1. Introduction
The success of organizations across all industries is heavily dependent on the quality of data in the current data-driven environment. Accurate and trustworthy data is essential for fostering innovation, obtaining a competitive edge, and making well-informed decisions in any industry, including healthcare, banking, retail, and others. Even so, a lot of businesses still have trouble guaranteeing the accuracy of their data. This may result in expensive mistakes, lost opportunities, and reputational harm. In this blog post, we look into the importance of data quality and why being in the dark about it might prove damaging in the long term.
2. The Basics of Data Quality
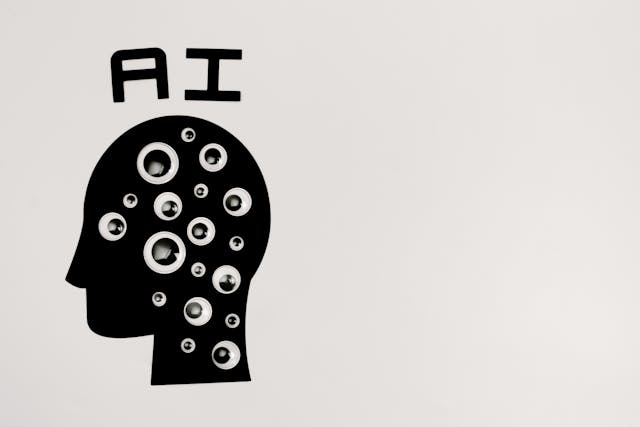
The accuracy, consistency, relevance, and completeness of the data in a dataset are referred to as data quality. It is essential because low-quality data can result in poor decision-making, unsuccessful business plans, and lost growth possibilities. Reliability in decision-making is ensured by high-quality data, which boosts operational effectiveness and facilitates improved customer service for enterprises. Essentially, data quality is about ensuring that the data being utilized is fit for its intended purpose.
Data quality is important for more than simply organizational decision-making; it affects many different facets of corporate operations. For example, tailored services and targeted marketing strategies depend on precise client data. Incomplete or inaccurate consumer data can lead to resource waste and lower customer satisfaction. Sustaining excellent data quality is essential for regulatory compliance and protecting the confidentiality and privacy of sensitive information in sectors like banking and healthcare.
To put it simply, data quality is the cornerstone around which companies develop their plans, base choices, and spur expansion. Organizations may maximize the value of their data assets and maintain a competitive edge in the current market by knowing what quality data is and investing in procedures to keep it that way.
3. Common Challenges with Data Quality
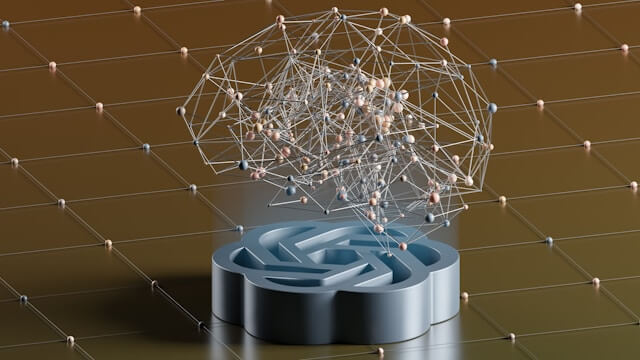
Businesses that want to keep high-quality data frequently face a number of difficulties. Data inconsistency, which occurs when information is entered in various formats across systems and causes errors and inaccuracies, is one prevalent problem. Another common issue is data duplication, which can lead to confusion and resource waste because the same data is used in various versions.
Completely lacking data is another major barrier. It is more difficult to get precise insights or come to well-informed conclusions when crucial fields are either blank or only partially completed. Outdated information that no longer accurately represents the situation can also lead to poor data quality, which can affect the applicability and consistency of analytics.
For businesses, maintaining regulatory compliance and data security is a constant problem. Robust safeguards and continuous vigilance are necessary to secure sensitive data from breaches or unauthorized access while complying with ever-changing data protection standards. To tackle these prevalent obstacles, one must have a proactive stance towards data management and establish efficient procedures and technology to uphold superior data standards.
4. Impact of Poor Data Quality
Inadequate data quality can significantly affect analytics, decision-making, and overall corporate performance. Decisions based on incomplete or erroneous data might result in incorrect conclusions and misapplied tactics. Inaccurate information can also lead to resource waste since decisions made may not be in line with the actual circumstances.
Inadequate data quality can distort analytics results, producing false insights and conclusions. This can erode confidence in data-driven decision-making procedures and impede an organization's ability to innovate and expand. Businesses might not be able to correctly recognize trends, opportunities, or risks without trustworthy data.
