1. Introduction to Data Maturity Map
Knowing where your company stands in terms of data maturity in the big data space is essential for making wise decisions and developing winning strategies. A data maturity map is a tool used to evaluate how well an organization is handling data at the moment, from basic data collecting to the use of complex analytics. This map helps identify strengths, flaws, and places for improvement within the data infrastructure.
Organizations can advance through the various phases or levels of a Data maturity map as they improve their capacity for efficiently handling and exploiting data. From simple data collecting and storage to predictive modeling and artificial intelligence application, each level denotes a rise in data practice sophistication. Businesses can develop a plan for methodically advancing their data strategy by comprehending these tiers.
Assessing many facets of data management, including data quality, governance, analytical expertise, and technological integration, is necessary when creating a data maturity map. Organizations can learn more about how well they use their data assets for innovation and decision-making by taking this assessment. The organization's current position on the path to becoming a data-driven company is clearly visualized by the map.
In addition to achieving greater degrees of sophistication, creating a strong Data Maturity Map also involves making sure that business goals and data capabilities are in line. Organizations may focus investments in areas that will have the biggest effects on their overall performance and competitiveness by outlining the existing situation and establishing targets for future developments. Watch this space for the next section, when we explore in more detail the various stages of maturity seen in a data maturity map.
2. Understanding the Importance of Data Maturity
Businesses trying to develop successful big data strategies must recognize the significance of data maturity. An organization's capacity to oversee, manage, and extract meaningful insights from its data assets is reflected in its data maturity. It includes a number of elements, including analytics capabilities, data integration, quality, and general data governance procedures.
Businesses can increase operational effectiveness, make better decisions, and obtain a competitive advantage in the market with a well-developed data ecosystem. Organizations can fully utilize their data resources to spur innovation, streamline operations, and improve customer experiences when they have a higher level of data maturity. Companies can use a well-defined data maturity roadmap to help them prioritize their investments in technologies, people development, and data infrastructure.
Organizations can discover areas for improvement by evaluating their present data maturity level and comprehending the effects it has on business processes. Companies are able to customize their big data initiatives to meet their unique demands and objectives because of this self-awareness. Businesses may better match their goals with changing technological trends and regulatory needs as they advance through different stages of data maturity. This helps them stay ahead in the rapidly expanding digital ecosystem of today.
3. Building Blocks of a Data Maturity Map
To guarantee that a Data Maturity Map is effective in directing an organization's data strategy, a number of building pieces must be included. These elements aid in determining the organization's present state of data maturity and offer a path forward for advancing toward more advanced stages of maturity. The following are the main components of a data maturity map:
1. **Framework for Assessment:** In order to examine the organization's current data capabilities in terms of data quality, governance, analytics, and infrastructure, a thorough evaluation framework must be developed. The organization's data environment may be understood in terms of its strengths, shortcomings, and opportunities for change thanks to this framework.
2. **Stages of Maturity:** The organization's data maturity can be categorized from ad hoc and reactive processes to proactive and strategic approaches with the use of clearly defined maturity levels. Organizations might establish attainable objectives for gradually improving their data capabilities by defining different levels of maturity.
3. **Indicators of Performance (KPIs):** Establishing pertinent KPIs that correspond with every maturity level helps firms monitor their performance against predetermined standards and gauge their growth. These metrics offer measurable measures of achievement and support the validity of the results of programs meant to improve data maturity.
4. **Developing the Roadmap:** Developing a comprehensive plan that includes concrete actions and deadlines is crucial for implementing ways to progressively increase data maturity levels. To guarantee conformity with company goals, the roadmap should include prioritized tasks, timetables, resource allocations, and dependencies.
5. **Plan for Change Management:** A strong change management strategy must be put into place to ensure that transitions to higher data maturity levels go smoothly. Ensuring the successful adoption of new practices throughout the business requires addressing cultural shifts, engaging stakeholders, developing communication strategies, and implementing training initiatives.
Organizations may obtain useful insights into their present data capabilities and map out a clear route towards enhanced decision-making, operational efficiency, and innovation driven by data-driven practices by including these building elements into the development of a Data Maturity Map.
4. Assessing Current Data Practices
One of the most important steps in creating a solid big data strategy is evaluating current data practices. Doing a comprehensive audit of your organization's current data sources, storage practices, and analytical capabilities will help you determine where it stands in terms of data maturity. Examine the ways in which data is gathered, handled, saved, and applied throughout the organization's many departments and systems.
Analyze the timeliness, correctness, completeness, and relevance of your data to determine its quality. Determine whether the organization has any data duplication or silos. Examine the security protocols in place to guard sensitive information and make sure they comply with applicable laws like HIPAA and GDPR.
To learn more about the ways in which important stakeholders now use data in their decision-making processes, think about conducting surveys or interviews with them. This might assist you in comprehending the potential and problems that currently surround the use of data within your company.
You can find opportunities for improvement and set the foundation for creating a more developed and successful big data strategy that is in line with your company objectives by carefully evaluating your present data practices.
