1. Introduction
Introduction: Data lineage is the record of data's origin, movement, changes,and transformations throughout its lifecycle. It provides a historical view that traces the journey of data from its source to its destination. Understanding data lineage is crucial for businesses as it offers transparency, accountability, and reliability in data-driven decision-making processes. By knowing where the data comes from, how it has been manipulated, and who has accessed it, organizations can improve data quality, regulatory compliance, and overall trust in their data assets.
Importance of understanding the history of data:
Gain insights into how data is used
Ensure compliance with regulations
Enhance data quality and accuracy
Mitigate risks associated with erroneous or outdated information
Improve decision-making processes based on accurate and reliable data
Facilitate troubleshooting and debugging in case of errors or discrepancies🟢
Enhance collaboration among different teams working with the same datasets
2. The Basics of Data Lineage
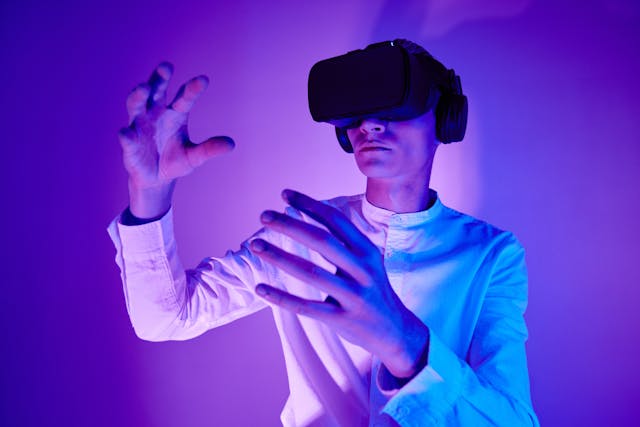
The term "data lineage" describes the entire documentation of every data movement that occurs inside an organization, including the origins, uses, and destinations of the data. Transparency into the data's flow and transformation is provided by following the data from its point of origin via a number of systems and processes. For a number of reasons, including regulatory compliance, maintaining data quality, facilitating better decision-making, and advancing general data management procedures, organizations must monitor data lineage.
Organizations can establish trust in the accuracy and reliability of their information and have a deeper understanding of their data assets by maintaining data lineage. Better traceability in the event of errors or discrepancies is made possible by this transparency, which facilitates prompt problem identification and resolution. Organizations can find process inefficiencies, optimize their data architecture, and enhance operational performance by tracking data lineage.
There are many advantages to preserving data lineage. By offering a transparent audit trail of data usage and guaranteeing compliance with legal standards like GDPR or HIPAA, it supports compliance initiatives. By enabling businesses to track changes to their data across time and identify abnormalities or inconsistencies, data lineage improves the quality of data. Proactive data management results in more trustworthy insights for making decisions.🤝
In order to properly utilize their information assets, modern firms must establish and maintain strong data lineage processes. Businesses can seize new chances for innovation, optimization, and strategic expansion by knowing where their data originates from and how it is used across the whole enterprise."
3. Data Lineage in Business Operations
Data lineage influences decision-making processes, which is important for company operations. Comprehending the data's past enables firms to make well-informed decisions grounded in precise insights and dependable data. Stakeholders can trust the integrity of the information and make more confident decisions that lead to company success when they are aware of how the data has been gathered, processed, and used.
Maintaining data lineage is crucial to guaranteeing the accuracy and dependability of data inside a company. Businesses can spot any problems like errors or inconsistencies that could affect decision-making processes by tracking the sources and transformations of data throughout its lifecycle. Companies can meet regulatory compliance standards and uphold their customers' trust by maintaining a clear lineage of data. Customers expect accurate and secure management of their information. Strong lineage tracking improves data governance procedures, encouraging accountability and transparency in all areas of the business.
