1. Introduction
Introduction: Data Mesh is a cutting-edge approach to data management that focuses on decentralizing data ownership and processing within an organization. Unlike traditional centralized data architectures, Data Mesh advocates for distributing data responsibilities across different business units or domains, empowering them to own and manage their data independently. This decentralized model aims to improve data accessibility, quality, and agility by breaking down silos and promoting a more collaborative and scalable environment for managing enterprise data.
Effective data management in today's data-driven world, when enterprises are flooded with massive amounts of data from multiple sources, may depend on taking Data Mesh into consideration. Organizations can overcome scalability, flexibility, and integration issues that frequently occur with centralized systems by implementing a Data Mesh framework. Businesses may democratize data access using Data Mesh while maintaining security and control, which eventually encourages creativity and well-informed decision-making throughout the company. In order to modernize your data infrastructure and realize the full potential of your data assets, it is crucial to determine whether Data Mesh is the perfect fit for your company.
2. Understanding Data Mesh
Gratitude Organizations trying to update their data architecture must use data mesh. A decentralized method for exchanging and managing data inside an organization is called data meshing. By treating data as a product and empowering domain-specific teams to own and manage the data they generate, it places a strong emphasis on dismantling data silos. This idea essentially moves the emphasis from dispersed accountability and autonomy to centralized control over data.
Data as a product, which treats data sets as reusable products with standardized interfaces, domain-driven decentralization, where cross-functional teams take ownership of data within their particular domains, self-serve infrastructure platforms that give teams the tools they need to manage their data efficiently, and federated governance, which guarantees compliance without centralizing control, are some of the fundamental tenets of Data Mesh.
By shifting away from monolithic, centrally managed systems and toward a more distributed and scalable paradigm, Data Mesh departs from conventional centralized data architectures. In traditional architectures, all of the organization's data collection, storing, and processing is usually handled by a single central team. On the other hand, Data Mesh is in favor of assigning these duties to specific teams that are located nearer the data source. This method improves organizational efficiency overall in managing vast and diverse data sets while enabling better agility, scalability, and creativity within each area.
3. Pros and Cons of Implementing Data Mesh
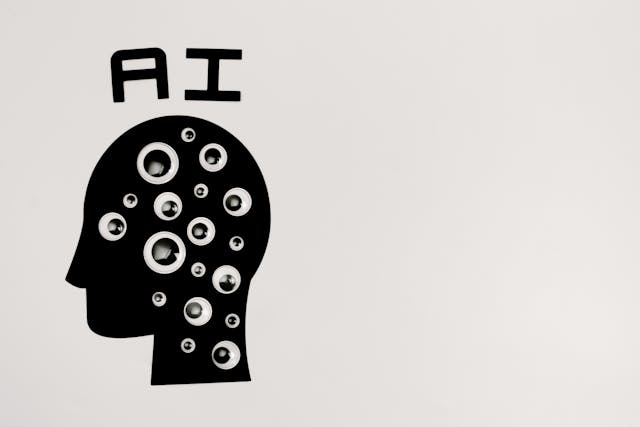
Adopting a Data Mesh approach has advantages and disadvantages of its own for your company. Let's start by discussing the advantages. Scalability is one of the main benefits; Data Mesh enables dispersed data ownership, which can aid in efficiently scaling up data processes. By allowing several teams to work freely on their own data domains without being restricted by a centralized data architecture, this strategy also adds flexibility to the table. By encouraging a culture where teams are more responsible for the accuracy and usefulness of their data, Data Mesh enhances data ownership.
On the other hand, one should not ignore the difficulties that come with putting data Mesh into practice. The intricacy it adds is one of the main obstacles. It can be difficult to maintain decentralized data architecture and make sure different domains communicate with each other smoothly. It could be necessary to hire new employees or upskill existing ones with experience in distributed systems architecture, data governance, and domain-driven design in order to implement a data mesh model. Organizational culture changes present another major obstacle; moving to a decentralized data approach frequently necessitates a change in perspective and working methods that may not be easy for everyone.
