1. Introduction to Data Analysis in Business Efficiency
Because data analysis may extract useful insights from unprocessed data, it is essential for improving corporate efficiency. In order to extract significant patterns and trends, it entails the processes of data collection, cleansing, transformation, and modeling. This helps companies to find growth possibilities, streamline operations, reduce risks, and make well-informed decisions.
It is impossible to overestimate the significance of data analysis for corporate efficiency in today's data-driven world. Organizations can gain a competitive edge by forecasting trends, understanding customer behavior, driving innovation, and increasing operational performance by utilizing advanced analytics tools. Businesses may improve efficiency, streamline processes, allocate resources wisely, and eventually accomplish their strategic goals with the help of data analysis.
2. Types of Data Sources for Business Analysis
Businesses can use both internal and external data sources as sources of data for business analysis. Information obtained from within the company, such as client databases, financial reports, and sales records, is referred to as internal data. The analytics team of the business can typically access and handle this kind of data more readily.
External data, on the other hand, originates from outside sources such as government databases, social media feeds, and market research reports. Although this data may be unstructured and need further processing to extract useful information, it can offer insightful information about customer behavior and market trends.
The structure of data sources can also be used to group them. Information arranged according to a predetermined format, such spreadsheets or relational databases, is referred to as structured data. It is simple to analyze this kind of data using conventional methods and instruments.
Unstructured data, on the other hand, does not fit into a tidy, structured manner. Social media posts, photos, videos, and text documents are a few types of unstructured data. Advanced methods like machine learning and natural language processing (NLP) are needed to analyze this kind of data in order to find patterns and insights that might help a business's decision-making process.
3. Key Data Analysis Techniques for Business Efficiency
Using data analysis to improve corporate efficiency requires the application of several critical approaches. Summarizing historical data is a key component of descriptive analytics, which helps explain prior performance. Digging further, diagnostic analytics pinpoints the reasons behind particular results or patterns. Prescriptive analytics suggests activities to maximize results, but predictive analytics predicts future trends based on data patterns.
Using tools and strategies for data visualization is another crucial component. Graphs, charts, and dashboards are examples of visual representations that make complex datasets easier to comprehend and more insightful. These technologies help decision-makers identify trends, patterns, and outliers more quickly and easily while also making data interpretation simpler.
Businesses may use data analysis to make well-informed decisions and improve overall efficiency across a range of organizational functions by implementing these strategies into their daily operations.
4. Implementing Data-driven Decision Making in Business
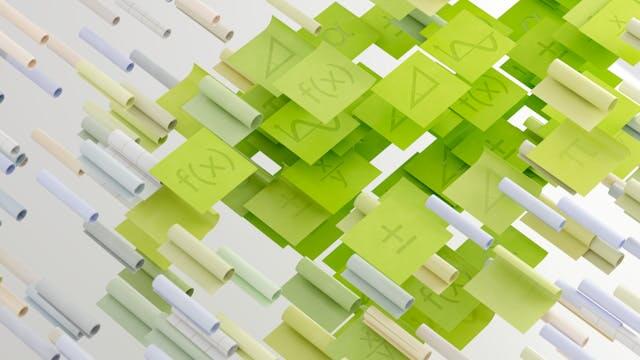
In the current competitive market, it is imperative for businesses to use data-driven decision making. The first step in successfully incorporating data analysis into decision-making processes is to identify key performance indicators (KPIs) that correspond with your company's objectives. Prior to analysis, gather pertinent data from a variety of sources and confirm its quality and accuracy.
Subsequently, employ data visualization tools to communicate insights to stakeholders in a comprehensible manner. Employees that receive excellent training on data interpretation and utilization will help to foster a culture that supports data-driven decision making. Keep your data strategy up to date and reviewed on a regular basis to keep up with shifting company requirements and industry trends.
Case studies are effective tools for demonstrating how data-driven decision making may be implemented successfully. For instance, Company X saw a 20% increase in customer retention rates following the use of consumer data to analyze purchase trends. Another case study can show how Company Y used real-time data analytics in their supply chain management to boost operational efficiency by 15%.
