1. Introduction to Data Science
In the broad subject of data science, knowledge and insights are extracted from both organized and unstructured data using scientific procedures, systems, algorithms, and methods. It requires mixing statistics, mathematics, computer science, domain experience, and communication abilities to identify patterns and make judgments based on data. In today's data-driven world, the capacity to collect, analyze, and interpret huge amounts of information has become critical for businesses and organizations to stay competitive.
Because data science offers useful insights from data analysis, it is essential to decision-making. Data scientists evaluate massive datasets using statistical methods, machine learning algorithms, and predictive modeling approaches to find patterns, anticipate results, and optimize strategies. These insights are crucial for decision-makers to make well-informed decisions that promote corporate expansion, boost operational effectiveness, enhance customer satisfaction, and reduce risks.
Essentially, data science equips businesses with the means to convert unprocessed data into insightful knowledge that drives strategic choices. Applications for it can be found in many different industries, including marketing, e-commerce, banking, healthcare, and cybersecurity. Businesses can obtain a competitive edge by using the predictive capacity of algorithms to precisely predict market trends or customer behavior by utilizing data science in their decision-making processes.
2. Data Collection and Processing
Important phases in applying data science to decision-making processes are data collection and processing. Depending on the sources and categories of information needed, different techniques are used to gather pertinent data. This can involve techniques like web scraping, interviews, surveys, and gathering data from sensors or Internet of Things devices. The process of gathering data can be made more efficient and accurate by utilizing a variety of tools and technologies, such as databases and APIs.
The next stage after data collection is to clean and preprocess raw data. This stage is crucial because raw data frequently has errors, missing values, inconsistencies, or outliers that might have a big impact on the analysis's conclusions. To get the dataset ready for analysis, data scientists employ methods include scaling numerical values, eliminating duplicates, standardizing formats, and imputation or deletion for missing values.
In order to guarantee the accuracy and dependability of the data used in decision-making processes, preprocessing and data cleaning are essential. Organizations can improve the accuracy of their analyses and make well-informed decisions based on dependable data sources by implementing best practices in these areas.
3. Data Analysis Techniques
Techniques for analyzing data are essential to decision-making in a variety of businesses. In order to comprehend the current situation, descriptive analytics summarizes historical data to provide insights into prior trends and performance. By using statistical algorithms and machine learning to predict future events based on past data patterns, predictive analytics helps businesses foresee possible outcomes and make well-informed decisions.
Prescriptive analytics goes one step further by suggesting courses of action that optimize results while taking into account a variety of constraints and goals, all based on predictive models. Organizations can improve their decision-making processes by utilizing these strategies to get insightful information from intricate databases. Businesses can use these analytical techniques to find hidden patterns, pinpoint performance-related factors, and model various decision scenarios in order to assess the possible effects prior to implementation.
Using these data analysis approaches gives decision-makers a thorough understanding of past, present, and future patterns, enabling them to make more confident strategic decisions. The quality and efficacy of decision-making at all organizational levels can be greatly increased by utilizing descriptive, predictive, and prescriptive analytics, whether the goal is supply chain operations optimization, marketing strategy improvement, or customer experience enhancement.
4. Applications of Data Science in Business Decisions
Decisions about business are shaped by data science in a variety of industries. Businesses use data analytics in the marketing domain to determine customer preferences, predict trends, and efficiently personalize campaigns. Businesses may precisely target particular audience segments with their marketing strategies by using tools like sentiment analysis and predictive modeling, which will increase conversion rates and boost return on investment.
Using data science to analyze competition insights, market demands, and customer feedback, product development processes can be streamlined significantly. Businesses may decide on product features, pricing policies, and innovation pipelines with knowledge by using tools like sentiment analysis for product reviews and machine learning algorithms for demand predictions. In addition to producing products that better satisfy consumer needs, this data-driven strategy shortens time-to-market and boosts competitiveness.
Data science improves operational efficiency by finding areas for improvement and streamlining corporate operations. Businesses can increase efficiency, reduce costs, and proactively manage risks by implementing algorithms for supply chain management, predictive maintenance, and resource allocation. Businesses may gain greater performance and agility in an increasingly dynamic marketplace by utilizing machine learning models and big data analytics in operations management. 😎
And, as I said above, the applications of data science in commercial decision-making are numerous and impactful. Organizations may remain competitive and flexible in the current fast-paced business environment by utilizing data-driven insights to improve marketing tactics, accelerate product development cycles, and optimize operational efficiencies. Through the utilization of sophisticated analytics tools and technologies, businesses can extract important insights from their data assets, promoting innovation, expansion, and success in every aspect of their operations.
5. Data-Driven Decision-Making in Healthcare
Healthcare decision-making is being revolutionized, and data science is essential to this. Within the field of customized medicine, data analytics allows medical professionals to use a plethora of patient data to customize therapies based on individual traits such as genetics, lifestyle, and surroundings. This method improves patient outcomes and overall care quality by enabling more accurate diagnosis and treatment options.
