1. Introduction
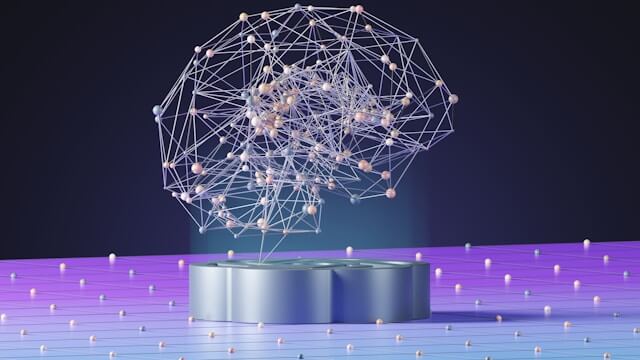
**Introduction:**
Two phrases that frequently rule conversations in the field of data are "small data" and "big data." Small Data describes datasets that are easier to handle, usually with sizes between a few megabytes and terabytes. Conversely, Big Data refers to extremely large amounts of data—often more than terabytes or even petabytes—that call for sophisticated methods and instruments for processing and analysis.
It's critical to comprehend the differences between little and big data because they affect the methods and resources used by companies to glean insights from their data. Because of its manageable size, Big Data offers a broader perspective by evaluating large-scale trends and patterns that could otherwise go missed with smaller datasets, whereas Small Data delivers more concentrated, granular insights. Both kinds of data are crucial to decision-making processes in a variety of businesses, which emphasizes the need for a comprehensive strategy that takes into account the advantages and disadvantages of each type.
2. The Basics of Small Data
The term "small data" describes datasets that are manageable by one person or small group of people without the need for sophisticated equipment or technology. Small data is characterized by its high quality, relevance, simplicity, and interpretability. Personal diaries, customer feedback forms, attendance sheets, and spreadsheets that track spending are a few examples of little data that are used in daily life.
The price and accessibility of tiny data analysis is one of its benefits. Little datasets don't require a lot of resources to yield insightful conclusions and useful data. Small data analysis's drawbacks, however, are its possible lack of representativeness and incapacity to identify intricate patterns found in larger datasets. Due to small sample numbers, biases may also affect little data.
Comprehending the fundamentals of little data is essential for individuals and enterprises seeking to effectively extract significant insights from small datasets. Small data analysis has its own set of features, applications, benefits, and drawbacks that must be understood in order to decide how best to use this kind of data in different situations.
3. Exploring Big Data
Big data is the term used to describe the massive amounts of both structured and unstructured data that constantly overwhelm a company. It may be impossible for conventional data processing technologies to effectively handle this deluge of information. The three Vs—volume, velocity, and variety—are among its essential characteristics. The terms volume, velocity, and variety all relate to the sheer amount of data generated, the rate at which new data is created and needs to be processed, and the various sorts of data sources, including texts, photos, and videos.
The significance of big data is eloquently illustrated by real-world situations. Social networking sites such as Facebook, for example, produce enormous volumes of user-generated material every day in the form of posts, likes, comments, and shares. Massive transactional data is gathered by e-commerce behemoths such as Amazon from millions of global customers' purchases. These illustrations demonstrate the enormous scope of big data's application in today's digital environment.🙃
Big data analytics' introduction has presented enterprises with both benefits and challenges. One difficulty is efficiently managing the vast amount and diversity of data in order to derive insightful knowledge. There are many obstacles in ensuring data security and quality. Big data analytics, on the other hand, gives businesses the chance to better analyze consumer behavior patterns, personalize content, increase operational effectiveness through predictive analytics, and make more informed strategic decisions. Using big data analytics can provide you a substantial competitive edge in the information-driven economy of today.
4. Small Data vs. Big Data: Contrasts in Size and Scope
The main differences between big and little data are in terms of volume, pace, diversity, and authenticity. While large data refers to enormous pools that require specialized tools for storage and analysis due to their scale exceeding ordinary processing capabilities, small data refers to manageable volumes commonly maintained in spreadsheets or basic databases. Compared to the quick and real-time nature of big data, the velocity of tiny data is slower and more predictable.