As previously stated, Data Mesh has many advantages, including scalability, flexibility, and better data ownership. However, before implementing Data Mesh, organizations need to carefully assess the challenges it presents, including complexity, skill requirements, and required cultural shifts. To decide if Data Mesh is the best option for advancing your data strategy effectively, it's critical to compare these benefits and drawbacks to your organization's unique requirements and capabilities.
4. Assessing Organizational Readiness for Data Mesh Adoption
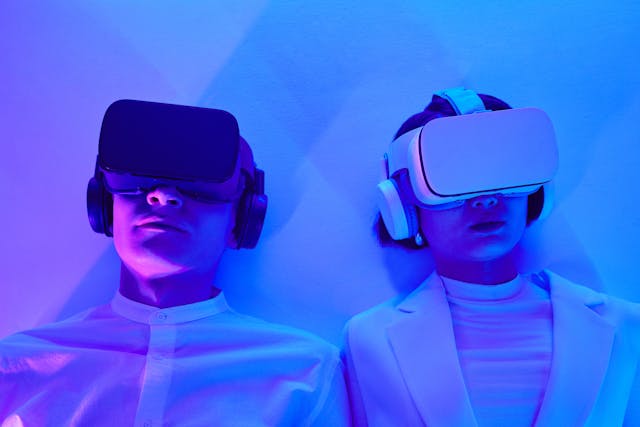
Prior to adopting Data Mesh, it is imperative to evaluate the organizational readiness for its implementation. A successful transition depends on taking into account a number of important elements. First and foremost, it's critical to assess the current infrastructure. This entails assessing integration capabilities, storage systems, and data pipelines to see if they adhere to the concepts of Data Mesh.
Second, it's critical to evaluate the culture of the company. In a Data Mesh structure, a fundamental change is toward decentralized data ownership and cross-functional collaboration. Assessing the culture's support for accountability, autonomy, and knowledge exchange will be helpful in determining how prepared people are for this new style of working.
Finally, it's important to examine the organization's resources. Making sure there are enough resources available to enable Data Mesh implementation—from trained staff to essential tools and technologies—is essential to the project's success. Organizations can assess their readiness for successful Data Mesh adoption by closely examining their infrastructure, culture, and available resources.
5. Setting Up a Data Mesh Framework
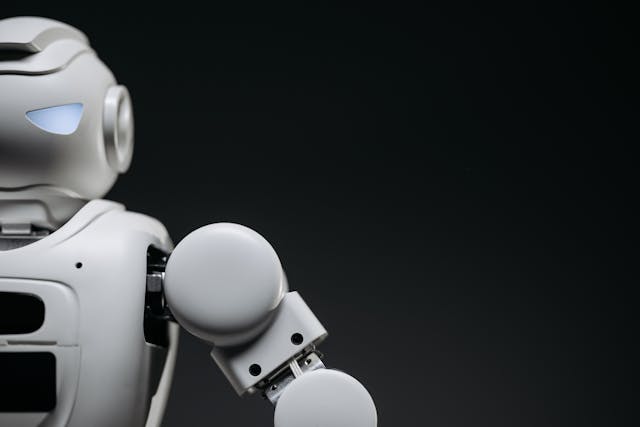
A Data Mesh structure must be set up using multiple crucial processes. First and foremost, it's critical to define domain boundaries. This entails recognizing the many departments within your company that produce or utilize data and drawing distinct divisions between them. This allows you to identify the source and consumption locations of data sets, facilitating more effective management and use.
Second, the effectiveness of a Data Mesh design depends on the definition of data products. In this step, the precise data sets or products that will be made available within each domain are identified, and their alignment with the requirements of data consumers is ensured. Organizations can enhance the quality, relevance, and accessibility of their data assets by developing clearly defined data products.