Deficient data quality has an effect on the performance of businesses as a whole. Inaccurate data can result in lost company opportunities, increased expenses from errors or rework, customer unhappiness, and operational inefficiencies. A company's reputation may also suffer if stakeholders come to doubt its reliability as a source of factual information.
For organizations to succeed in today's data-driven environment, gain meaningful insights through analytics, and make well-informed decisions, it is imperative that high-quality data is maintained.
5. Strategies for Improving Data Quality
Improving data quality is essential for businesses to make informed decisions and gain valuable insights. Here are some strategies to enhance the quality of your data:
1. **Implement Data Quality Tools:** Invest in data quality tools that can help identify and correct errors, ensure consistency, and maintain accuracy in your data sets.
2. **Establish Data Quality Standards:** Clearly define data quality standards inside your company to guarantee that all data meets predetermined requirements like timeliness, accuracy, completeness, and consistency.
3. **Data Validation Procedures:** Implement robust data validation procedures to catch errors at various stages of the data lifecycle, from inputting data to processing and reporting.
4. **Regular Data Cleaning:** Set up regular data cleaning processes to remove duplicates, outdated information, and irrelevant data that can compromise the overall quality of your dataset.
5. **Train Your Team:** Provide training to your team members on best practices for maintaining high-quality data standards and emphasize the importance of accurate data entry and management.
6. Keep an eye on data quality metrics: Set up key performance indicators (KPIs) to keep an ongoing eye on the accuracy of your data. This will assist you in spotting problems early and acting quickly to fix them.
7. **Data Governance Framework:** Create a solid framework for data governance that explains the roles, duties, guidelines, and practices involved in maintaining and guaranteeing the accuracy of organizational data.
8. **Collaborate Across Departments:** To guarantee a common knowledge of data quality requirements and best practices, encourage collaboration between departments involved in data collection, analysis, and use.
By implementing these strategies proactively, organizations can improve the overall quality of their data assets and leverage them effectively for driving business success.
6. Tools and Technologies for Data Quality Management
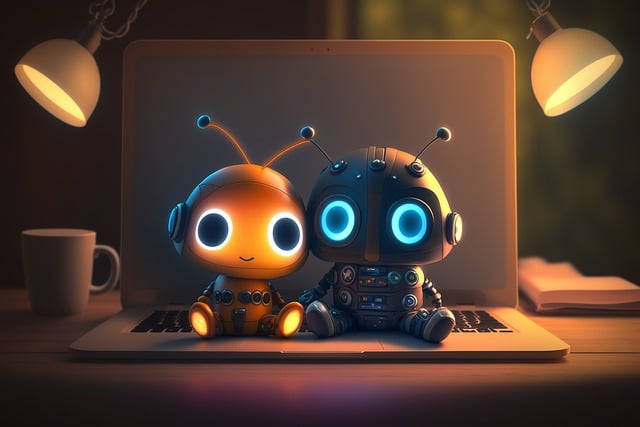
Businesses may guarantee the accuracy, consistency, and dependability of their data using a wide range of tools and technologies available to them in the field of data quality management. One key tool is data profiling software, which helps firms understand the structure and content of their data. This enables companies to find flaws, irregularities, and anomalies that could compromise the quality of their data.
Master Data control (MDM) systems are another important piece of technology for data quality control. By offering a single source of truth for all important business data, these systems guarantee that there is only one source of information used throughout the whole company. Businesses can enforce standards and norms and retain high-quality data by implementing data governance rules within MDM systems.
Tools for data purification are also necessary to enhance the quality of the data. By eliminating duplication, standardizing formats, and adding missing values, these tools assist in locating and fixing mistakes in datasets. Businesses can improve decision-making procedures and boost operational effectiveness by routinely cleansing their data.
Tools for automated data monitoring are essential for preserving data quality over time. These technologies monitor important data quality parameters continually and send out real-time notifications when problems occur. Organizations can proactively address any issues before they affect business operations by utilizing such technologies. 😼
Businesses can extract valuable insights from their data by utilizing advanced analytics solutions, such as machine learning algorithms, which can also detect patterns or outliers that may suggest substandard data quality. Businesses are able to successfully anticipate and prevent future issues by using predictive analytics into their data quality management approach.
Businesses can improve the quality of their data by taking proactive measures by utilizing these various tools and technologies. All of these technologies—profile software, MDM systems, automated monitoring tools, and advanced analytics solutions—are essential to guaranteeing that businesses have access to consistent, dependable data for strategic planning and well-informed decision-making.