4. Implementing Data Lineage Tools and Processes
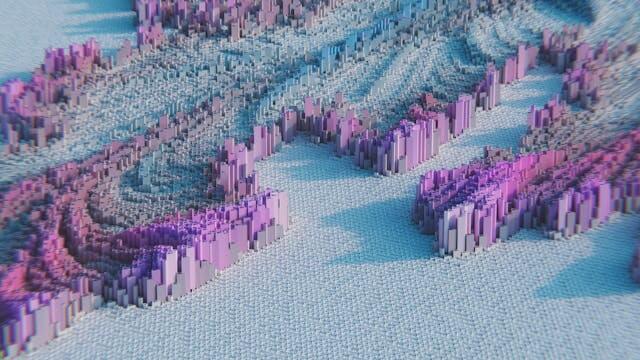
For companies trying to trace the origins and significance of their data, putting data lineage procedures and tools into place is essential. Organizations can manage and trace their data provenance more efficiently with the help of a variety of technologies on the market. Waterline Data, Apache Atlas, Collibra, and Informatica are a few of the well-liked choices.
To ensure the success of data lineage processes, it is imperative to adhere to best practices during their establishment. Clear data governance principles, thorough documentation of data flows, the application of metadata management techniques, frequent audits of data lineage procedures, and the promotion of cooperation amongst various data management teams are a few important pointers.
Businesses can improve data quality and integrity, comply with regulatory requirements, reduce the risk of inaccurate or incomplete data, and ultimately make better decisions by utilizing these tools and adhering to best practices. Businesses can also gain deeper insights into their data ecosystem.
5. Regulatory Compliance and Data Lineage
One of the most important aspects of data management is regulatory compliance, and data lineage is essential to meeting regulatory obligations. Through the process of tracking data origins and transformations, entities can exhibit data processes that are transparent, accurate, and accountable. Regulators frequently demand that companies demonstrate a thorough grasp of the collection, storage, and use of data. This visibility is made possible by data lineage, which illustrates the full data journey from source to destination.
Numerous laws stress how crucial data lineage is to guaranteeing compliance. For instance, companies are required by the General Data Protection Regulation (GDPR) to keep accurate records of all activities related to the processing of personal data. Data lineage enables firms to efficiently respond to regulatory inquiries by tracking the movement of personal data between systems and processes. In a similar vein, laws such as the Sarbanes-Oxley Act (SOX) mandate that companies have controls over their financial reporting procedures. Data lineage provides insights into the acquisition, transformation, and reporting of financial data, which helps develop these controls.
Regulations like the Health Insurance Portability and Accountability Act (HIPAA) in the healthcare sector demand stringent protections for patient health information. Data lineage shows how patient data is handled inside healthcare providers' systems, which helps them stay in compliance. Through data lineage documentation, enterprises can guarantee compliance with privacy rules and avert unapproved access or security breaches by recording every sensitive information touchpoints.
Basel III and similar laws, which are designed to improve risk management procedures in banks, are mandatory for financial institutions to follow. Through data lineage, these institutions may chart the flow of risk-related data through their operations and develop a comprehensive perspective of their risk exposure. In addition to guaranteeing regulatory compliance, this openness improves decision-making procedures by offering insights into possible hazards connected to certain datasets.
Strong data lineage procedures help firms comply with regulations and make well-informed decisions, strengthen data quality control systems, and increase their general credibility while managing sensitive data, in addition to helping them comply with legal obligations. Businesses can reduce the risks associated with non-compliance and more adeptly navigate complicated compliance environments by acknowledging the importance of data lineage in regulatory frameworks.
6. Data Lineage Case Studies
Businesses must comprehend data lineage in order to guarantee data quality, accuracy, and compliance and to make well-informed decisions. The following are actual instances of how companies have profited from knowing their data history:📄
1. **Financial Services**: By tracking the origins of its data across several platforms, a well-known financial institution enhanced its risk management procedures. The company's comprehension of the transformation and utilization of data during its entire lifecycle allowed it to better comply with regulations, spot possible obstructions, and make better decisions.
2. **Healthcare**: By examining the history of electronic health records (EHRs), a healthcare provider improved the way it provided patient care. The organization ensured better patient outcomes, decreased medical documentation errors, and highlighted opportunities for improvement in care coordination by monitoring the flow of data from initial patient intake to diagnosis and treatment plans.
3. **Retail**: To optimize its supply chain processes, a multinational retail chain used data lineage insights. The organization reduced stockouts, improved stock management, and customized marketing campaigns by mapping the sources of product data, including sourcing information, inventory levels, and consumer sales trends.