Since tiny data frequently consists of organized information like text or numbers, its variety is constrained. On the other hand, big data includes a wide range of formats, including photos, videos, posts on social media, sensor readings, and more. In both cases, authenticity is essential; however, because big data comes from so many different and large sources, it might be harder to guarantee accuracy and dependability.
Traditional statistical approaches such as regression analysis, along with basic visualization techniques, are the main emphasis of methods for evaluating tiny data. However, in order to effectively extract insights from the enormous datasets, handling big data necessitates the use of advanced analytics techniques like machine learning algorithms, artificial intelligence models like neural networks, and distributed computing frameworks like Hadoop or Spark. The transition to big data analytics requires proficiency with sophisticated tools and programming languages like as Python or R in order to analyze and comprehend large-scale datasets efficiently.
5. Applications and Use Cases
When it comes to use cases and applications, tiny data shines in niche markets like local enterprises and targeted marketing. Since these contexts usually entail smaller data needs that can be managed and evaluated efficiently without the need for complex tools or systems, small data sets are generally sufficient for them. Knowing the unique interests and habits of each customer on a smaller scale might help personalized marketers create more focused and successful advertising efforts. In a similar vein, local companies can use tiny data to customize their products to the unique requirements of their neighborhood.
However, large-scale research projects and predictive analytics are two areas where big data excels. The power of big data resides in its capacity to analyze enormous volumes of data from various sources in order to find hidden correlations, patterns, and insights that would be hard to find with smaller data sets. For instance, predictive analytics forecasts future patterns and behaviors with a high degree of accuracy by evaluating vast amounts of historical and real-time data. By using the power of vast datasets, big data helps researchers tackle challenging challenges in large-scale research initiatives across multiple fields, like social sciences, healthcare, and climate science.
Big data and little data both have unique benefits and can be useful depending on the situation in which they are used. Comprehending their advantages enables firms to make the most of the appropriate kind of data for their particular requirements, be they customized marketing plans for a neighborhood company or ground-breaking study projects with worldwide ramifications.
6. Delving into Practical Scenarios
Small data might provide significant advantages in the field of data analytics that huge data may not always provide. For example, using tiny data from customer feedback cards and in-store observations to inform focused marketing efforts resulted in a large increase in sales in a case study of a boutique apparel business. Big data, however, is relevant to larger businesses, such as the e-commerce behemoths. Personalized suggestions have been improved by Amazon's use of big data to examine consumer purchasing trends and behaviors, which has increased customer happiness and sales volume.
The success story of a nearby coffee shop that customized its menu offers by using basic sales records and client preferences demonstrates the efficacy of utilizing tiny data. Higher profit margins and more client loyalty were the outcomes of this customisation. On the other hand, Netflix is a prime example of how effectively big data insights can be used; it leverages viewership data to offer tailored content recommendations depending on user activity. This degree of accuracy has proven crucial in keeping users interested and influencing how they watch.
These real-world examples show the critical roles that big and tiny data play in many situations. Businesses can concentrate on unique insights and use limited resources to make targeted decisions thanks to small data. Big data, on the other hand, provides businesses with enormous amounts of information that enable them to recognize larger trends, forecast shifts in the market, and greatly enhance their operations. Organizations can more successfully drive growth and innovation by utilizing little and large data by knowing the distinct advantages of each method.
7. Balancing Act: Leveraging Both Small Data and Big Data
Finding the right mix between small and big data is essential to gaining insightful knowledge in today's data-driven environment. Small data delivers more specialized and qualitative details, but big data supplies enormous volumes of information for analysis. By merging the advantages of both strategies, companies can improve their decision-making procedures and get more profound understanding.