7. Best Practices for Data Quality Assurance
Implementing a robust data quality assurance process within an organization is essential for ensuring accurate and reliable data for decision-making. Here are some best practices to consider:
1. Establish Unambiguous Data Quality Standards: Clearly define the measurements and standards that will be used to evaluate data quality throughout the company. This can involve timeliness, accuracy, consistency, completeness, and integrity.
2. Implement Data Profiling Tools: Utilize data profiling tools to assess and understand the quality of your data. Outliers, missing values, duplication, and other problems that can affect the overall quality of your data can be found with the aid of these tools.
3. Schedule Regular Data Cleaning: To maintain your data current and clean, schedule routine data cleansing tasks. This may entail clearing out duplicates, fixing mistakes, standardizing formats, and updating out-of-date data.
4. Implement Data Governance Policies: Create policies outlining who is responsible for what in terms of upholding data quality standards. Accountability is ensured throughout the company in this way.
5. Conduct Data Quality Audits: To evaluate the efficacy of your data quality assurance procedures, conduct data quality audits on a regular basis. Determine where you need to improve, then make the necessary corrections.
6. Invest in Training: Educate staff members on best practices for data management and the value of preserving high-quality data. This contributes to the development of an awareness of data quality throughout the company.
7. Leverage Automation: To expedite the procedures involved in data quality assurance, use automation technologies whenever feasible. Automation can guarantee constant adherence to data quality standards and assist lower manual mistake rates.
In today's data-driven world, companies may create a strong basis for preserving high-quality data that supports well-informed decision-making and economic success by adhering to these best practices.
8. Case Studies: Success Stories in Data Quality Improvement
Case Studies: Success Stories in Data Quality Improvement
Empirical instances demonstrate the observable advantages of allocating resources towards enhancing data quality. Inaccurate customer data was a problem for Company X, which resulted in lost sales opportunities and irate customers. They increased customer satisfaction by 20% and streamlined their operations by putting in place a data quality management system.
Company Y struggled with duplicate records in its database, which led to resource waste and disorganized marketing campaigns. By implementing deduplication techniques and data cleansing programs, they were able to decrease marketing expenses by 30% while increasing campaign effectiveness by 25%.
Disparate departments' disparate data formats presented problems for Company Z and impeded their ability to make decisions. They saw a 15% boost in operational efficiency and more accurate reporting capabilities after standardizing their data governance procedures and imposing tougher data entry requirements.
These success stories demonstrate how giving data quality top priority inside firms can have a profoundly positive impact. Through proactive resolution of critical problems and creative thinking, businesses can open up new avenues for expansion, cost reduction, and enhanced overall efficiency.
9. Future Trends in Data Quality Management
In the field of data quality management, several potential trends are defining the future landscape. The growing significance of data governance frameworks—which guarantee that data is reliable, safe, and consistent with legal requirements—is one such trend. Businesses are becoming more and more aware of how important strong governance is to preserving high-quality data.
Data quality management is also being revolutionized by AI and machine learning technology. By automating the process of locating and fixing problems with data quality, these technologies enable businesses to use their data more effectively and efficiently. Businesses can proactively solve issues with data quality before they have an influence on decision-making processes by utilizing AI and ML.
The emergence of blockchain technology as a means of improving data quality is another topic to watch. Blockchain's decentralized and unchangeable nature makes it suitable for protecting the integrity and validity of data. Organizations can build trust in their transactions and data sources by utilizing blockchain, which will ultimately improve the overall quality of their data.
Scalable solutions are essential to handle the flood of heterogeneous data that businesses are collecting from a multitude of sources in increasingly large numbers. In the future, data quality management systems that can process vast amounts of inconsistent data while preserving correctness will be essential.
These developing trends hint towards a future where enterprises prioritize high-quality data as a strategic asset required for informed decision-making and sustained success in an increasingly data-driven environment.📰
10. Conclusion
To put it succinctly, in order for organizations to succeed and make wise judgments, they must comprehend the importance of data quality. Inaccurate insights from low-quality data can have an impact on strategies and results. Organizations can improve overall performance, customer happiness, and operational efficiency by placing a high priority on data quality.🔖
It is imperative that readers do a rigorous assessment of their current data quality procedures. Conducting frequent audits, adopting data governance frameworks, and investing in tools for data cleansing and validation are key stages. To guarantee correctness, consistency, and dependability in data management, organizations should also set up explicit policies and procedures.
In today's data-driven world, organizations need to prioritize data quality if they want to remain competitive. Companies may realize the full value of their data assets and create the conditions for long-term growth and innovation by proactively addressing data quality issues and cultivating a culture that values correct information. Act now to improve your organization's data quality standards and provide it the tools it needs to prosper in the increasingly digital world.