1. Introduction
Big data is essential for predicting when the wind and sun will blow in the world of renewable energy. In the context of renewable energy, big data refers to enormous volumes of information gathered from diverse sources, including sensors, weather satellites, and historical patterns. It is critical to forecast wind and solar energy availability to guarantee effective energy production and delivery. Renewable energy suppliers can optimize energy output by anticipating changes in cloud cover or wind speeds through the use of big data analytics. Because of its ability to forecast future trends, renewable energy sources are becoming more dependable and economical substitutes for conventional fossil fuels.
2. Big Data in Weather Forecasting
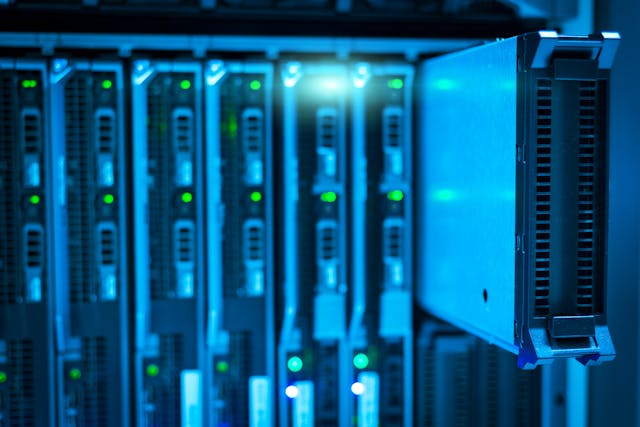
Big data is essential to the field of weather forecasting since it helps predict renewable energy sources like solar and wind power. A variety of platforms, including satellites, weather stations, sensors, and radars, are used to gather meteorological data. Subsequently, this data is processed through the use of advanced algorithms capable of real-time data analysis on massive volumes. When predicting when the wind and sun will blow and shine, these algorithms aid in the identification of patterns, trends, and correlations.
Because advanced algorithms can swiftly and efficiently sort through large datasets, they are crucial to weather forecasting for renewable energy. Machine learning methods, like decision trees and neural networks, are able to identify minute correlations between various meteorological variables. These algorithms combine real-time data with past weather patterns analysis to produce more accurate forecasts of upcoming weather phenomena.🎑
When paired with sophisticated algorithms, the predictive ability of big data offers useful information for planning the production of renewable energy. Renewable energy providers can lower costs, increase energy efficiency, and streamline operations by utilizing this technology. Big data analytics combined with weather forecasting improves renewable energy sources like solar and wind power's overall sustainability and dependability.
3. Predicting Wind Patterns
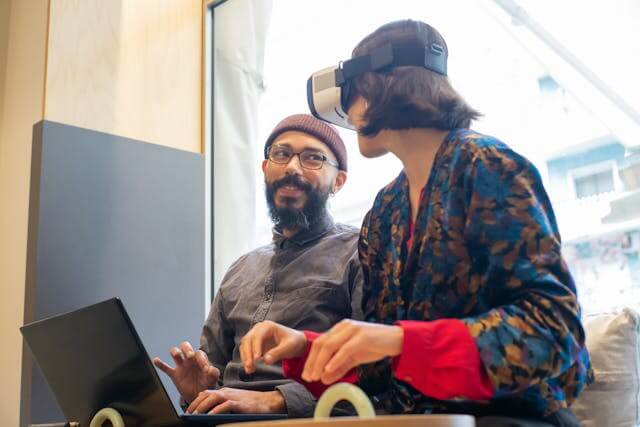
Accurately predicting wind patterns is essential to maximize energy output from wind farms. Data analysts can create predictive models to foresee future wind conditions by utilizing historical wind data. Forecasts of wind direction and speed are heavily influenced by terrain, proximity to bodies of water, and local weather patterns. Large volumes of data are processed by sophisticated algorithms, which produce precise forecasts that allow energy companies to plan maintenance, modify energy distribution, and increase output efficiency. The renewable energy industry has undergone a revolution with the integration of big data analytics in wind pattern forecasting, resulting in increased cost-effectiveness and reliability.💡
4. Forecasting Solar Radiation
To maximize the generation of solar energy, solar radiation forecasting is essential. Big data analytics play a vital role in predicting sunshine intensity accurately. Algorithms assess past weather patterns, satellite imagery, and atmospheric conditions to predict how much sunlight a specific place will receive. Predictions of solar energy are more accurate when geographic parameters like latitude, longitude, elevation, and climate data are taken into account.
