1. Introduction
The world of big data has significantly shifted from traditional data warehousing in the ever-changing field of data management. Big data solutions, which offer scalability and real-time insights, have replaced data warehousing as the mainstay of corporate intelligence. The enormous amount, speed, and diversity of data produced in the current digital era has played a major role in driving this growth.
For contemporary organizations hoping to maintain their competitiveness in a data-driven environment, making the shift from data warehousing to big data is essential. Big data technologies allow businesses to quickly make well-informed decisions by using the power of large databases and revealing insightful information. Teams that embrace this shift will be better able to analyze large, complicated data sets more quickly, gain deeper understanding, and produce superior business results.
2. Understanding Data Warehousing
An organization can store and manage data from multiple sources in an organized manner by employing data warehousing. It entails the extraction, transformation, and loading (ETL) of data from various operating systems into a data warehouse, which is a centralized repository. In order to facilitate analytical reporting, the data in a warehouse is usually arranged into dimensions and facts.
Three layers make up a traditional data warehousing architecture: the warehouse, which houses integrated and cleaned data, the staging area, which loads and transforms raw data, and the presentation layer, which allows for reporting and querying. Businesses can efficiently execute complicated searches over vast databases thanks to this design.
Improved decision-making through access to aggregated, high-quality data for analysis is one benefit of data warehousing. It enables business intelligence tools, eases regulatory compliance, improves overall corporate performance, and gives historical context for trends detection. Nevertheless, large amounts of unstructured or real-time data are frequently difficult for conventional data warehouses to manage efficiently. Their rigid structures may make them difficult to grow and difficult to adjust to changing business needs.
3. The Rise of Big Data
The emergence of big data represents a fundamental shift in the way businesses handle and use enormous amounts of data. Big data is the term used to describe datasets that are too big or complicated to be processed effectively by conventional methods. The three Vs: volume, velocity, and variety, define it. Volume is a symbol for the enormous amount of data that is produced every day from various sources, including sensors and social media. The speed at which this data is generated and the necessity for real-time analysis is known as velocity. Variety refers to the range of forms and kinds of information that are being gathered.
Big data solutions are becoming more and more popular in modern enterprises for a number of reasons. First, in order to quickly extract meaningful insights, new tools and methodologies are required due to the exponential growth of data. Big data technologies give businesses the power to quickly evaluate enormous datasets and reveal hidden patterns or trends that were previously difficult to identify with traditional techniques. Second, there has been an increase in demand across industries for real-time decision-making. Big data solutions enable businesses to quickly make well-informed decisions based on the most recent information by providing near-instantaneous analysis. Finally, big data gives businesses a competitive edge by empowering them to use data-driven strategies to innovate, boost consumer experiences, increase operational effectiveness, and spur growth.
4. Challenges in Transitioning to Big Data
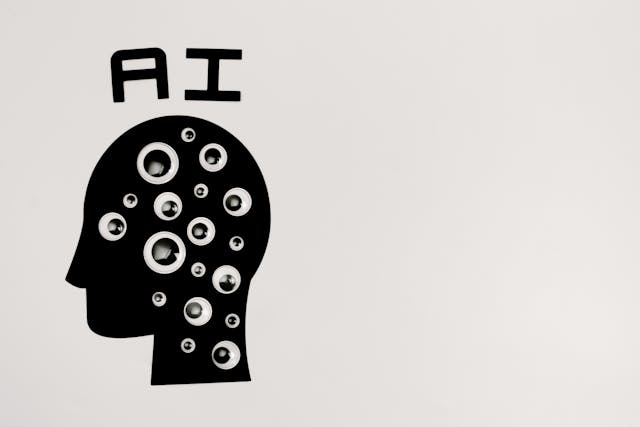
For enterprises, moving from traditional data warehousing to big data can present a number of difficulties. The inability of the current infrastructure to scale to handle massive volumes of data in real-time is one typical barrier. Conventional systems frequently lack the capacity to manage the amount, speed, and diversity of data that big data technologies require.
The team's disparity in skill sets presents another difficulty. When working with large data, a different set of abilities is needed than when working with typical data warehousing. It might be necessary to hire new personnel with experience in data science, distributed computing, and programming languages like Python or R, or to train current team members.
Organizations can employ experts in big data technology or engage in training programs to upskill their current team members in order to effectively address these difficulties. Establishing a culture of ongoing learning and adaptation is crucial to ensuring that the team is prepared to fully utilize big data's potential.
Rather than enacting drastic changes, organizations should think about progressively migrating their current systems and procedures. Teams may learn and adjust at a sustainable pace with this staged approach, which minimizes interruptions to existing operations.
During the transition period, business stakeholders and IT teams must work together closely. All parties can better coordinate their efforts and make sure they are working toward the same goal by having clear communication about expectations, timetables, and goals. Frequent checkpoints and feedback sessions can also offer insightful information about the areas that have progressed and still need work. 🔆
Organizations may effectively traverse the shift from data warehousing to big data by proactively tackling these issues with well-informed strategies, opening up new avenues for insights and innovation in the process.
