1. Introduction:
Artificial intelligence (AI) has completely changed the way firms function in the retail industry. AI technology have improved consumer experiences and streamlined operations in a variety of areas, including inventory management and tailored suggestions. Data annotation, or the process of identifying and classifying data so that machine learning algorithms can understand it, is essential to the efficacy of AI in retail. Massive volumes of data may be accurately analyzed and learned from by AI systems thanks to data annotation. Robust data annotation is necessary in the retail setting to train AI models that comprehend customer behavior, anticipate trends, improve pricing tactics, and efficiently manage supply chain operations.
2. Enhanced Customer Experience:
Enhanced Customer Experience: Data annotation plays a crucial role in elevating the customer experience within the retail sector, particularly through refining product recommendations. By accurately labeling and categorizing data related to shopper preferences, behaviors, and purchase history, AI algorithms can offer more personalized and relevant product suggestions. This leads to increased customer satisfaction and engagement as individuals are presented with items that align closely with their interests and needs.
Retailers can gain a more comprehensive and detailed picture of their customers by utilizing annotated data. Businesses can obtain important insights into customer behavior patterns by annotating customer demographics, past encounters, and feedback thoroughly. More personalization tactics are made possible by this abundance of data, which enables merchants to customize their products and advertising to appeal to particular client demographics or even personal tastes. Retailers can build enduring brand loyalty and closer relationships with their target market by doing this.
3. Operational Efficiency:
Annotating data is essential for improving operational effectiveness in the retail industry. Retailers can simplify inventory management procedures by using annotated data. Precise product labeling and classification facilitates enhanced stock tracking and management, resulting in better inventory control and fewer overstock or stockout situations.
Annotated datasets offer important information that aids in precisely estimating demand, which is helpful in supply chain logistics optimization. Retailers can make well-informed judgments that optimize the flow of the supply chain by annotating data pertaining to product categories, locations, and consumer preferences. Because of this accuracy, delays are reduced, routing is optimized, and eventually, cost savings are achieved through effective logistics operations.
In summary, data annotation plays a critical role in transforming retail operations by promoting operational efficiency through improved supply chain logistics and efficient inventory management. In today's cutthroat retail environment, merchants may make well-informed decisions that improve their entire business performance and their bottom line thanks to the precise and comprehensive information annotated datasets provide.
4. Fraud Detection and Security:
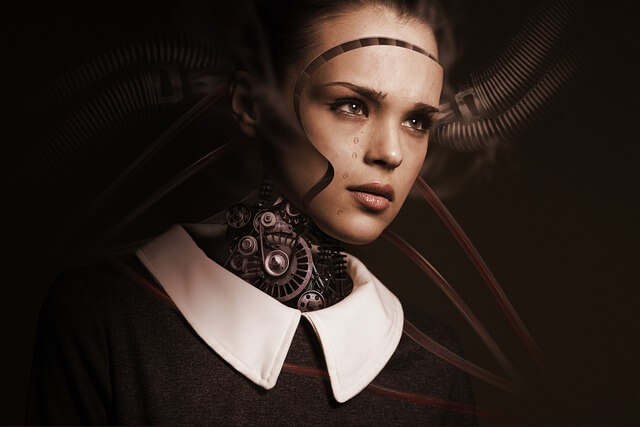
To protect transactions and improve overall security in the retail industry, data annotation plays a critical role in fraud detection and security measures. Retailers may create powerful fraud detection systems that examine trends, irregularities, and disparities in real-time transactions by utilizing annotated data. These comments improve the accuracy with which AI systems detect fraudulent actions like account takeovers, payment fraud, and illegal access.
Robust annotations are essential for reinforcing security protocols in retail settings. Security protocols can be strengthened by AI-powered systems that are trained on carefully labeled datasets and are capable of identifying possible threats, weaknesses, and suspicious activity. Retailers may put preventive security measures in place with this proactive strategy, safeguarding sensitive customer data, preventing data breaches, and guaranteeing customers a safe and secure shopping experience.
Through the use of annotated data for security and fraud detection, businesses can reduce financial risk and foster customer trust by implementing dependable and secure transaction procedures. The foundation for a robust cybersecurity architecture that can react to changing threats and protects the integrity of retail operations in an increasingly digital environment is laid by the integration of AI technologies powered by precisely labeled datasets.