By implementing these strategies and learning from successful case studies, businesses can harness the power of data analysis to drive informed decisions and enhance overall efficiency.
5. Measuring the Impact of Data Analysis on Business Efficiency
Optimizing operations requires measuring the impact of data analysis on business efficiency. Data analysis efficacy can be measured using key performance indicators (KPIs) like customer happiness, revenue growth, and productivity metrics. Businesses can measure the effect of their analytical efforts and make well-informed decisions by monitoring these KPIs.
In order to propel initiatives for continuous improvement grounded in data insights, companies must establish a feedback loop. This cycle entails data analysis, trend or improvement area identification, change implementation, and outcome measurement. Businesses may quickly adjust to changes in the market and streamline procedures to increase productivity and competitiveness by using this iterative method. In the fast-paced business world of today, continuous improvement based on data-driven choices is crucial.
6. Challenges and Solutions in Data Analysis for Business Efficiency
Resolving problems with data quality is essential to guaranteeing that business decisions are accurate. Missing values, duplications, and inconsistent formats are common problems. Businesses can address these problems by putting in place procedures for validating data, defining precise guidelines for data entry, and using data cleaning tools to improve the quality of their datasets. Maintaining high-quality data for analysis also requires routinely checking and updating data quality measures.
Implementing data analysis successfully in enterprises requires overcoming organizational opposition to change. Fear of the unknown, a lack of knowledge about the advantages of using data analytics, or worries about job security are common causes of resistance. Organizations should concentrate on developing a culture that encourages learning and innovation in order to overcome this. All stakeholders' buy-in can be promoted and opposition can be reduced by giving staff moving to new systems or procedures sufficient training and support.
Businesses may fully utilize data analysis to drive efficiency and make educated decisions that improve their bottom line by proactively addressing data quality concerns and overcoming opposition to change within their companies. 📑
7. Emerging Trends in Data Analytics for Enhanced Business Efficiency
Staying ahead in the fast-paced corporate environment of today requires utilizing the potential of new developments in data analytics. Applications of machine learning (ML) and artificial intelligence (AI) have emerged as essential resources for companies looking to increase productivity. These technologies enable businesses to quickly make well-informed decisions, automate operations, and analyze enormous volumes of data to find insightful information.
Another revolutionary development in the pursuit of operational excellence is real-time analytics. Businesses may instantly get data from several sources by integrating Internet of Things (IoT) devices. Because of this real-time data flow, decisions may be made more quickly and proactively using the most recent information available. IoT integration gives analytics procedures a competitive edge by streamlining operations and quickly spotting opportunities.
8. Ethical considerations in Data Analysis for Business Efficiency
Responsible practices in the field of data analysis for corporate efficiency are greatly influenced by ethical issues. Data security and privacy issues are critical because companies need to keep their consumers' trust by protecting sensitive data. To reduce the risks of data misuse or unauthorized access, it is imperative to implement strong data protection measures and comply with all rules.
Upholding ethical norms in data analysis necessitates ensuring fairness and transparency in decision-making processes. Companies should work to prevent decision models and algorithms from having biases that could produce unfair results. Open communication regarding the gathering, processing, and application of data can strengthen stakeholder trust and improve responsibility, promoting an integrity-conscious culture inside the company.
Businesses can use data analysis ethically to safeguard their own interests and those of their clients by addressing privacy issues, improving data security protocols, and encouraging equity and openness in decision-making procedures.
9. Training and Upskilling Employees for Effective Data Utilization
Improving staff proficiency in data analysis is essential for increasing company productivity. At all organizational levels, data literacy is necessary to guarantee that staff members can efficiently analyze and apply data insights in decision-making procedures. Through the provision of requisite competencies, organizations can fully leverage their data assets.
Developing a data-driven culture within the company requires putting measures in place to train staff members on data analysis tools and methods. Offering thorough training courses, workshops, and other materials can enable people to use data to drive better operational performance. Training staff through online courses, mentorship programs, and practical exercises are good approaches to improve their ability to use data analytics tools.