There are many advantages and challenges associated with incorporating data analytics into healthcare decision-making. Even though there is a wealth of data available, there are several obstacles to overcome in order to ensure data security, privacy, and accuracy. Overcoming reluctance to change and cultivating a culture that prioritizes evidence-based decision-making are necessary for integrating data-driven procedures into the current healthcare systems. Nonetheless, there are several advantages, such as better disease preventive tactics, improved clinical decision support systems, increased operational efficiencies, and ultimately better patient outcomes. There is much promise for a future in healthcare where treatments are more effective and individualized thanks to the possibility of data-driven decision-making.
6. Ethical Considerations in Data-Driven Decision Making
Ethical issues are crucial in data-driven decision-making because they guarantee that judgments are transparent, equitable, and accurate. It is imperative to tackle challenges like bias, privacy, and openness when utilizing data to guide decisions. Organizations can make more equitable decisions by being aware of potential biases in datasets and algorithms and taking proactive steps to mitigate them.
Another essential component of ethical data utilization is privacy issues. Sustaining stakeholder trust and adhering to legal obligations depend on making sure that sensitive data is appropriately safeguarded and used exclusively for intended reasons. Encouraging transparency at every stage of the decision-making process promotes accountability and criticism.
Guidelines and precepts are provided by ethical frameworks to prevent data misuse. In addition to facilitating the making of educated decisions, establishing explicit regulations for data collecting, storage, sharing, and analysis also safeguards the privacy and rights of persons. Following these concepts helps an organization develop a culture of accountability and integrity, which eventually results in more moral decision-making.
7. Case Studies: Real-World Applications of Data Science
Real-world data science applications highlight the significant influence this field has on decision-making procedures. Through the use of sophisticated recommendation systems that evaluate watching behavior to provide personalized suggestions, companies such as Netflix have changed the user experience. Netflix boosts subscription retention rates and improves user engagement by utilizing data science.
Data science-driven weather prediction algorithms have revolutionized forecasting accuracy and made it possible to send out timely alerts for severe weather events. Advanced data analytics is used by agencies like the National Weather Service to process large datasets and produce more accurate forecasts. By enhancing disaster preparedness plans and delivering early warnings, this use of data science in meteorology is vital to the protection of people and property.
These case studies demonstrate how companies and organizations may use data science to make well-informed decisions by using insights from intricate datasets. Businesses may improve consumer experiences, obtain a competitive edge, and spur innovation across a range of industries by utilizing data analytics. The success stories of organizations that have used data science highlight how important it is for determining strategic outcomes and efficiently maximizing performance indicators.
8. Future Trends in Applying Data Science for Decision-Making
Future trends indicate that developing technologies like AI and machine learning will play a major role in decision-making. Because these developments offer more precise insights for making wise judgments, they are reshaping the landscape. Because AI systems can quickly evaluate large amounts of data, organizations are better able to predict patterns and take preemptive measures. Strategic planning and risk management are improved by the prediction capabilities of more advanced machine learning models.
The development of data science tools and applications will have a significant impact on decision-making in the future. We can anticipate data science becoming become more specialized and available to a wider range of sectors as technology develops. It is probable that predictive analytics will become increasingly important in predicting results and refining tactics. Quick decision-making based on current information is made possible by real-time data processing, which promotes flexible and adaptable corporate procedures.
The ability to comprehend complex information will be enhanced by developments in data visualization tools, enabling decision-makers at all levels to properly interpret data. By automating the creation of insights through the integration of augmented analytics with business intelligence systems, businesses will be free to concentrate on making decisions rather than analyzing data. The trajectory of data science advances revolutionizing decision-making processes across several sectors appears positive for the future.
9. Education and Training for Data-Driven Decision-Making
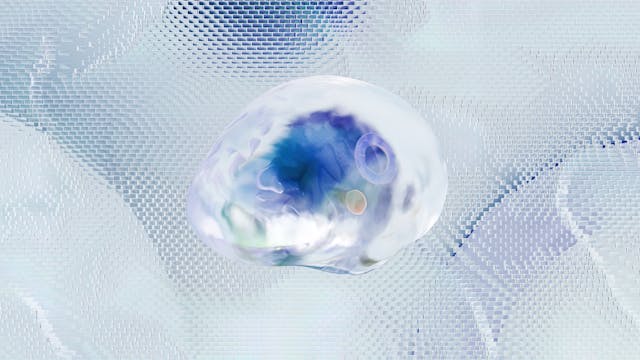
Learning statistics and programming languages like Python and R is essential when it comes to education and training for making decisions based on data. These abilities enable people to efficiently evaluate facts and extract significant insights for well-informed decision-making. Professionals can understand data effectively by knowing statistical concepts, and they can analyze and visualize complicated information efficiently by knowing computer languages.
There are several suggested courses and resources accessible for anyone looking to specialize in this field. Many different programming, statistics, and data science courses are available on websites such as Coursera, Udemy, and edX, which also offers courses for varying ability levels. To acquire practical skills in data analysis, specific courses like the University of Michigan's "Applied Data Science with Python" on Coursera or Jose Portilla's "Data Science and Machine Learning Bootcamp" on Udemy are highly recommended.
