1. Introduction to Data Mesh
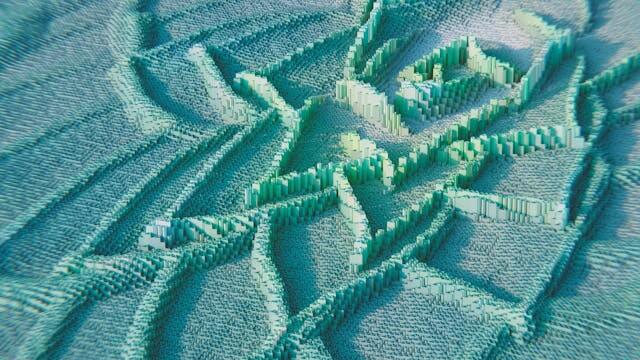
Data Mesh represents a paradigm shift in the use and management of data within businesses. Data Mesh is a term that ThoughtWorks' Zhamak Dehghani used to describe a decentralized method of data architecture. Data Mesh calls for dismantling these data silos into smaller, more manageable areas, in contrast to traditional centralized data systems where data is segregated and controlled by specialist teams.
The practice of centralizing all data into a single, enormous repository in traditional data architectures frequently results in bottlenecks, inefficiencies, and scalability issues. These centralized systems find it difficult to handle the increasing volume, diversity, and velocity of information that enterprises are collecting. Because it mostly relies on monolithic solutions that take a lot of work to grow and maintain, this rigid structure inhibits innovation and agility.
Data Mesh, on the other hand, supports decentralization by giving domain-specific teams inside an organization ownership over data. Every team is in charge of managing the data in their area from beginning to end, from gathering and processing to storing and analyzing it. In addition to encouraging ownership and autonomy, this strategy frees teams from the limitations of a centralized, one-size-fits-all system to customize solutions that best meet their unique requirements.
2. Key Principles of Data Mesh
The idea of Data Mesh has become popular as a viable substitute for conventional centralized methods in the field of data management. Two fundamental ideas of this paradigm shift are a domain-driven approach and decentralization. Decentralization is dividing large, cohesive data systems into more manageable, standalone components that are in line with particular business areas. This architectural change promotes agility and autonomy in decision-making processes by enabling domain teams to take ownership of their data.😽
The marketing of independent, self-serve data goods is another essential Data Mesh tenet. Teams no longer require the assistance of centralized data engineering teams to independently access and utilize the data they require thanks to the encapsulation of domain-specific logic and capabilities within these solutions. This methodology not only expedites the time-to-insight but also fosters grassroots invention and experimentation.
To put these ideas into practice, organizations must adopt a new perspective that prioritizes democratizing data access and empowering domain experts. By using Data Mesh, businesses can foster a culture of creativity and cooperation among cross-functional teams and increase the flexibility, scalability, and resilience of their data management strategies.
3. Benefits of Adopting a Data Mesh Architecture
Adopting a Data Mesh architecture has several advantages for contemporary businesses. Scalability and agility in data processing are two major benefits. Organizations can efficiently scale their systems to meet increasing needs without running into bottlenecks by decentralizing data ownership and processing. This adaptability makes it possible to allocate resources more effectively, streamline processes, and respond more quickly to shifting business requirements.
Enhancing data governance and quality can be achieved by implementing a Data Mesh structure. Clear ownership of data domains that are divided across different teams or domains increases accountability and responsibility for the accuracy and integrity of data. By encouraging improved data governance procedures, this decentralized strategy makes sure that data is maintained accurate, standardized, and compliant with laws.
In summary, implementing a Data Mesh design has the potential to completely transform the way businesses manage their data procedures. Businesses can unlock new levels of efficiency and effectiveness in using their most precious asset—data—by enabling scalability and agility in data processing while improving data quality and governance processes.
4. Challenges and Considerations When Implementing Data Mesh
Organizations must carefully negotiate the challenges and issues associated with implementing a Data Mesh methodology. Coordinating the organizational change needed to move toward decentralized data ownership is a major obstacle. In order to implement this paradigm change, previous centralized data governance models must give way to a distributed approach in which domain-specific teams are responsible for the organization's data domains. Promoting this new way of thinking among staff members and making sure that teams are in agreement can be difficult but essential to a successful Data Mesh implementation.