5. Planning for Transition
Making the shift from data warehousing to big data involves considerable thought and strategy, and planning is a crucial step in the process. The first step in creating a thorough transition strategy is to clearly define the migration's aims and objectives. Determine which internal stakeholders, such as IT directors, data engineers, and business analysts, will be essential to this shift.
Early involvement of important stakeholders is essential to the transition's success. Talk to them to find out about their needs, worries, and expectations for the move to big data. It is possible to make sure that the transition plan is in line with the organization's overall strategic direction by getting input and support from these stakeholders.
Set aside the funds required to train and advance your team's knowledge of big data technologies. This could entail spending money on workshops, training courses, or engaging outside experts with big data experience. Examine your present systems and infrastructure to find any weaknesses or areas that require modernization to effectively handle big data processing.
Work together with your IT staff to create a plan for integrating the new tools and technologies needed to handle large amounts of data. Set up deadlines, checkpoints, and deliverables to monitor the transition process's progress. You can make sure that the transition from traditional data warehousing to big data processing goes smoothly and successfully by establishing clear goals and incorporating important stakeholders early on.
6. Evaluating Big Data Technologies
Succeeding in transferring your team from traditional data warehousing to big data requires careful consideration of the appropriate technologies. Well-known big data platforms like Spark, Hadoop, and others have special features that can revolutionize the way your team handles and examines data.
The capacity of Hadoop to handle and store enormous volumes of data over a dispersed computer network is well known. It is perfect for effectively managing jobs involving large-scale batch processing. In contrast to Hadoop's disk-based processing, Spark offers in-memory processing capabilities, enabling quicker data processing speeds.
It's crucial to take into account aspects like scalability, performance requirements, cost-effectiveness, ease of use, and compatibility with current systems when choosing the right technology for your company's needs. Assess if the technology can effectively support future expansion and is in line with the aims of your firm. 😽
Evaluate your team's skill sets to find out what technologies they can quickly become accustomed to. During the decision process, it is important to take into account training resources and vendor or community support to guarantee a seamless transition and continued success in utilizing big data technology.
You can prepare your team for a smooth transition to utilizing big data for enhanced decision-making and insights by carefully weighing these factors and choosing the best big data technology that fits your goals as a business.
7. Training and Upskilling Team Members
It's critical to train and upskill team members when switching from big data technology to traditional data warehousing. It gives them the ability to adjust to the new instruments and procedures, guaranteeing a seamless shift. By investing in training, you not only ready your workforce for the future but also raise their morale and engagement.
In order to effectively upskill staff members, take into account customized training plans that accommodate varying skill levels within the group. Team members can learn concepts more quickly and apply them in real-world situations if they have access to online courses, mentorship opportunities, and hands-on workshops. Fostering a culture of growth and creativity within the team is achieved through regular knowledge sharing sessions and certification programs that encourage continual learning.
8. Implementation and Migration Process
There are several crucial processes involved in the implementation and migration from data warehousing to big data platforms. To begin with, thoroughly evaluate your existing data infrastructure to determine data sources, storage options, and integration points. Next, specify the migration project's goals and parameters as well as the key performance indicators (KPIs) that will be used to gauge its success.
Create a detailed plan detailing the migration strategy, including deadlines, resource allocation, and possible obstacles, following the initial assessment. Key stakeholders from the business and IT departments must be included in order to guarantee that the process is aligned with organizational objectives at every stage.
An important part of moving to big data systems is data mapping and transformation. Make that the new platform's data structures and formats work with the ones that already exist. To maximize data quality for analysis in the big data world, data normalization and cleansing could be required.
Before final implementation, testing is an essential step. To find and fix problems early, thoroughly test system integrations, ETL (Extract, Transform, Load) workflows, and data migration procedures. Parallel testing environments should be used to reduce risks during the transition period.
To guarantee a smooth implementation process when transitioning your team from data warehousing to big data, adhere to best practices such as:
1. Communication: Keep lines of communication open with every member of the team working on the migration. Frequently report on accomplishments, swiftly resolve issues, and foster a cooperative work atmosphere.
2. Training: Provide workshops or training sessions on new big data system-related tools, technologies, and procedures. Ascertain that team members possess the necessary abilities needed for an adoption to be effective.
3. Change Management: Put change management techniques into practice to assist staff members in adjusting to their new tasks and responsibilities as a result of the shift. To overcome reluctance, emphasize the advantages and offer assistance as required.
4. Data Security: During the migration process, give top priority to data security measures by putting encryption methods, access limits, and frequent audits into place. Preserving confidential data is essential for upholding customer confidence and adhering to legal requirements.
5. Scalability Planning: Plan for future scalability requirements by designing flexible architectures that can accommodate expanding datasets and user demands over time.🥰
Your team can maximize the value from your organization's data assets while navigating the challenges of moving from traditional data warehousing to robust big data systems by adhering to these best practices and creating a clear implementation plan that includes the specific steps mentioned above.