5. Better Decision-making:
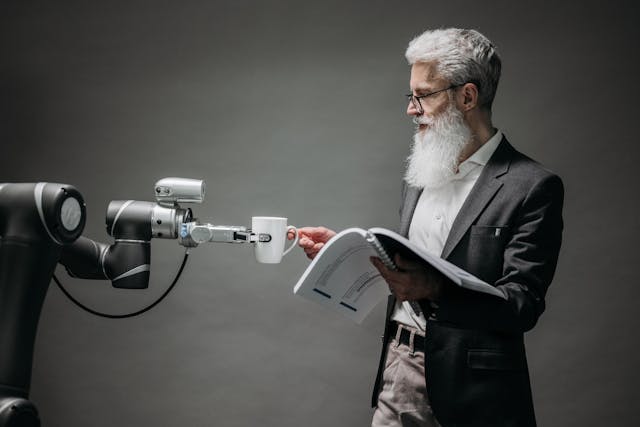
Annotating data is essential for improving decision-making in the retail industry. Retailers may more accurately forecast trends and consumer behavior by utilizing annotated data. Businesses are able to anticipate changes in the market and adjust their strategy to match changing customer needs because to this predictive capabilities. Precise data annotations play a major role in enhancing strategic decision-making by providing merchants with trustworthy insights from annotated data sets upon which to base their decisions. As a result, decisions become more impactful and accurate, which helps propel growth and success in the cutthroat retail sector.
6. Product Development and Innovation:
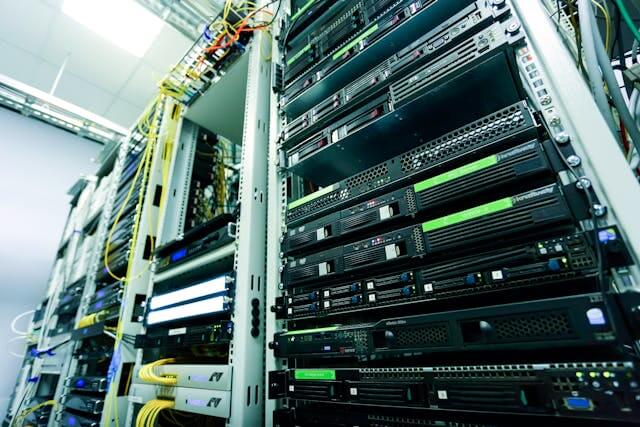
In the retail industry, data annotation is essential to innovation and product development. Retailers can learn important information about the preferences and trends of their customers by using annotated data in their market research. Thorough annotations offer a more profound comprehension of customer inclinations, enabling companies to efficiently customize their offerings to fulfill certain requirements. This procedure improves overall client happiness while streamlining the development of new products.
Because data annotation enables businesses to make data-driven decisions based on accurate and trustworthy information, it fosters innovation in the retail industry. Retailers can recognize new trends and modify their tactics by using detailed annotations to understand consumer behavior. By using the power of annotated data, organizations can develop more tailored experiences for their customers, and this proactive approach stimulates innovation within the sector.
Fundamentally, data annotation propels product innovation and development in the retail industry, enabling businesses to stay ahead of the curve by quickly adapting to shifting consumer preferences and market realities. In an increasingly competitive world, retailers can seize new chances for development and distinction by implementing comprehensive annotations into their strategic planning processes.
7. Cost Reduction and Profit Maximization:
Because it simplifies processes and boosts productivity, annotated data is essential for cutting costs in the retail industry. Retailers may automate a number of tasks, including demand forecasting, inventory management, and customized marketing campaigns, with the help of precisely labeled information. In addition to lowering the expense of human work, this automation also lowers errors, resulting in more accurate decision-making.
Retailers may increase revenues through focused strategies by utilizing AI-driven insights from well-annotated information. Artificial intelligence (AI) systems can more accurately detect patterns, consumer preferences, and outliers by examining annotated data. Retailers are able to efficiently match client expectations by customizing their offers, pricing tactics, and promotions, which in turn boosts sales and profitability.
In summary, data annotation essentially serves as the cornerstone that propels operational efficiency and opens up revenue streams for the retail sector by facilitating precise forecasts and well-informed decision-making grounded in dependable datasets.
