1. Introduction
The enormous volume of organized and unstructured data that businesses and organizations produce on a daily basis is referred to as "big data." Numerous sources, including social media, sensors, devices, and more, provide this data. The insights that big data may yield make it crucial to use it wisely. Businesses that analyze this data can find important patterns, trends, and correlations that can guide their decision-making, enhance their operations, and provide them a competitive advantage in their particular markets.
Even though big data has the ability to completely transform business, there are certain typical blunders that companies should stay away from in order to fully utilize this technology. We'll talk about five important pitfalls to avoid when using big data in this blog post. Companies can make sure they are getting the most out of their big data investments and producing significant outcomes for their operations by avoiding these mistakes.
2. Mistake 1: Neglecting Data Quality
When working with huge data, it is typical to overlook data quality. Any good analysis starts with clean, accurate data. Inaccurate or filthy data can result in poor insights, bad judgments, and resource waste. Making sure that the data being used is of the highest quality requires a significant investment of time and money.
Relying on incomplete or inaccurate data may have negative effects. It may lead to erroneous conclusions, which could affect company plans and results. Decision-makers could make expensive errors as a result of basing their decisions on inaccurate information. Inadequate data quality has the potential to weaken public confidence in analytics platforms and lower the overall success of big data projects. Prioritizing data quality from the beginning is crucial to preventing these negative consequences on the success of your company.😄
3. Mistake 2: Overlooking Data Security and Privacy
Mistake 2: Overlooking Data Security and Privacy
Neglecting data security and privacy when it comes to big data can have catastrophic repercussions. In big data projects, protecting sensitive data is essential to preserving consumer trust and protecting important data assets. Your company could be exposed to cyberattacks and breaches if security procedures are neglected, which could lead to significant financial losses, legal ramifications, and reputational harm.
Beyond merely monetary losses, data breaches can have far-reaching effects. They may damage a brand's reputation, diminish consumer confidence, and even result in legal action or sanctions from the government. For those whose privacy has been violated, the disclosure of sensitive information may have long-term consequences. Organizations must place a high priority on strong data security procedures, encryption standards, access restrictions, monitoring systems, and adherence to pertinent data protection laws like the CCPA and GDPR in order to reduce these risks. Through proactive resolution of data security and privacy issues in big data initiatives, companies can enhance their ability to safeguard themselves and their stakeholders against possible harm.
4. Mistake 3: Failing to Define Clear Objectives
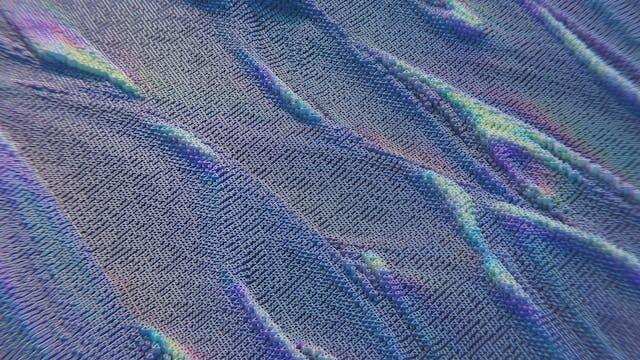
Mistake 3: Failing to Define Clear Objectives
When using big data, one critical error that is frequently made is not defining clear objectives before beginning analysis. Setting clear objectives is crucial because it guides the data analysis process and guarantees that the conclusions drawn are applicable and practical. Without specific goals, firms risk squandering time and money gathering and examining data that is incongruent with their overarching strategic objectives.
Imprecise or unclear goals might result in erroneous perceptions and poor decision-making. Uncertain objectives increase the risk of inaccurate interpretation of the data and findings. As a result, the organization may make bad strategic decisions that have unfavourable effects. You may extract valuable insights that have a direct influence on business performance by laying out your goals for data analysis in a clear and concise manner.
Setting definite goals is essential when handling large amounts of data. Setting clear objectives that complement the organization's strategic direction is crucial to guaranteeing that the knowledge gleaned from data analysis is applicable and worthwhile. By avoiding the error of having imprecise goals, one can save money and avoid drawing the wrong conclusions, which will ultimately result in more effective decision-making based on precise data-driven insights.
5. Mistake 4: Ignoring Scalability and Infrastructure Needs
The fourth mistake made when working with big data is ignoring the infrastructure and scalability requirements. The challenges of scaling infrastructure for effective Big Data operations must be addressed as data quantities rise. Using a distributed computing platform such as Apache Hadoop or Spark is one approach to make sure systems can manage increasing amounts of data. When data volume increases, these frameworks enable horizontal scalability by adding more machines to the cluster, enabling smooth expansion without sacrificing performance.
Cloud services like Google Cloud Platform and Amazon Web Services (AWS) can offer Big Data infrastructure on-demand scalability. Cloud platforms allow businesses to quickly adjust to changing requirements without having to make large upfront hardware investments since they provide resources that can be readily scaled up or down based on data processing needs.
Scalability of the system can be improved by implementing effective data storage options such as NoSQL databases or HDFS (Hadoop Distributed File System). Large-scale data storage and retrieval are handled efficiently by these technologies, guaranteeing that the infrastructure can withstand the growing demands of Big Data operations without experiencing bottlenecks.
Through the proactive use of distributed computing frameworks, cloud services, and efficient data storage solutions, organizations can effectively handle expanding data volumes and establish a strong foundation for their Big Data initiatives. This effectively addresses scalability and infrastructure concerns.
6. Mistake 5: Disregarding Ethical Considerations
Mistake 5: Disregarding Ethical Considerations
Making ethical decisions is crucial when working with big data. Throughout the process, it's critical to keep the ethical ramifications of data gathering, analysis, and application in mind. Disregarding ethical issues can have serious repercussions, including invasions of privacy, prejudice, and even legal trouble.
Data analysis can lead to a number of moral conundrums. Unauthorized data collecting without consent is a prevalent problem that infringes upon people's right to privacy. Using biased algorithms that uphold discrimination against particular groups on the basis of attributes like gender or ethnicity presents another conundrum. Decisions based on inaccurate or insufficient data can have detrimental effects on companies and society at large.
Organizations may increase customer trust, reduce risks, and promote a more fair and equitable use of data for the benefit of all parties involved by putting ethics first in their Big Data operations.
7. Conclusion
To sum up what I wrote above, in order to use Big Data effectively, it's critical to avoid these critical errors: ignoring security precautions, putting too little emphasis on expertise, failing to align insights with business goals, and losing out on cross-team collaboration. Organizations must place a high priority on ethical behavior and strategic planning if they are to fully realize the possibilities of big data.
Strategic planning that is effective makes sure that decisions based on facts are in line with company goals and produce significant results. Businesses may foster trust among stakeholders and mitigate potential risks by including ethical considerations into every level of the data analysis process. Recall that in today's data-driven world, big data is an effective instrument that needs to be used wisely for long-term success.🖇