When and where the sun will shine the brightest can be predicted using machine learning algorithms that have been trained on enormous amounts of weather and solar radiation data. To produce more accurate forecasts, these models consider a number of factors, including cloud cover, air pollution levels, and time of day. Solar power plant operators can more effectively plan energy generation and storage based on expected sunlight availability by utilizing big data insights.
By combining real-time sensor data with previous weather information, predictive analytics can adapt solar energy production in response to rapid changes in sunshine intensity. This adaptability minimizes energy loss and enables more effective resource use. Using big data for solar radiation forecasting is crucial in the dynamic world of renewable energy to maximize clean energy production while lowering costs and environmental effect.
5. Machine Learning Applications
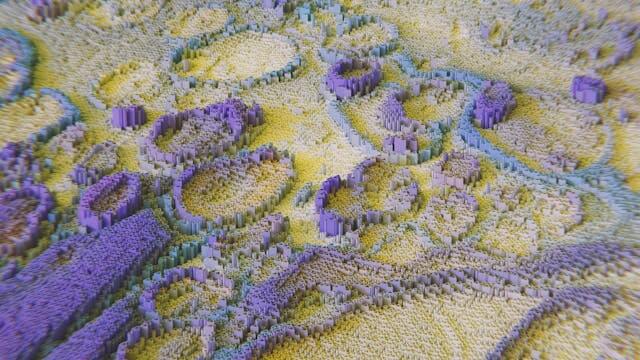
When it comes to renewable energy, the application of big data and machine learning techniques has completely changed our ability to forecast the sun's and wind's movements. Through the use of sophisticated techniques like neural networks and regression analysis, academics have produced forecasts that are far more accurate and helpful to the industry. With the use of these methods, analysts can now easily sort through enormous volumes of complex data to find patterns and insights that were previously hard to find.
Predictive maintenance for wind turbines is one prominent use in this sector. Operators can save money and downtime by reducing the likelihood of failures by putting machine learning algorithms into practice. Because machine learning algorithms can reliably predict sunlight levels, they have played a key role in enhancing the efficiency of solar panels. This maximizes energy output by allowing solar farms to modify their operations in real-time based on anticipated weather conditions.
Case studies provide more evidence of machine learning's efficacy in the renewable energy sector. One research by a major energy business, for example, showed how neural networks might enhance wind power estimates by as much as 30%, leading to better resource planning and more revenue collection. Another example demonstrated how solar plant operators might make well-informed judgments about energy output by using regression analysis to estimate solar irradiance levels with remarkably high precision.
Furthermore, as I mentioned previously, the combination of machine learning and big data analytics has made it possible to make forecasts about renewable energy sources like solar and wind power that are more accurate. We may anticipate even more cutting-edge applications that will propel sustainability and efficiency in the renewable energy industry as long as research and technology continue to progress.
6. Challenges and Solutions
Weather patterns are unpredictable, and solar and wind energy generation is erratic, making it difficult to forecast renewable energy sources with any degree of accuracy. It is difficult to predict when and how much energy will be produced because of these considerations. The intricate process of merging data from multiple sources may result in imprecise forecasts.
Enhancing the prediction data is one way to make it better. More precise forecasts can be produced by gathering high-quality, real-time data from a variety of sources, including satellites, IoT devices, and weather stations. Cleaning up noisy data, fixing discrepancies, and making sure the datasets are complete and current are all part of this data refining process.
Optimizing prediction models with cutting-edge machine learning algorithms is another important remedy. Through the utilization of methods such as deep learning, ensemble learning, and artificial neural networks, scientists may create increasingly complicated models that more accurately reflect the intricacies involved in the production of renewable energy. Energy grid operators may more effectively control supply and demand changes with the use of these optimized models, which can also increase prediction accuracy.
7. Economic Impacts
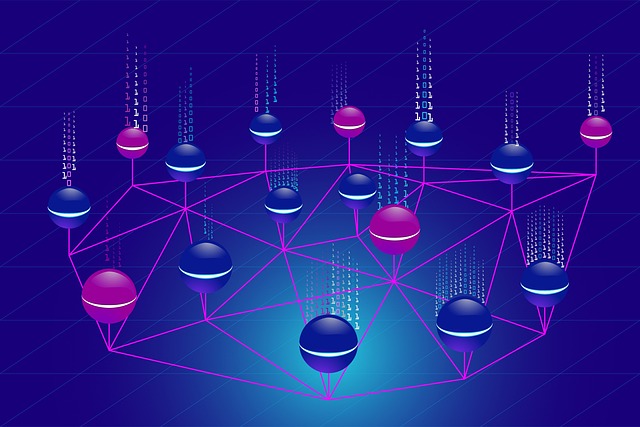
Understanding the effects of weather on energy generation requires analyzing the financial advantages of accurate renewable energy predictions made with big data technologies. Predictive models are able to predict with surprising accuracy when the wind and sun will blow thanks to sophisticated algorithms and vast volumes of data. These discoveries not only increase the production efficiency of renewable energy but also have important ramifications for sector investment choices.