8. Challenges Faced in Data Annotation for Retail AI:
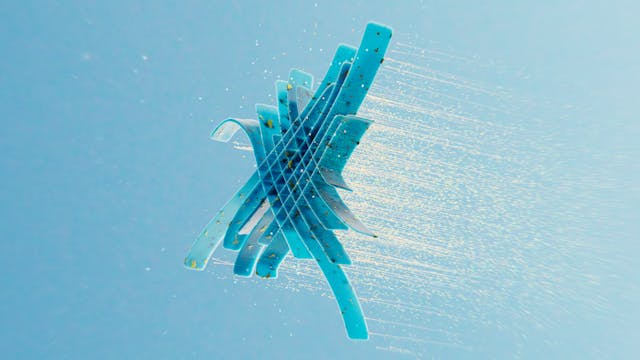
Data annotation presents a number of issues in the field of retail AI that must be successfully resolved. Scalability is the first issue because retail datasets can be large and heavily resource-intensive to accurately annotate. Another challenge is maintaining quality assurance, which makes sure that the annotations are accurate and uniform across the dataset. Diversity in annotated datasets is essential for taking different retail settings and demographics into consideration.
It is imperative to put strong tactics into place in order to overcome these obstacles. Using automated tools for annotation can speed up the process and cut down on human labor while preserving accuracy. Regular audits and feedback loops are two examples of quality assurance techniques that can guarantee the accuracy of annotations. Diverse viewpoints can be added to datasets through crowdsourcing or outsourcing the annotation process, which can help add the diversified insights required for successful AI adaption in retail environments.
Retailers may build well-annotated datasets that serve as the basis for successful AI integration into their operations by solving challenges with scalability, quality assurance, and diversity through strategic methods.
9. Tools and Technologies for Data Annotation:
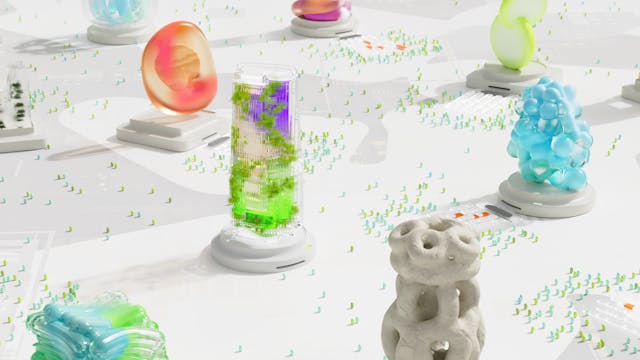
Data annotation is a key component of machine learning model training in the retail AI domain. To precisely and effectively annotate retail datasets, a variety of methods are used. Scale AI, Labelbox, and Amazon SageMaker Ground Truth are a few of the well-liked products. These platforms provide functionality related to retail data types, including as object detection, text categorization, and image tagging.
In the context of retail AI adaption, there are advantages and disadvantages to both automatic and manual annotation methodologies. Because human annotators are able to comprehend the context, linkages, and subtleties included in the data, manual annotation ensures excellent accuracy. However, for large datasets, it is expensive and time-consuming. Conversely, automated annotation methods make use of algorithms to greatly accelerate the process, but they might not be as accurate for complicated retail data, which frequently contains minute details and variances.
In order to train AI models for their particular needs, retailers must find a balance between automated and manual data annotation techniques. In the highly competitive retail environment, merchants may successfully harness the potential of annotated data to drive innovation and improve consumer experiences by carefully combining these approaches based on dataset complexity and budgetary restrictions.
10. Regulatory Compliance and Ethical Considerations:
An important part of the process is data annotation, as the retail business uses AI for more and more jobs. Data annotation becomes even more important when it comes to ethical and regulatory compliance. Adherence to regulations such as GDPR is necessary when annotating sensitive retail data to guarantee the preservation of consumer privacy and data protection consistently. The ethical aspects of privacy and bias need to be properly taken into account when annotating retail datasets for AI models.
Retailers can reduce the risks of handling sensitive data improperly by putting in place appropriate data annotation procedures that adhere to laws like GDPR. Gaining the trust of stakeholders and customers requires handling annotated data in an ethical and transparent manner. It is imperative to tackle prejudice in data annotation in order to create equitable AI models that do not reinforce biased results in the retail industry.
