1. Introduction
Creating a strong big data strategy is essential for businesses trying to properly harness the power of information in today's data-driven environment. An organization's big data strategy describes how it will collect, store, process, and apply massive volumes of data to extract insightful knowledge. It entails making strategic judgments about what data is significant, how it will be used, and what tools and technologies are required to support these activities in addition to the technical aspects of data collection and management.
To guarantee the success of their big data strategy, businesses must take into account a few crucial concerns. These considerations center on setting specific goals, comprehending the data sources that are accessible, evaluating the technology needed, resolving issues with governance and security, choosing analytical techniques, and creating KPIs to track results. Early on in the planning phase, companies may develop a roadmap that synchronizes their big data projects with their overarching company goals and objectives by addressing five crucial concerns.
2. What is your business objective?
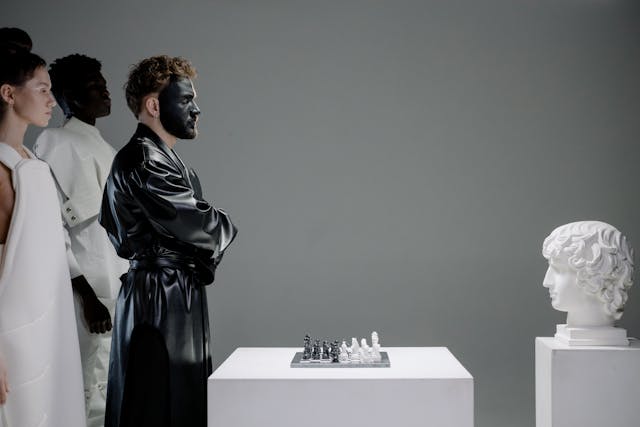
It's critical to have specific company objectives when creating a big data strategy. Establish clear objectives for using big data in your company first. Consider how big data may improve and assist your decision-making procedures, consumer experiences, and business operations. In order to optimize the results of your big data initiatives, be sure that these goals are quantifiable and consistent with your overall business plan. You can ensure that your big data activities are contributing significantly to the success of your organization and streamline them by explicitly defining your business objectives.
3. What type of data do you need to collect?
Choosing the right kind of data to gather is a critical first step in creating a big data strategy. This entails figuring out which data sources are pertinent to your company and knowing what kinds and formats are needed for analysis. Start by determining what precise data is required to achieve your strategic objectives. Think about internal data, such sales transaction data, customer information, or operations data. Important information can also be obtained from other sources including industry studies, social media feeds, and market trends.
The ability to distinguish between structured, unstructured, and semi-structured data is essential for creating a successful big data strategy. Quantitative information that neatly fits into predetermined categories, such as databases or spreadsheets, is referred to as structured data. Text documents, social media posts, videos, and photos that are more difficult to organize are all considered unstructured data. In between is semi-structured data, which has components like metadata or tags that provide some organization but still need to be processed in order to extract insights. Organizations may make sure they gather the necessary data to support educated decision-making and successfully accomplish their goals by outlining the kinds and forms of information required up front.
4. How will you collect and store the data?
One important thing to think about when creating a Big Data strategy is how you will effectively gather and store the data. It is crucial to evaluate different data collection techniques, such as batch processing or streaming. Real-time data processing and transport are part of streaming, which makes it perfect for applications that need quick insights. However, batch processing is more appropriate for less urgent analyses as it handles massive amounts of data at predetermined intervals.
Making the decision to use cloud solutions or store data on-premise servers is crucial. Although they provide complete control over data, on-premise servers can be expensive to scale and maintain. Pay-as-you-go cloud solutions offer cost-effectiveness, scalability, and flexibility. Determining the best strategy for efficiently gathering and storing Big Data will depend on how well you understand your organization's requirements.
5. Who will have access to the data?
The question of who will have access to the data must be taken into account when creating a big data strategy. It is critical to address big data security and privacy issues in order to safeguard sensitive data. By clearly defining responsibilities and rights for data access and handling, breaches and misuse can be minimized as only authorized workers can interact with the data. Organizations can preserve data integrity and regulatory compliance by setting access levels according to job duties and needs. Strong security measures that are put into place, like encryption, authentication procedures, and frequent audits, can help protect the data even more from cyberattacks and unwanted access.
6. How will you analyze the data?
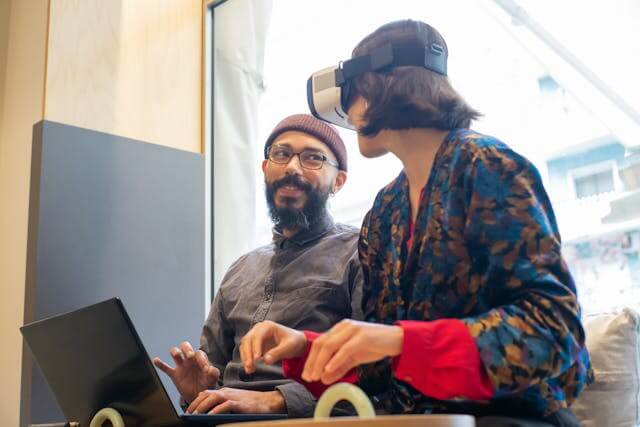
How you will examine the data is an important consideration to have when creating a big data strategy. This entails investigating different technologies and systems that facilitate the effective processing and analysis of large amounts of data. You may more effectively choose which of these tools—Hadoop, Spark, or Elasticsearch—align with the objectives and requirements of your company by having a deeper grasp of them.