When deciding where and when to invest in projects, investors can make better decisions when they can forecast the availability of renewable energy. Stakeholders can manage their investments to maximize returns while limiting risks associated with weather-related changes when energy generation is more certain. Because of this fine-grained forecasting, decision-makers can spend resources more wisely, which in turn spurs innovation and growth in the renewable energy market.
Accurate forecasts from big data analytics are essential for developing grid management plans. Energy grid managers can modify supply and demand dynamics by using real-time insights, giving customers a steady and dependable supply of electricity. Grid operators can improve overall system resilience, cut waste, and optimize grid operations by incorporating these forecasts into their decision-making processes.
The renewable energy business will be significantly impacted economically by using big data to forecast the wind and solar radiation. Precise forecasting helps stakeholders take advantage of opportunities in this quickly changing industry while promoting sustainable growth for a more environmentally friendly future. It does this by directing investment decisions and establishing grid management strategies.
8. Environmental Sustainability
The way we forecast renewable energy sources like solar and wind power has completely changed as a result of big data analytics breakthroughs. With the use of data, we can now predict with astonishing precision when the sun will rise and the wind will blow. This lessens our dependency on fossil fuels and increases the efficiency of the creation of clean energy.
The advancement of sustainable development goals is significantly aided by increased forecasting accuracy for renewable energy. We can more effectively incorporate wind and solar energy into the grid, improving its use and cutting waste, by accurately forecasting their availability. Reduced carbon emissions and a more sustainable energy ecology result from this enhanced efficiency.
Fighting climate change and advancing environmental sustainability require us to use clean energy sources more efficiently in order to lessen our reliance on fossil fuels. Big data has made it possible for us to use renewable resources more wisely, providing a route forward for a more environmentally friendly future. We are moving closer to a future with clean, renewable energy that is dependable and eco-friendly by adopting these technological advancements.
9. Future Trends in Renewable Energy Prediction
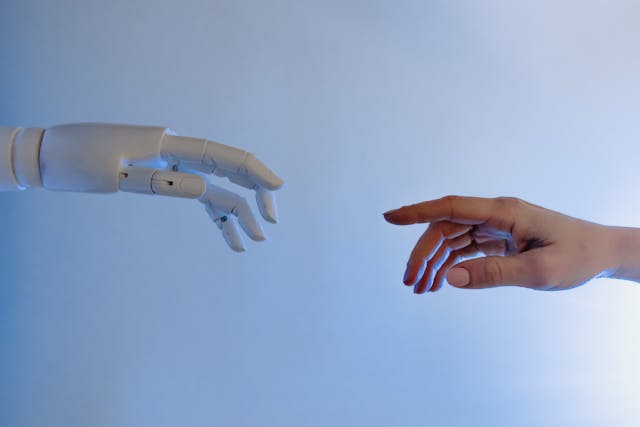
New technologies are changing the game when it comes to predicting future trends in renewable energy. Accurate forecasting is being revolutionized by innovations like blockchain integration, satellite imaging, and Internet of Things sensors. In order to maximize the production of renewable energy, these developments open the door to more accurate forecasts of the wind and sun.
With the increasing need for renewable energy sources worldwide, forecasting accuracy is critical. The efficient fulfillment of this increasing demand is possible with the use of emerging technology. Predictive models can make more accurate predictions about the patterns of renewable energy generation by utilizing data analytics and real-time monitoring via Internet of Things sensors and satellite photography.
Forecasting systems that include blockchain technology benefit from increased data management security and transparency. In addition to guaranteeing the accuracy of prediction models, this integration makes it easier for different players in the renewable energy industry to work together smoothly. These advancements point to a bright future where reliable and effective projections of renewable energy promote environmentally friendly energy practices worldwide.