After putting the aforementioned into summary, we can say that retailers can use AI to their advantage while maintaining customer confidence and advancing justice in their business practices if they give regulatory compliance and ethical issues first priority during the data annotation process. To effectively use AI to improve consumer experiences and streamline business processes, merchants must approach data annotation with sensitivity to privacy, transparency, and justice.
11. Case Studies:
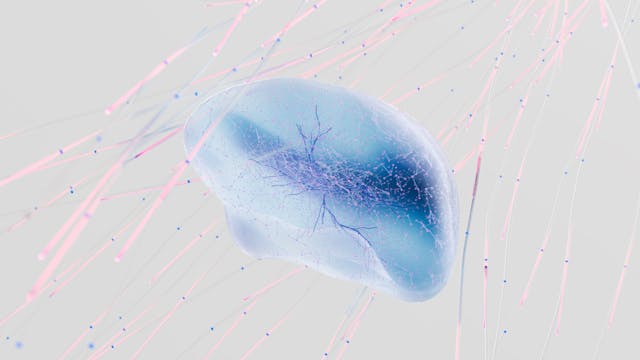
In order to show how annotated data can effectively advance AI capabilities in the retail sector, case studies are essential. These real-world examples demonstrate how accurate data annotation transforms retail processes and produces effective outcomes. A well-known online fashion company used annotated consumer data to improve their recommendation algorithm in one such case study. They greatly increased the accuracy of their algorithm by appropriately tagging photos and products with pertinent tags, which raised consumer happiness and sales.
An impressive example of a case study that makes use of annotated data for inventory management is provided by a grocery chain. They reduced waste, expedited ordering procedures, and improved stock levels by carefully labeling product photos and descriptions. This approach not only reduced expenses but also made sure that shelves were always filled with in-demand merchandise, enhancing the general shopping experience.
Annotated data was used by a well-known electronics store to improve pricing tactics. Their analytic of competition pricing data and consumer reviews yielded insightful knowledge about how to dynamically modify prices. This strategy raised profit margins, boosted sales volume, and enhanced market competitiveness.
These case studies highlight the critical function that data annotation plays in enabling AI-powered retail solutions. Retailers can seize new chances for innovation, efficiency, and growth by utilizing appropriately labeled data.
12. Future Outlook:
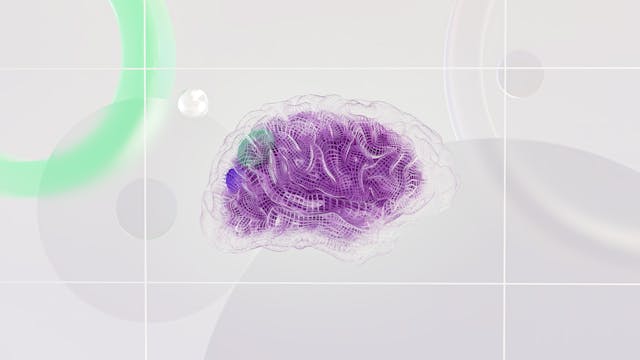
Data annotation will remain essential in influencing AI advancements in the retail industry in the future. The need for high-quality annotated data will only grow as technology develops. As a result, more advanced methods and tools for data annotation will be developed to guarantee precision and effectiveness while training AI models for use in retail settings.
A move toward more automated and scalable data annotation systems is something we should anticipate. Large datasets will be annotated more rapidly and precisely with the help of machine learning techniques, which will minimize the need for personal interaction. The process of annotating data will be sped up by this automation, which will also raise the standard of annotated data overall.
It's conceivable that data annotation will grow increasingly specialized and application-specific for particular retail use cases. The development of tailored annotation methods will take into account the particular difficulties and needs posed by various forms of retail data, including text, image, and sensor data. AI models with greater accuracy and greater capacity to manage the intricacies of the retail environment will be produced as a result of this specialization.
Data annotation appears to have a bright future in retail AI adoption. To remain competitive in the ever-changing retail sector, businesses must invest in high-quality annotated data as they depend more and more on AI technology to spur development and innovation. Retailers may seize new chances for personalization, optimization, and operational efficiency by implementing advanced data annotation tools and processes.