Last but not least, creating governance models is essential to preserving control and uniformity inside a Data Mesh architecture. Rules pertaining to data ownership, security, privacy, access rights, quality standards, and compliance requirements are helped to be established by governance frameworks. Organizations can guarantee that data is managed responsibly and ethically across all domains and data products by putting strong governance processes in place.💿
6. Case Studies: Successful Implementation of Data Mesh
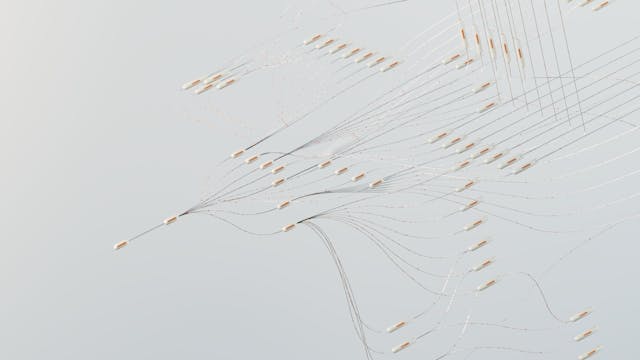
Case Studies: Successful Implementation of Data Mesh
Data Mesh has been effectively deployed by a number of enterprises to transform their data management procedures. Leading e-commerce platform is one example of a firm that experienced issues with data silos and restricted access for analytics teams. They decentralized data ownership by implementing Data Mesh, enabling distinct domains to independently maintain their data infrastructure. Improved data quality, quicker decision-making, and better cross-business unit communication were the outcomes for the organization.
An additional effective case study is provided by a multinational financial services company that used Data Mesh to eliminate data silos and improve processing agility. They enhanced data governance and expedited time-to-insights for crucial business decisions by shifting to a domain-oriented strategy. This change boosted operational effectiveness, decreased effort duplication, and gave teams more freedom to innovate utilizing data-driven insights.
These applications demonstrate the revolutionary potential of Data Mesh when carefully implemented within an enterprise. Simplified data pipelines, improved data dependability, and expanded scalability for changing business requirements are the results attained. The case studies provide valuable insights into the significance of cross-functional collaboration, corporate buy-in, and continuous domain boundary refinement for the sustained success of Data Mesh adoption.
7. Pitfalls to Avoid When Transitioning to a Data Mesh Model
Organizations may run into common implementation difficulties while switching to a Data Mesh paradigm. One such error is to underestimate the necessary cultural change. It's critical to guarantee team buy-in by thoroughly outlining the advantages of this new strategy. The efficacy of the Data Mesh can be hampered by neglecting data governance, which can result in inconsistent and diverse data sources.
Organizations can use risk-mitigation and seamless transition techniques to help them overcome these obstacles. First off, putting money into comprehensive training programs that teach staff members the fundamentals of Data Mesh can promote acceptance and comprehension at all organizational levels. Second, during the transition process, alignment with business objectives is ensured by defining precise measurements and KPIs to monitor progress and assess performance.
Encouraging cross-functional teams to collaborate and share expertise is essential to the implementation process's success. Promoting open lines of communication and setting up comment sections can help to resolve issues quickly and promote ongoing development. Organizations can effectively move to a Data Mesh architecture for enhanced data management and analytics capabilities by giving priority to these techniques and avoiding frequent pitfalls.
8. The Future of Data Management with Data Mesh
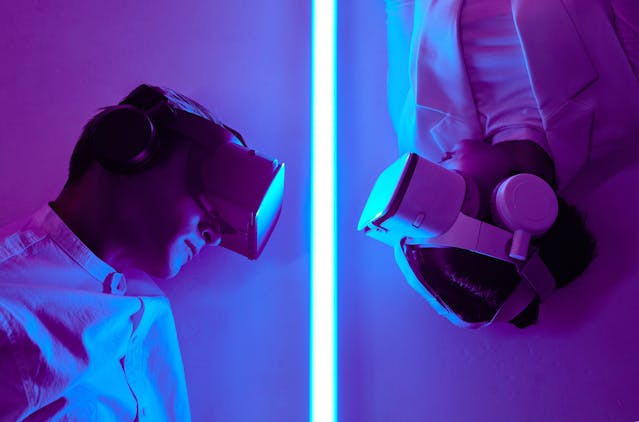
Data management is set to undergo substantial transformation in the future as long as enterprises continue to embrace Data Mesh. Forecasts indicate that a growing number of businesses will embrace Data Mesh, a decentralized method of handling data, as it becomes more commonplace across industries. This change is probably going to spark a major revolution in the way that data is gathered, stored, and used in businesses.
We may expect Data Mesh to have a significant impact on AI/ML capabilities, data analytics, and overall business performance in the upcoming years. Data Mesh can improve the caliber and scope of insights obtained from data analytics by dismantling data silos and facilitating cross-functional teams' access to and use of data. Organizations are now able to make better decisions by using a more thorough grasp of their data landscape because to the democratization of data.