5. Defining Goals and Objectives for Data Strategy
We explore the crucial stage of establishing goals and objectives for your data strategy in the second installment of our series on big data strategy. This stage is crucial because it establishes the direction and synchronizes your organization's data initiatives with your business goals. To guarantee responsibility and clarity, your goals should be SMART (specific, measurable, achievable, relevant, and time-bound).
Start by evaluating your present organizational preparedness, analytical capabilities, and data architecture. Determine the main obstacles and possibilities present in your data environment. Utilize this research to set specific objectives that target these areas for development, or use your current advantages to create value.
To acquire varied viewpoints that complement the overall business objectives, think about including stakeholders from several departments. Working together with important decision-makers guarantees that the aims of the data strategy are supported at all levels and are linked to larger organizational goals.
As you define your objectives, remember to prioritize them based on their impact on business outcomes. Every objective, whether it's improving customer satisfaction, streamlining processes, or facilitating data-driven decision-making, should directly advance the strategic direction of your company. Make sure that everyone in the organization is working toward the same success vision by effectively communicating these priorities to all of the departments.
To put it succinctly, the first step in properly utilizing the power of big data is to define specific, attainable goals and objectives for your data strategy. You may design a roadmap that extracts true value from your data assets by prioritizing initiatives according to their strategic significance, incorporating key stakeholders in the process, and creating SMART goals that are tightly tied to your company objectives. We'll be exploring the execution phase of a successful big data strategy in our future installment, so stay tuned.
6. Implementing Strategies for Advancing Data Maturity
Putting strategies in place to promote data maturity requires a methodical approach that is customized to the aims and actual situation of a business. Starting with the data maturity evaluation, it determines the main areas that need improvement. Measuring success requires defining precise goals and measures to monitor development. Establishing a specialized team to lead these projects guarantees targeted efforts are made toward reaching data maturity benchmarks.
Utilizing tools and technology that correspond with the organization's aims is vital. Investing in automation technologies, scalable infrastructure, and data analytics platforms can improve decision-making powers and expedite procedures. Employees who receive training on data literacy and best practices are more equipped to use data in their jobs and help the firm as a whole develop a data-driven culture.
Ensuring data security, compliance, and quality requires the establishment of strong data governance structures. Conducting routine evaluations and audits facilitates the identification of deficiencies and guarantees compliance with set protocols. Involving stakeholders from other departments promotes cross-functional knowledge sharing and innovation while fostering collaboration and alignment toward shared data goals.
Regular evaluations and feedback loops enable ongoing progress tracking, allowing for any necessary strategy revisions. Honoring successes and giving credit to those who push the boundaries of data maturity can boost interest and dedication to creating an organization that is data-driven. In the constantly changing field of big data analytics, businesses can seize fresh chances for growth and innovation by cultivating a culture that views data as a strategic asset.
7. Tracking Progress and Iterating on the Data Maturity Map
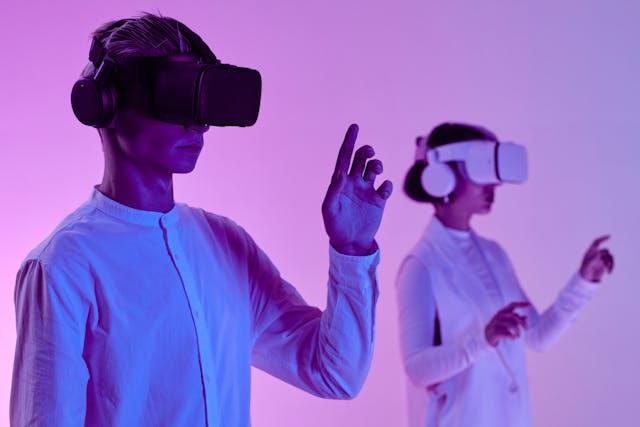
A key component of developing your big data strategy is monitoring your progress and making adjustments to the data maturity map. Through consistent observation of your company's progress through the several phases of data maturity, you may pinpoint areas in need of development and modify your approach accordingly. You can efficiently adjust to shifting market conditions, technological advancements, and business needs thanks to this iterative approach.
Establishing key performance indicators (KPIs) in line with each data maturity map stage is a useful approach to monitor development. These KPIs could track things like the overall business impact, accessibility, quality, and consumption of the data. Reviewing these indicators on a regular basis gives you important information about how well your company is doing in terms of achieving its objectives for data maturity.
Updating the data maturity map iteratively entails taking into account fresh insights, obstacles faced, and triumphs realized throughout the execution of your big data plan. You might find that there are more stages or subtleties in the ones that are already there that need to be included in the map as you collect more information and insights. Your data maturity framework will always be current and in line with your company's goals thanks to this ongoing improvement.
To make sure your data maturity map is applicable and useful, you should actively seek input from stakeholders in all departments. Their advice may reveal ignored details or blind spots that could have a substantial influence on the outcome of your big data projects. Working together with a variety of stakeholders encourages a sense of ownership and dedication to enhancing data practices throughout the company.
After reviewing the material above, we can draw the conclusion that businesses may manage and utilize their data assets more effectively over time by continuing to monitor their progress and make iterations to the data maturity map. In the quickly changing digital landscape of today, organizations may stay ahead by adopting these iterative methods and staying flexible.