Encouraging staff members to pursue ongoing education and training in data analysis might help them gain a competitive advantage in the current dynamic corporate environment. Promoting an inquisitive and exploratory attitude towards data enables employees to obtain important knowledge and stimulate creativity in their jobs. Putting money into training programs for efficient data use improves individual performance and raises the organization's analytical capacity as a whole.
10. Best Practices in Implementing a Data Culture within Organizations
Establishing a culture that values data-driven decision-making is a necessary step in implementing a data culture within a company. This can be accomplished by emphasizing the importance of data informing strategic decisions and motivating staff members to base choices on data insights as opposed to gut feeling or prior knowledge. It is essential to cultivate a culture that values experimentation and learning from mistakes. Employee experimentation, hypothesis testing, and iteration based on results are encouraged, which promotes innovation and ongoing organizational growth.
Organizations can assist employees develop their data literacy skills by offering training and resources. This will help create a culture where data-driven decision-making is valued. This entails putting on workshops on methods and tools for data analysis as well as advocating for the use of data at all organizational levels to support decisions and verify hypotheses. Employers may encourage staff members to use data in decision-making by showcasing the benefits of data-driven choices through case studies and success stories.✉️
Creating a secure environment where staff members can try out novel concepts and projects without worrying about failing is essential to promoting experimentation. Organizations can change their emphasis from avoiding mistakes to accepting them as a necessary component of the innovation process by recognizing both triumphs and failures as chances for learning and development. Activating teams to carry out small-scale trials, collect data on results, evaluate findings, and modify tactics correspondingly cultivates a proactive problem-solving and ongoing learning culture.
Leadership commitment is necessary to prioritize and encourage the use of data in decision-making processes in order to establish a strong data culture. In today's fast-paced business environment, firms may better exploit data to create efficiency, innovation, and competitive advantage by integrating these best practices into organizational principles and norms.
11. Leveraging Advanced Analytics to Drive Sustainable Business Growth
In today's dynamic corporate environment, using advanced analytics has become essential to promoting long-term growth. Through the application of advanced statistical techniques, companies might find unexplored prospects for expansion within their current data. These techniques go beyond simple analysis to find correlations, trends, and patterns that are missed by more conventional methods.
Another potent technology that has the potential to completely transform strategic planning procedures is predictive modeling. Through the use of previous data and patterns, organizations may estimate future outcomes and make well-informed decisions that optimize their chances of success. Businesses can stay ahead of the curve by taking a proactive approach, recognizing changes in the market, and modifying their plans accordingly.
Businesses can open up new growth and innovation opportunities by utilizing the potential of sophisticated analytics approaches like predictive modeling and advanced statistical methods. In an increasingly competitive world, these state-of-the-art solutions enable firms to make data-driven decisions that propel sustainable corporate growth.
12. Harnessing Big Data Strategies for Long-Term Competitiveness
To maintain long-term competitiveness in the fast-paced business environment of today, big data tactics must be effectively utilized. Effective big data collection, archiving, and analysis enables businesses to gain important insights that can inform strategic decision-making. Businesses can obtain a competitive edge by using these data to better understand market trends, customer preferences, and operational efficiencies.
Gathering data properly entails gathering information from a variety of sources, including social media interactions, commercial transactions, and consumer interactions. Ensuring the secure storage of this data guarantees its availability for analysis while adhering to data privacy laws. In order to identify significant patterns, correlations, and trends that can yield insightful business information, big data analysis requires the application of sophisticated tools and methodologies.
By using big data insights, businesses can replace guesswork and intuition with real-time knowledge to make well-informed decisions. These insights can assist companies in finding new revenue sources, streamlining operations, raising customer happiness, and successfully managing risks. In a market that is changing quickly, companies may maintain an advantage over their competitors by utilizing big data efficiently.
Utilizing big data methods is essential for companies looking to stay competitive over the long term in the fast-paced market of today, as I mentioned above. Businesses can establish themselves as leaders in their field with a thorough grasp of their target markets and a proactive approach to satisfying client requirements by efficiently gathering, storing, and analyzing large amounts of data and making intelligent use of the insights they obtain.