Organizations' use of AI/ML capabilities is predicted to transform with the implementation of Data Mesh. Data Mesh helps hasten the creation and implementation of machine learning models by offering a unified view of data assets and encouraging cooperation between domain experts and data practitioners. Because of this expedited procedure, AI efforts are guaranteed to be based on solid foundations of diversified, high-quality datasets, which produces forecasts that are more accurate and insights that can be put to use.
By utilizing organizational data to its fullest, Data Mesh's widespread implementation will boost overall corporate performance. Businesses may uncover new growth opportunities, optimize operations, and react quickly to market dynamics with improved access to relevant and dependable data sources. In an increasingly data-driven world, Data Mesh's agility helps businesses stay ahead of the competition and quickly adjust to changing business situations.
9. Training and Upskilling for Data Mesh Adoption
Adopting a Data Mesh structure inside an organization requires training and upskilling. Since technology is advancing so quickly, it is crucial to make sure that staff members are knowledgeable on the latest tools and techniques. By funding training initiatives, employers can provide current staff members the know-how to successfully negotiate the intricacies of a data mesh architecture.
Various measures can be implemented by organizations to facilitate the process of upskilling. Offering thorough training programs that address important ideas like domain-driven design, decentralized data ownership, and data governance is one strategy. These programs can be offered both internally and outside through collaborations with specialized training suppliers. Encouragement of staff to obtain certifications in pertinent domains can improve their knowledge and preparedness for putting Data Mesh solutions into practice.
When new talent with a solid understanding of Data Mesh principles is needed, hiring may be necessary. Candidates with expertise in data engineering, distributed systems, and industry-specific domain knowledge should be sought after by recruiters. Putting together a team with a variety of skill sets and a common goal for Data Mesh implementation can greatly improve adoption efforts.🤩
Giving training and upskilling programs top priority shows that a firm is committed to embracing innovation and developing a data-driven culture. Companies can position themselves for long-term success in utilizing Data Mesh to unlock the full potential of their data assets by investing in chances for people to learn and grow. 📣
10. Building Business Cases for Adopting a Data Mesh Approach
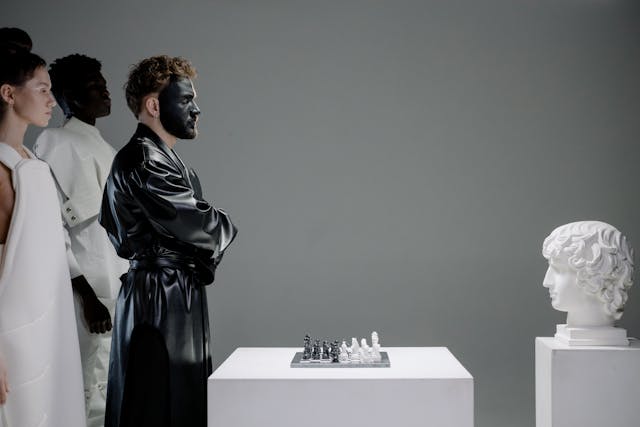
Developing a compelling business case is essential if your company is thinking about implementing a Data Mesh method. This entails developing frameworks for cost-benefit analyses to support the expenditures necessary to switch to a data mesh paradigm. Through the articulation of the prospective ROI and enduring advantages, stakeholders can gain a deeper comprehension of the value proposition associated with this transition.
Using case studies or pilot projects is a useful technique to illustrate the possible return on investment (ROI) of putting a data mesh into place. These efforts have the potential to provide observable outcomes, like better data quality, faster insight production, and higher team cooperation. Organizations may demonstrate the beneficial effects of a data mesh strategy on their operations and decision-making processes quantitatively by comparing key performance indicators (KPIs) before and after implementation.
Your business case will benefit from having success stories and real-world examples to help unify the organization's different stakeholders. These case studies provide as useful examples of how, in today's data-driven environment, a data mesh framework may foster creativity, adaptability, and competitiveness. Gaining support and funding for this revolutionary endeavor requires proving the viability and efficiency of a data mesh through measurable outcomes.