9. Managing Change within the Team
For a shift from data warehousing to big data to be successful, the team must effectively manage change. One of the major challenges during such moves is addressing resistance to change among team members. To lessen this, it's critical to properly explain the rationale for the change while emphasizing the advantages and opportunities presented by big data. Resistance will also be lessened if team members receive the assistance and training they need to learn new abilities.
Establishing an environment where new technologies are welcomed requires the use of open communication techniques. Providing constructive criticism, opening up communication lines, and including team members in decision-making procedures can help team members feel appreciated and involved during the change. Clear communication regarding expectations, schedules, and modifications fosters trust and lowers doubts, which frequently result in resistance.👗
Team members can express their worries and ask questions by participating in regular updates through meetings, workshops, or even casual get-togethers. Acknowledging and appreciating minor victories along the road raises spirits and maintains drive. Organizations can successfully move from data warehousing to big data by emphasizing excellent communication and involve the team in the process.
10. Monitoring Performance and Success Metrics
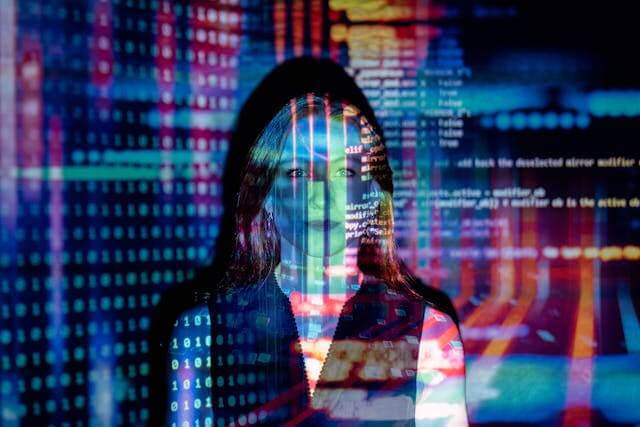
Keeping an eye on success and performance metrics is essential when moving your organization from data warehousing to big data. Setting up key performance indicators (KPIs) in line with your new big data goals and objectives is crucial to the success of this transformation. These KPIs ought to be SMART—specific, quantifiable, achievable, relevant, and time-bound—and offer precise standards by which to measure advancement. 💡
It's equally crucial to select the appropriate instruments and procedures for tracking and assessing these performance indicators. Advanced analytics tools like Spark, Elasticsearch, and Apache Hadoop can be used to collect and analyze data in real-time and provide insightful information about the performance of your team after the transfer. Presenting complex data sets in an understandable and useful fashion can be facilitated by implementing data visualization technologies such as Tableau or Power BI.📚
You may maximize your team's performance in the big data environment by routinely reviewing these KPIs and indicators, identifying areas for improvement, and tracking trends over time. Your organization's successful shift from data warehousing to big data will be built on a foundation of ongoing monitoring and prompt adjustments based on the insights gathered.
11. Ensuring Data Security and Compliance
When moving your team from data warehousing to big data, it is critical to ensure data security and compliance. Big data settings contain enormous amounts of sensitive data, therefore upholding strong security standards is essential to warding off possible threats and breaches. Protecting data integrity and confidentiality can be aided by the implementation of encryption, access controls, and monitoring systems.
Big data management becomes much more complicated when compliance issues like the California Consumer Privacy Act and the General Data Protection Regulation are taken into account. These laws mandate that businesses manage personal data sensibly, respecting individuals' rights, consent, and openness. There are harsh fines and reputational harm associated with breaking these rules. Therefore, for long-term profitability and stakeholder trust, incorporating compliance regulations into your big data strategy is crucial.
12. Case Studies or Success Stories
For any firm, making the switch from traditional data warehousing to big data can be a difficult adjustment. Examining real-world case studies and success stories might give you important information about how other people have transitioned effectively. One such instance is Company X, a sizable retailer that made the decision to update its data infrastructure in order to manage the growing amount and diversity of data that is produced on a daily basis. Through the implementation of advanced analytics, Company X was able to obtain deeper insights from their data and increase processing speed by switching from a structured data warehouse to a scalable big data solution.
Another interesting case study is Company Y, a financial services organization that started using big data to improve its risk management skills. Through this shift, they were able to leverage real-time analytics on massive volumes of unstructured data streams to not only optimize their current processes but also open up new business opportunities. The most important takeaway from Company Y's experience was how crucial it was to match the organization's aims with the potential provided by big data technology, such that each action made helped to accomplish strategic goals.
These success stories demonstrate how switching from traditional data warehousing to big data solutions may have a dramatic effect. To fully realize the promise of big data, they emphasize the significance of not only modernizing technology but also reinventing company culture and reorganizing processes. Organizations can obtain insightful knowledge from these stories and their experiences, which will help them navigate a smooth transition and maintain their competitiveness in the data-driven world of today.