10. Case Studies: Successful Implementations
A number of noteworthy case studies demonstrate how big data has been successfully applied to the prediction of solar and wind patterns. A noteworthy instance of this is the partnership between The Weather Company and IBM, wherein The Weather Company's extensive data sources and IBM's cutting-edge analytics technologies were combined to maximize forecasts for renewable energy. By using a synergistic strategy, wind farms were able to increase operational efficiency by dramatically improving the accuracy of wind speed predictions.
The collaboration between National Renewable Energy Laboratory (NREL) and GE Energy Consulting is another noteworthy example. This partnership produced a breakthrough in solar power forecasting by utilizing NREL's experience in renewable energy research and GE's Predix platform for data analytics. By combining historical meteorological data, satellite imaging, and real-time sensor data, solar plants are now able to estimate solar irradiance levels with more accuracy, leading to increased generating capacity and energy output.
Vaisala's success story is noteworthy because they improved their wind forecasting models by incorporating state-of-the-art machine learning methods. By combining historical weather data with current meteorological information, Vaisala was able to significantly improve forecast accuracy for evaluations of wind resources. This development allowed for the more effective integration of variable wind power into the current electrical networks, which enhanced grid stability in addition to optimizing energy production planning.🗒
These case studies demonstrate the real-world advantages of using big data analytics for forecasting renewable energy. Businesses can minimize risks, cut expenses associated with operations, and seize fresh growth prospects in the quickly changing field of sustainable energy generation by utilizing predictive analytics.
11. Policy Implications
Policymakers have a revolutionary chance to completely change how they approach the production of sustainable energy by incorporating big data projections into national energy strategy. Governments may improve the efficiency and dependability of renewable energy sources like solar and wind power by utilizing these cutting-edge technology. In order to facilitate the adoption of these prediction technologies and lessen dependency on fossil fuels in the future, policymakers have the opportunity to establish incentives.
The requirement for regulatory frameworks that facilitate the incorporation of big data analytics into energy planning and operations is a significant policy impact. To encourage openness and cooperation among players in the energy industry, policymakers might endeavor to set standards for data gathering, exchange, and analysis. To encourage investment in predictive analytics technologies and hasten industry adoption, governments can offer grants, tax credits, or other financial incentives.
To accelerate the development and implementation of big data solutions for renewable energy forecasting, policymakers should consider forming alliances with academic institutions and businesses in the private sector. Governments may utilize the expertise of multiple professions to propel innovation in clean energy technology by promoting collaboration among various sectors. This cooperative strategy promotes economic growth and job creation in developing industries in addition to hastening the shift to a more sustainable energy system.
Encouraging policymakers to incorporate big data predictions into national energy strategy is a chance to tackle urgent environmental issues like climate change. Governments can lessen the effects of global warming by encouraging the use of predictive analytics technologies in the production of renewable energy. Policymakers may guide their nations toward a low-carbon future while guaranteeing energy security and affordability for consumers by enacting targeted policies that promote investment in clean technology solutions.
To sum up what I've written so far, national energy strategies that incorporate big data projections have enormous potential to transform the way we produce and use electricity in a way that is more sustainable. When it comes to fostering innovation and investment in predictive analytics solutions for renewable energy applications, policymakers are essential. Taking advantage of this chance, governments may steer their countries toward a more resilient and cleaner energy future while promoting economic growth and environmental stewardship by instituting policies that encourage the use of big data insights.
12. Conclusion
The application of big data to forecast solar and wind patterns for the production of renewable energy is extremely important. Through the utilization of copious amounts of data from many sources, including weather stations, satellites, and sensors, we are able to predict with remarkable accuracy when the sun will shine and the wind will blow. This capacity for prediction is essential for maximizing the production of renewable energy, enhancing grid stability, and lowering dependency on fossil fuels.
With the use of big data analytics, we can more accurately predict variations in the generation of renewable energy. This gives energy operators the ability to decide on resource allocation, energy distribution, and grid management with knowledge. We clear the path for a more sustainable future marked by a greater penetration of renewable energy sources and a less environmental effect by utilizing sophisticated forecasting tools powered by big data.
It is impossible to exaggerate the importance of big data in improving our comprehension of solar and wind patterns as we move toward a world fueled primarily by renewable energy sources. With ongoing developments in AI-powered analytics systems, machine learning algorithms, and predictive modeling, we have the potential to completely transform the dependability and efficiency of renewable energy production on a worldwide basis. Adopting these technologies gives us the chance, as well as the obligation, to create a more sustainable future for future generations.