In a hybrid approach to data analytics, the power of big data's scale and patterns is combined with small data's specific insights and precision. To fully reap the benefits of any type of data, it is imperative to know when to use it. While big data is best at finding patterns and correlations across massive datasets, little data can be particularly helpful for understanding the preferences or behaviors of specific customers.
It is critical to match your strategy with your unique objectives and available resources in order to determine the best combination of little and big data for your company. For example, you might get better results by concentrating on little data if your objective is to personalize consumer experiences. However, using big data analytics might be more successful if your goal is to foresee market trends or increase operational efficiency.🤓
Businesses may boost innovation, make better decisions, and maintain their competitiveness in a market where consumers are becoming more and more data-savvy by implementing a balanced strategy that takes into account the advantages of both little and big data.
8. Tools and Technologies for Small Data Analysis
There are a number of technologies and tools designed specifically to handle small datasets and analyze them efficiently. These tools give customers the skills they require without the complexity or overhead associated with big data solutions, and they are specifically designed to meet the demands of working with smaller amounts of data.
Microsoft Excel is a widely used spreadsheet application that provides robust data analysis functionalities appropriate for modest datasets. Excel's pivot tables, charts, and other formulas make it possible for users to work with and evaluate small datasets effectively. Because of its intuitive interface, even those without considerable technical knowledge can utilize it.
Google Sheets is another tool that is worth noting. It is a cloud-based spreadsheet solution that allows for real-time updates and collaboration on modest datasets. Comparable functionality to Excel are provided by Google Sheets, which also has the benefit of being accessible from any location with an internet connection. It is especially helpful for groups who collaborate on tiny datasets.
Interactive data visualization is possible using tools like Tableau Public for more in-depth exploration of small datasets. With Tableau Public, users can immediately find patterns and insights by creating dynamic visualizations of their tiny datasets. Without requiring any coding knowledge, its drag-and-drop interface makes the process of building visualizations easier.
Programming languages like Python and R provide strong libraries for efficiently managing tiny datasets in addition to these tools. For smaller-scale tasks, packages like R's dplyr and Python's pandas offer functions for data cleaning, manipulation, and analysis. For users who want to run more complex studies on their tiny datasets, these languages provide flexibility and scalability.💽
The size of the dataset, its complexity, the user's level of experience, and the particular needs of the project at hand all play a role in selecting the best tool for small data analysis. Analysts may easily gain significant insights from their data and optimize workflows by utilizing specific tools and technologies that are optimized for managing smaller datasets.
9. Harnessing Big Data Analytics Tools
Numerous widely used technologies allow for the processing of enormous amounts of data in the field of Big Data analytics. One such piece of technology is Apache Hadoop, an open-source framework that makes use of straightforward programming concepts to enable the distributed processing of massive data sets across computer clusters. Hadoop is a mainstay of the Big Data ecosystem because of its speedy processing and massive data storage capabilities.
A significant participant in this field is Apache Spark, a quick and all-purpose cluster computing platform that offers high-level APIs in R, Python, Scala, and Java. Spark is ideally suited for iterative algorithms required in machine learning and interactive data analysis because of its in-memory computing capabilities.
Real-time streaming data processing has also been made possible by technologies such as Apache Flink and Apache Kafka. Kafka serves as a distributed streaming platform built to handle large volumes of data with fault tolerance, whereas Flink offers effective stream processing capabilities with low latency and high throughput.
These tools, which offer scalable methods for effectively processing and analyzing big information, have completely changed the way businesses handle their data. Businesses may gain useful insights from their Big Data reserves at a never-before-seen pace by utilizing these technologies, which allows them to implement data-driven decision-making strategies that will help them win in the current competitive environment.
