasets, it's critical to prioritize quality over quantity and make sure the data being used is pertinent to and indicative of the issue at hand.
Some people could think that using the least amount of data equates to oversimplifying the issue or model. In actuality, performance may be optimized even with sparse data by utilizing sophisticated approaches like regularization, feature engineering, and model selection. When working with small datasets, it's critical to find a balance between complexity and simplicity to prevent overfitting or underfitting.
Persuading team members or stakeholders that using less data is effective may present difficulties. To allay doubts and foster confidence in this process, it can be helpful to present the efficiency advantages, illustrate previous accomplishments using approaches that required little data, and offer concise justifications.
In summary, although there may be obstacles and misunderstandings surrounding little data utilization, a deliberate approach that prioritizes quality, relevance, and optimization strategies can result in insightful findings. Through proactive resolution of these challenges, companies can successfully leverage minimal data in their decision-making procedures.
9. Examining how focusing on quality over quantity can enhance data accuracy and relevance
When it comes to data gathering and analysis, quality over quantity can greatly improve accuracy and relevance. Organizations may guarantee the accuracy and dependability of the information they need to make decisions by placing a high priority on the quality of data inputs. Better results and more informed decision-making result from reliable data. Businesses may concentrate on what really matters when there is no noise from inaccurate or unnecessary information due to the presence of high-quality data.
Sustaining data relevance also benefits from prioritizing quality over quantity. In comparison to a larger dataset rife with errors and noise, a smaller, high-quality dataset frequently offers more useful insights. For inferences to be significant and for patterns to be recognized as potential drivers of strategic efforts, pertinent data is necessary. Organizations can make more significant decisions and streamline their operations by concentrating on gathering only the most accurate and pertinent data points.
Putting quality first when gathering data encourages effective analysis. Organizations can more efficiently allocate resources toward gaining insights and putting strategies based on trustworthy data when they have access to high-quality data since they don't have to spend as much time cleaning, organizing, and interpreting it. Because of their increased agility in decision-making, these efficiency levels can offer firms a competitive advantage in the fast-paced world of today.
Additionally, as I mentioned previously, companies position themselves for success by guaranteeing the precision, applicability, and effectiveness of their decision-making procedures when they prioritize quality over quantity when it comes to data gathering and analysis. Putting in the time and effort to gather high-quality data will eventually pay off in the form of greater results, enhanced tactics, and a more dominant position in the market.
10. Future trends and emerging technologies supporting a streamlined approach to data processing
Future developments in fields like artificial intelligence (AI) and machine learning are expected to completely transform how we handle data. These state-of-the-art instruments can facilitate data processing by automating operations that would typically call for human involvement. Large volumes of data may be rapidly parsed by AI algorithms, which can then extract significant insights and patterns at a speed and scale that is not achievable for humans alone.
Data processing can now occur closer to the source thanks to advancements in edge computing, which eliminates the need to send massive amounts of unprocessed data to centralized servers for analysis. By keeping critical information localized, this improves data security and privacy while simultaneously cutting down on processing time.
The development of blockchain technology is changing the safe storage and access of data. Blockchain is perfect for sectors like healthcare and banking where data integrity is crucial because of its decentralized structure, which guarantees that data is visible and impervious to manipulation. This technology offers a safe and effective means to record transactions and track changes over time, which could significantly streamline operations involved in data processing.
As previously said, the key to efficient data processing in the future is to fully utilize edge computing, blockchain, AI, and machine learning capabilities. Businesses can streamline operations, enhance decision-making procedures, and obtain a competitive advantage in the quickly changing digital landscape of today by utilizing these advancements.
11. Impact of minimalistic data practices on business efficiency and innovation
Minimalistic data methods can significantly influence the inventiveness and efficiency of businesses. Businesses can simplify their operations and decision-making by concentrating on gathering only the most important data that directly advances their objectives. This strategy lessens the workload associated with handling enormous volumes of redundant or irrelevant data, enabling businesses to function more quickly and efficiently.
Minimalistic data practices can result in reduced handling complexity, faster data processing times, and cheaper storage costs. Businesses can respond to market trends or client requests more quickly and correctly when they have a leaner dataset, which allows for faster information analysis. Companies may be able to gain a competitive advantage in fast-paced sectors where accuracy and speed are essential thanks to this enhanced agility.
Organizations that use minimalist data procedures are more likely to have an innovative culture. Companies may better spot patterns, find significant correlations, and produce actionable knowledge from their data by focusing on important metrics and insights. This targeted strategy fosters innovative problem-solving and gives teams the ability to make defensible judgments based on novel insights drawn from small but significant data sets.
Adopting minimalistic data techniques helps firms maximize value from minimum inputs, which in turn spurs innovation and improves operational efficiency. Companies may drive strategic objectives with more assurance, optimize resources, and push the frontiers of what is possible through focused insights and analytics by prioritizing quality over quantity in their data strategies.
12. Conclusion summarizing key takeaways and emphasizing the importance of strategic data utilization
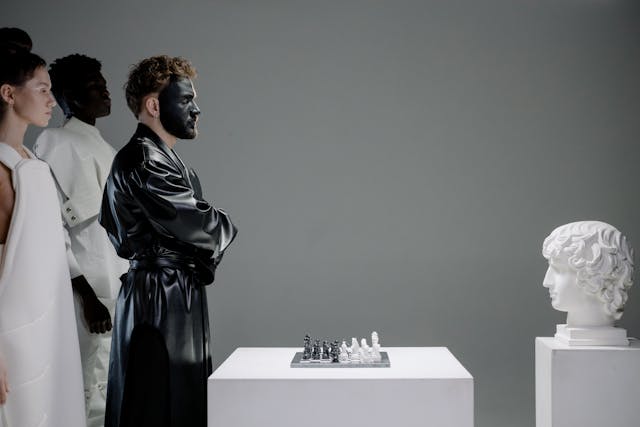
After putting everything above in order, we can say that the idea of "less is more" when it comes to data emphasizes how important it is to use data strategically. Businesses can obtain meaningful insights that support well-informed decision-making by prioritizing quality over quantity. Adopting a focused strategy for gathering and analyzing data maximizes the value produced from data assets overall while also decreasing noise and increasing efficiency. To fully realize the potential of their data, organizations should thus give top priority to improving the quality of their data sources, optimizing their workflow, and utilizing analytics tools efficiently. In today's data-driven world, strategic data use provides the road for sustainable growth and competitive advantage.