With the help of tools like Kaggle, you may work on actual data science projects and take part in contests to improve your problem-solving and practical skills. Learners can engage with peers and increase their level of knowledge in the field of data science by having access to a large repository of code libraries and projects through open-source communities such as GitHub. Through the utilization of these courses and tools, individuals can acquire the requisite competencies to flourish in professions involving data-driven decision-making in the education industry.
10. Implementing a Data Strategy for Effective Decision Making
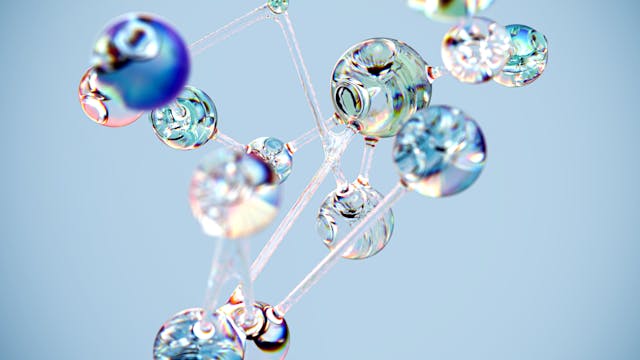
For a company to ensure successful decision-making, a data strategy must be implemented. There are various crucial steps involved in creating a strong data strategy. Firstly, organizations need to identify clear objectives and goals they seek to attain through data-driven decisions. This entails being aware of the precise issues or business possibilities that they hope to leverage through the application of data analytics.
Organizations also need to evaluate their existing data infrastructure and capabilities. It is crucial to identify the sources of current data, as well as their accessibility, quality, and the instruments required for gathering, storing, and analyzing it. This stage facilitates the comprehension of the gaps that must be filled in order to base judgments on accurate and trustworthy information.
Next, firms should focus on building systems for data governance and management. This entails setting up procedures for data sharing across organizational departments, guaranteeing data security and quality, and specifying roles and duties for managing data.
For employees to become more proficient with data literacy and analytics technologies, organizations must make investments in training programs and resources. This guarantees that decision-makers possess the know-how and abilities needed to accurately understand data insights and apply them to propel corporate objectives.
There are several solutions available when it comes to tools and software that make it easier to integrate data-driven decisions into corporate processes. Decision-makers can more quickly analyze complex datasets with the use of interactive dashboards and visualizations offered by business intelligence (BI) products like Tableau, Power BI, or Qlik.
Organizations can use machine learning platforms like Python with packages like Scikit-learn or TensorFlow for predictive modeling or clustering activities for more complex analytics needs. With the use of these tools, businesses may find patterns within big datasets and forecast future trends or results with precision.
Scalable infrastructure is provided by cloud-based services like Microsoft Azure, Google Cloud Platform (GCP), and Amazon Web Services (AWS) for effectively processing and storing massive amounts of data. The implementation of machine learning models and the automation of decision-making processes based on real-time insights are further integrated solutions offered by these platforms.
After putting everything above into perspective, we can say that putting a data strategy into practice entails coordinating organizational objectives with data resources through appropriate planning, governance, training, and utilizing the right tools and software to facilitate effective decision-making processes that lead to business success.
11. Performance Measurement through Data Analytics
An essential component of assessing the efficacy of decision-making models across a range of domains is performance measurement. Organizations can use data analytics to obtain insights into metrics and key performance indicators (KPIs) that are essential for evaluating the effectiveness of their decision-making procedures. By comprehending these metrics, companies may monitor their advancement, pinpoint opportunities for enhancement, and make well-informed selections in the future.
Using big data analytics has become crucial for improving performance evaluation techniques in today's data-driven world. Organizations are able to identify patterns, trends, and correlations that would otherwise be hard to find by analyzing enormous amounts of data in real-time. This helps them improve the overall efficacy and efficiency of their decision-making models by allowing them to fine-tune their performance indicators and modify their methods accordingly.
12. Collaborative Decision Making and Team Dynamics
In any business, collaborative decision-making is essential to successful teamwork. Collaboration solutions, such project management platforms or shared dashboards, let teams monitor progress, communicate more effectively, and come to data-driven choices more quickly. These technologies let team members coordinate their activities toward shared objectives by giving them instant access to pertinent data and insights.
Team dynamics can be greatly improved by incorporating interdisciplinary approaches that combine domain expertise with data insights. Teams that bring together the expertise of individuals with diverse backgrounds are better able to tackle problems from multiple angles and provide more all-encompassing solutions. Data science is essential to this process because it offers evidence-based insights that support varied teams' creativity and serve as a decision-making framework.
Applying data science to collaborative decision-making increases productivity while promoting team creativity and synergy. It gives people the ability to use their special talents while utilizing data to make decisions that are well-informed and promote organizational success.🥰