It is imperative to concentrate on creating models and algorithms that are capable of extracting significant knowledge from the enormous volumes of data gathered. These models are essential for converting unprocessed data into useful information that may inform corporate strategy and decision-making. Some of the strategies used to extract meaningful patterns from large data sets are data mining techniques, predictive analytics, and machine learning algorithms.
You can make sure that your company makes the most of the information at its disposal by including good data analysis in your big data strategy. In a world where data is driving decisions more and more, this step not only helps with decision-making but also establishes the groundwork for innovation and expansion.
7. How will you ensure data quality?
Creating a big data strategy requires careful consideration of data quality. Putting policies in place to ensure data quality and integrity ought to be a primary concern. This entails setting up procedures for standardization, cleansing, and validation of data. To keep errors from influencing analysis and data-driven decision-making, data quality issues must be routinely monitored and addressed throughout time. Organizations can make sure that the insights derived from their big data projects are trustworthy and useful by putting strong quality control procedures in place.
8. How will you integrate big data into existing systems?
When designing a big data strategy, integrating it into current systems is vital. Evaluating the interoperability and compatibility of systems should be part of the planning phase for the integration with your existing IT infrastructure. To ensure smooth operations, think about how the new big data components will integrate with your current systems. The proper integration of these components necessitates a clear understanding of the data flow between them in order to enhance efficiency and avoid disruptions. Early on in the integration process, addressing any possible obstacles or bottlenecks will assist expedite the shift to a more reliable and data-driven infrastructure.
9. How will you measure the success of your big data strategy?
Key performance indicators (KPIs) that support your business objectives should be established before attempting to gauge the effectiveness of your big data approach. You may monitor your progress and determine whether your initiatives are producing the expected results with the aid of these KPIs. You can get important insights into the efficacy of your plan by routinely assessing how your big data activities are affecting business outcomes. Through the tracking of KPIs like return on investment (ROI), customer happiness, operational effectiveness, and other pertinent indicators, you can evaluate the accomplishment of your big data projects and make informed decisions to maximize performance.
10. What are the potential risks and challenges?
It is essential to take into account the possible dangers and difficulties that may arise while creating a big data strategy. A successful implementation depends on recognizing typical development process problems. These hazards could include things like inadequate cybersecurity protections, a lack of scalability, and problems with integration and poor data quality.
It is critical to mitigate cybersecurity threats when working with large data sets. It is essential to make sure that strong security procedures are in place to shield private data from breaches and illegal access. To prevent legal ramifications, compliance with legislation like the GDPR and other data protection laws must also be taken into consideration.
Another issue with big data solutions is scalability. Long-term success depends on making plans for future expansion and making sure the infrastructure can handle growing data volumes. Businesses can save money and avoid future restrictions or costly disruptions by foreseeing their scalability needs early on.
Organizations may create a strong big data strategy that protects against dangers and makes use of valuable insights by tackling these risks head-on and putting mitigation mechanisms in place.
11. How will you adapt and evolve your strategy over time?
A big data strategy must be modified and improved over time to be successful in the quickly dynamic corporate environment of today. Flexibility and scalability should be given top priority while creating a plan. Large quantities of complicated data with a variety of sources and structures are frequently involved in big data endeavors. You may more effectively adapt to changes in data sources, formats, and business requirements by including flexibility into your approach.
Maintaining the efficacy of your big data strategy and keeping it in line with your company's objectives requires constant improvement. This entails routinely assessing performance indicators, getting user input, and examining new trends in the sector. You can quickly rectify any holes or inefficiencies in your plan by remaining flexible and open to input.
Anticipating demands and obstacles in the future as your company expands is part of planning for evolution. This can entail growing your analytics capability to accommodate more intricate queries or scaling up your infrastructure to manage larger data volumes. You can steer clear of certain pitfalls and make sure your big data strategy is meaningful and relevant for the long term by proactively planning for scalability.
It takes a proactive approach that prioritizes flexibility, scalability, and continual optimization to adapt and evolve a big data strategy. Through constant adaptation to evolving business requirements and technical breakthroughs, enterprises may fully leverage their data assets and stimulate innovation throughout their whole operational spectrum.
12. Conclusion
For firms hoping to take use of data, creating a thorough big data strategy is essential. Businesses should lay a strong basis for their big data initiatives by defining clear objectives, taking scalability into account, assuring data quality, resolving security and privacy concerns, and promoting a data-driven culture.
Choosing the appropriate technology stack for the organization's needs, establishing strong governance procedures to guarantee data integrity and compliance, investing in ongoing learning and adaptation to stay ahead in the quickly changing big data landscape, and coordinating big data goals with business objectives are among the most important lessons learned from this process.
Effective use of big data has the power to revolutionize businesses by providing insightful information, stimulating creativity, optimizing decision-making procedures, boosting client experiences, and giving them a competitive edge in the modern digital economy. Businesses that use big data strategically will be more likely to prosper in a future where data is used more and more.