These case studies show how a thorough understanding of data lineage may lead to improved corporate performance across a range of industries by promoting operational efficiency, regulatory compliance, and strategic decision-making. Organizations can gain important insights that foster innovation and expansion by tracing the history of their data assets.
7. Challenges and Solutions in Data Lineage Implementation
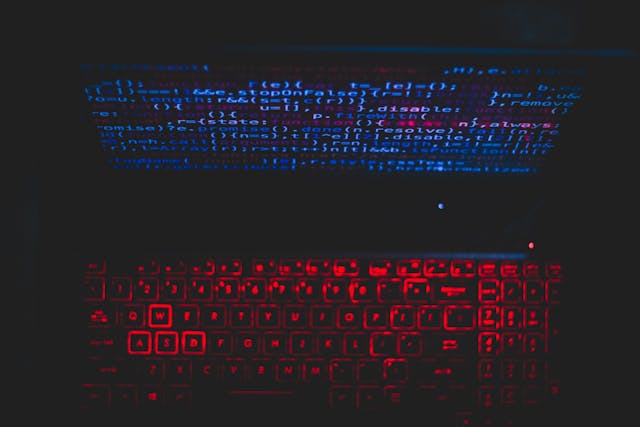
Lack of standard data formats, inconsistent data quality across sources, and complicated data ecosystems are common problems that make implementing comprehensive data lineage difficult. Overcoming siloed data environments and ensuring standard metadata management procedures are frequent challenges. Organizations can begin addressing these issues by developing cross-functional collaboration and well-defined data governance policies to guarantee alignment on data standards.
By putting in place a metadata library, metadata management can be centralized and a uniform view of data lineage across systems can be obtained. Processes can be streamlined and manual errors can be decreased by using automation tools for tracking data transformations and gathering lineage information. In the long run, lineage information correctness and dependability can be guaranteed by implementing frequent data quality checks and audits.
For data lineage implementation to be successful, cooperation between IT, data engineering, and business stakeholders is necessary. Organizations can build trust in the precision and integrity of their data lineage by cultivating a culture of responsibility and openness around data consumption and transformation procedures. The lineage is kept current and reflects the most recent condition of the data ecosystem thanks to ongoing monitoring and documenting of changes made to data pipelines.
8. Future Trends in Data Lineage
Understanding data lineage is crucial since businesses are depending more and more on data to make decisions. Future developments in artificial intelligence, machine learning, and blockchain are expected to have a big impact on data lineage procedures. These technologies have the potential to improve data quality, automate metadata management, and increase data traceability.
Data lineage is expected to experience significant evolution in the upcoming years. Using AI algorithms to improve lineage tracking and analysis is a major trend. Organizations will be able to quickly and accurately automate the detection of dependencies across large datasets thanks to this. Technological developments in big data and cloud computing will further simplify data lineage procedures, increasing their scalability and efficiency.
An even more important factor in the future of data lineage will be the growing emphasis on data governance and regulatory compliance. Global data protection standards are becoming more stringent, and companies will require strong data lineage capabilities to prove accountability and maintain compliance. As a result, more complete solutions that provide end-to-end visibility into the information flow within an organization are to be expected.
Future developments in data lineage indicate that increasingly advanced technologies will provide deeper understanding of the flow of data throughout an organization. Businesses can guarantee reliability and openness in their utilization of data resources, as well as enhance their decision-making procedures, by using these developments.
9. Data Lineage and Advanced Analytics
In order to advance analytics capabilities within enterprises, data lineage is essential. Businesses may achieve unprecedented levels of success with their machine learning and predictive modeling operations by leveraging the power of data lineage. Gaining a thorough understanding of the origins, transformations, and movements of data sets the stage for producing insights and forecasts that are more accurate.
Data lineage serves as a blueprint for advanced analytics, guiding analysts through the complex process of data processing. Organizations can guarantee the accuracy and dependability of their analytical outputs by documenting each piece of information's historical development and returning to its original source. With this all-encompassing perspective, data scientists may make well-informed judgments based on a thorough comprehension of the manipulation and use of data during its entire lifecycle.