10. Ethical Considerations in Data Analysis
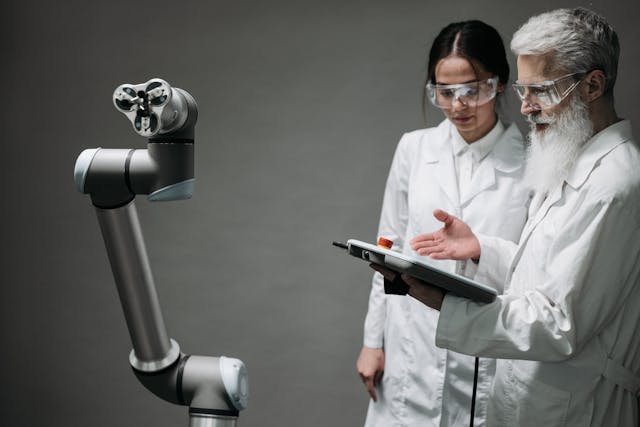
In the realm of data analysis, privacy concerns are paramount whether dealing with small-scale personal information or large-scale aggregated datasets.
The danger with limited data is that people could be identified by seemingly insignificant details. The likelihood of privacy breaches rises with the collection and analysis of more personal data. De-anonymization can occasionally result from simple actions as merging a few ostensibly anonymised data points.😬
However, big data comes with its own set of difficulties. Large datasets may mask individual identities, but the sheer bulk and interconnectedness of big data make it more likely that they may be misused or accidentally disclosed. When aggregated data is merged with outside sources or cross-referenced in unexpected ways, it may unintentionally reveal private information.
Therefore, protecting people's right to privacy must come first in ethical concerns for data analysis, regardless of scale. In today's data-driven world, preserving ethical standards and preserving trust require finding a balance between using data to get insights and safeguarding personal information.
11. Future Trends in Small Data vs. Big Data
Future developments in small-versus big-data trends have the potential to completely change the data analytics field. We may anticipate a move toward more complex data processing and analysis techniques as a result of technological breakthroughs like automation, machine learning, and artificial intelligence. The growing use of AI-driven technologies in small- and big-data analytics is one significant trend that allows for more effective data processing, interpretation, and collecting.
The emergence of edge computing, which involves processing data closer to the point of generation instead of depending entirely on centralized servers or cloud services, is another important trend that is about to happen. This method lowers latency and improves the ability of organizations to make decisions in real time, regardless of the size of their datasets. It is anticipated that blockchain technology will be essential in guaranteeing data security, integrity, and transparency in a society where protecting personal data is critical.
The Internet of Things (IoT) will generate enormous amounts of heterogeneous data as it spreads throughout businesses, necessitating the development of creative storage, management, and analysis solutions. The integration of IoT with big data analytics presents novel prospects for enterprises to extract significant insights from networked devices and sensors. Organizations can get a competitive advantage through improved operational efficiency and customer-centric initiatives by utilizing these technologies in concert.
Furthermore, as previously mentioned, the direction of future trends in little versus big data is towards a more intelligent and linked approach to data analytics. Businesses who adopt these innovations will be able to make more informed decisions more quickly, find hidden patterns in their datasets, and seize new chances for expansion and innovation in a world going more and more digital.
12. Conclusion: Embracing a Balanced Approach
Finding a balance between big data approaches and small data principles in the field of data analysis is essential to gaining insightful knowledge. Organizations may leverage the greatest features of both worlds by fusing the scale and speed of big data with the accuracy and depth of small data.
Small data provides a narrowed-down, detailed perspective that aids in understanding subtleties and information that large data may miss. Conversely, big data offers wide-ranging patterns and trends that can uncover comprehensive understandings and predictive analytics. Combining these methods enables a thorough comprehension of intricate events.
In order to successfully align small data principles with big data approaches, enterprises need to put context before quantity. Knowing the context in which each kind of data functions guarantees that the insights are applicable and useful. Building confidence with stakeholders requires upholding transparency in data gathering procedures and guaranteeing the ethical use of information.
Organizations may make educated decisions by adopting a balanced approach that utilizes both big and small data, providing a comprehensive picture of their data landscape. In today's data-driven environment, businesses may drive innovation, enhance operations, and eventually achieve sustainable success by identifying the benefits of each strategy and intelligently combining them.