8. Case Studies: Successful Implementation of Data Maturity Maps
Case studies provide practical instances of data maturity map implementation gone right. These anecdotes show how companies used their data maturity maps to drive improvements and accomplish their strategic goals.
Company X, a global company in the retail industry, is one interesting case study. Company X was able to pinpoint important areas where their data skills were lacking and create a plan for progress by putting a data maturity map into practice. By making focused investments in talent development and technology, they greatly improved their data analytics skills. Better customer insights, better decision-making, and eventually more profitability resulted from this.
Organization Y, a healthcare provider, used data maturity mapping in another case study to improve the efficiency of their patient care procedures. They found bottlenecks in information flow and decision-making by examining their data maturity levels across departments. They reorganized their data management procedures and introduced new technology as a result of this realization to guarantee prompt access to vital patient data. Consequently, Organization Y witnessed decreased wait times, enhanced patient results, and increased levels of staff satisfaction.😡
These case studies demonstrate how, when used carefully and deliberately, data maturity mapping may have a revolutionary effect. They show how businesses may seize new chances for creativity, efficiency, and competitiveness in today's data-driven world by figuring out where they are in their data journey and setting up a growth trajectory.
9. Overcoming Challenges in Developing a Robust Data Strategy
Organizations frequently run across a number of obstacles while trying to build a strong data strategy, which can impede their progress. The absence of specific goals and objectives for data management and exploitation is a significant obstacle. It becomes challenging to successfully coordinate data projects with business goals in the absence of a clear strategic direction.
The problem of governance and data quality is another frequent difficulty. Inaccurate analysis and decision-making resulting from low-quality data might undermine the efficacy of the data strategy as a whole. In order to guarantee data security, quality, and integrity across the whole enterprise, strong data governance procedures must be established.
Creating a coherent data strategy can be seriously hampered by organizational silos and outdated systems. Breaking down these silos and connecting diverse technologies are key stages in developing a cohesive data ecosystem that supports the organization's goals effectively.🫡
The development of a strong data strategy may be hampered by a lack of qualified personnel in data management and analytics. This problem may be solved and a solid basis for fruitful data projects can be established by investing in staff training and upskilling or forming alliances with outside specialists.
Last but not least, enterprises trying to improve their data strategies constantly face the problem of keeping up with the quickly changing landscape of technology and market trends. In a constantly changing digital environment, keeping up with new developments in Big Data tools, practices, and laws is essential to maintaining the relevance and efficacy of the data strategy. Organizations may overcome difficulties and create a strong data strategy that promotes innovation, efficiency, and growth throughout the whole enterprise by proactively tackling these challenges. 👓
10. Future Trends in Big Data and Implications for Data Maturity Maps
In evaluating the future trends in big data and their consequences for data maturity maps, it is vital to evaluate the dynamic environment of technology and data-driven decision-making. Going forward, a number of significant themes are anticipated to influence how businesses approach big data strategies:
1. **AI and Machine Learning Integration**: The integration of artificial intelligence (AI) and machine learning into big data analytics will continue to drive improvements in predictive and prescriptive analytics. The increasing impact of these technologies on data processing and analysis will need to be taken into consideration in data maturity maps.
2. **Edge Computing**: Edge computing will be crucial in processing data closer to its source as a result of the proliferation of IoT devices and the enormous volumes of data they produce. Maps of data maturity should take into account how businesses may best use edge computing capabilities.
3. **Data Privacy and Security**: Ensuring data privacy and security will be crucial for enterprises working with big data as laws like the CCPA and GDPR become increasingly strict. Data maturity maps must take into account best practices for safeguarding sensitive data as well as compliance needs.
4. **Ethical Data Use**: Organizations need to give ethical principles top priority in their big data initiatives since ethical considerations around data collection and utilization are coming under more scrutiny. Data maturity maps should integrate frameworks for responsible data practices.😽
5. **Data Democratization**: More workers now have access to insightful information thanks to the trend in firms toward democratizing data access. Data maturity maps can be used to assist define procedures that guarantee public access while upholding data governance and quality.
6. **Real-Time Analytics**: As companies strive to make choices more quickly by utilizing the most recent information, there is an increasing need for real-time analytics. Real-time processing and analysis skills should be highlighted in future data maturity maps.
7. **Blockchain Integration**: Data may be shared and stored in a transparent and safe manner thanks to blockchain technology. Organizations may need to make changes to how they map out their data maturity levels in order to incorporate blockchain into big data strategy.
8. **Hybrid Cloud Solutions**: As hybrid cloud solutions become more common, businesses must manage complicated environments that combine public and private cloud services with on-premises infrastructure. Data maturity maps can aid firms in optimizing their hybrid cloud installations.
9. **Data Monetization Practices**: Businesses are looking into joint ventures, direct sales, and other arrangements as means of generating revenue from their data assets. Organizations hoping to make new uses of their data could find routes included in data maturity maps.
By considering these future trends in big data, organizations can better prepare themselves by updating their approaches outlined in their respective Data Maturity Maps accordingly.