By using data lineage for advanced analytics, businesses can find relationships, trends, and correlations that might not be immediately obvious. Through identifying the connections between diverse information and analytical techniques, companies can unearth untapped insights that propel innovation and gain a competitive edge. Advanced analytics procedures that integrate a thorough data lineage approach improve model relevance and accuracy, producing more reliable forecasts and tactical suggestions.
In the field of machine learning and predictive modeling, thorough data lineage is essential for developing highly accurate and dependable algorithms. Data scientists can enhance the performance and generalization capacities of their models by adding historical context into the training and validation phases. A deeper comprehension of the structure, cleaning, and transformation of historical data enables more accurate feature selection, which improves prediction accuracy and decision-making.
In summary, companies can fully utilize their data assets by incorporating data lineage into sophisticated analytics procedures. In addition to improving the caliber of insights obtained from analytical procedures, this holistic viewpoint promotes an environment of openness and confidence in data-driven decision-making. In order to stay ahead of the competition in today's market, firms must build a strong foundation based on thorough data lineage, since they will increasingly rely on advanced analytics tools to spur growth and innovation.
10. The Role of AI in Data Lineage Management
AI is essential to the transformation of data lineage management in the corporate world. Organizations are able to track and analyze their data history more effectively than ever before by utilizing AI technologies. With AI, data flow can be automatically recorded, tracked, and monitored throughout its entire lifecycle, offering a complete picture of how data is utilized and changed inside a company.
Increased accuracy and speed are two important advantages of utilizing AI to improve data lineage procedures. Artificial intelligence (AI) systems can swiftly sort through enormous volumes of data to find connections and links, which minimizes the chance of human mistake and manual labor in lineage tracking. Businesses can take proactive measures to address problems before they get out of hand by using AI to assist them find abnormalities or inconsistencies in their data lineage.
But there are drawbacks to incorporating AI into data lineage management as well. In order to minimize biases or mistakes that could influence decision-making processes, rigorous validation and oversight are necessary to ensure the quality and dependability of AI-driven insights. It might be essential to make large investments in hiring staff training and purchasing the technical infrastructure required to enable sophisticated analytics skills in order to implement AI solutions.
The use of AI to improve data lineage procedures has advantages and disadvantages, but for companies trying to effectively leverage their data history, the potential gains in productivity, accuracy, and decision-making skills make the investment worthwhile.
11. Maintaining Data Privacy with Comprehensive Data Lineage
In the current digital landscape, where data breaches are a major worry, maintaining data privacy is essential. A thorough data lineage is essential for this since it not only tracks the sources and modifications of data but also makes sure that private information is kept safe during the entire process. Organizations can meet regulatory compliance requirements and effectively protect their precious data assets by using strong data lineage processes. 💎
Businesses must be careful in knowing how their data is gathered, kept, shared, and used across numerous systems and applications due to the regulatory scrutiny surrounding data privacy that is growing. By tracing the path of every piece of data, comprehensive data lineage offers transparency into these areas and helps businesses recognize and address security threats or vulnerabilities that can endanger sensitive data.
Ensuring that only authorized workers can view or alter sensitive data is made possible by strong data lineage policies, which enable organizations to build efficient access controls and encryption measures. Businesses can improve their overall security posture and gain the trust of clients who entrust them with their personal information by establishing stringent governance procedures and meticulously documenting the flow of data.
Taking into account everything said above, we can say that protecting data privacy entails more than just putting strong cybersecurity safeguards in place; it also entails knowing your data's past through thorough lineage monitoring. Through the implementation of robust data lineage processes and the protection of sensitive information, businesses may effectively mitigate possible risks and optimize the value of their data assets in a secure and compliant manner.
12. Conclusion: The Future Impact of Understanding Your Data's Past
From the foregoing, it is clear that knowing your company's data lineage and history can have a significant influence on future strategic choices. Understanding the origins, transformations, and destinations of your data will help you improve data quality, guarantee compliance, and build confidence in your data-driven insights. Using data lineage facilitates error detection in a timely manner, process improvement, efficient resource allocation, and the creation of a more flexible and responsive company environment. In today's data-driven world, utilizing the potential of historical data fosters innovation and creates a competitive advantage.