In terms of technology, some technological prerequisites must be met in order to develop a Data Mesh architecture that can efficiently handle decentralized data activities. This entails implementing a strong data infrastructure that can support the decentralized nature of a data mesh, such as scalable storage systems, frameworks for data processing, and tools for managing metadata. In order to provide smooth collaboration, data discovery, quality assurance, and governance within a decentralized data environment, organizations need to assess their current tech stack, pinpoint any potential gaps in their technological capabilities, and either build new tools or invest in existing ones. Overcoming technological obstacles in the implementation of a Data Mesh strategy requires striking the correct balance between providing autonomous data capabilities to domain teams and upholding overall control and standardization.
5. Case Studies: Successful Implementations of Data Mesh
A number of businesses have revolutionized their data architecture strategies by successfully implementing Data Mesh. For example, a well-known e-commerce platform decentralized its data architecture using Data Mesh. The organization increased its scalability and agility in reacting to constantly changing market needs by dismantling organizational silos and enabling individual teams to handle their data domains efficiently.
In a another instance, a global financial institution used Data Mesh to make data integration procedures more efficient. This strategy ensured regulatory compliance while enabling the firm to reconcile different data sources across divisions. Consequently, the organization attained improved data quality and expedited decision-making skills.
Adopting Data Mesh concepts improved the product development lifecycle of a tech business. The organization accelerated innovation cycles and promoted cross-functional team collaboration through the use of domain-oriented data ownership and governance standards. This strategy enhanced the overall quality of the product while enabling the company to quickly respond to consumer input and industry developments.
These real-world examples show how democratizing data access, encouraging cross-team collaboration, and improving operational efficiency can all lead to significant business outcomes with Data Mesh. In the upcoming years, it is anticipated that Data Mesh usage across a range of industries will pick up steam as more businesses realize how important scalable and decentralized data infrastructures are.
6. Evaluating if Your Organization Should Embrace Data Mesh
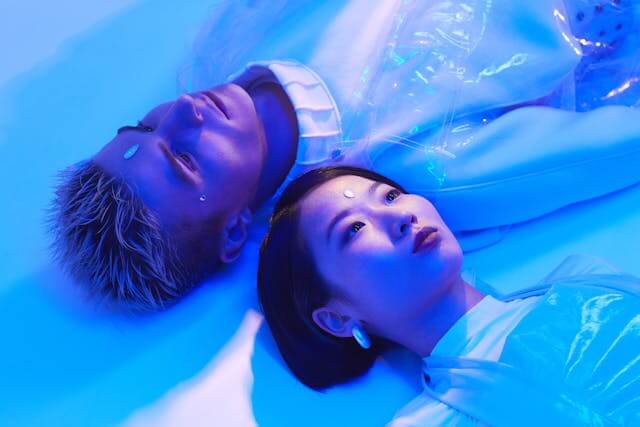
Consideration should be given to a number of criteria when determining if your firm should implement a Data Mesh architecture. Start by evaluating the condition of your data stack and infrastructure. Assess whether the data environment in your company is decentralized and complex, rendering centralized approaches less successful.
Second, think about the culture and structure of your company. A move toward decentralized, domain-oriented teams with distinct data product ownership is necessary for Data Mesh. Evaluate if your firm is prepared for this change in thinking and has the requisite skills and leadership to support it.
Examine your data platform's requirements for scalability. Data Mesh's distributed methodology provides scalability by design. Making the switch to a Data Mesh architecture could put your company in a better position for future scalability requirements if you foresee substantial increase in the amount and complexity of data.
Evaluate the data governance procedures that are currently in place in your company. Strong governance frameworks are essential to ensuring that data quality, security, compliance, and privacy are upheld across the mesh as a result of Data Mesh decentralizing data ownership. Examine whether your current governance procedures make sense in light of this new paradigm.
Finally, assess how prepared your current teams are to implement a Data Mesh architecture. Employee upskilling and training may be required to give them the abilities they need to function well in a domain-oriented organization.
As I mentioned before, carefully consider aspects like organizational preparedness for cultural changes, governance capacities, scalability needs, and current infrastructure maturity before meshing using Data Mesh.
and team readiness for skill adoption to determine if transitioning to a Data Mesh architecture aligns with your organization's strategic goals and operational capabilities.
7. Steps to Start Implementing a Data Mesh Strategy
Implementing a Data Mesh strategy requires careful planning and execution to ensure success. Here are seven key steps to get started:
1. **Evaluate Your Present Situation**: Start by performing a comprehensive evaluation of the team competencies, governance policies, and data infrastructure that are currently in place inside your company. This will direct the creation of your strategy and assist you in identifying areas that require improvement.
2. **Define Business Objectives**: Clearly state the goals you have for your company's use of a data mesh approach. Knowing your objectives will help your organization's decentralization and democratization of data.
3. **Create Cross-Functional Teams**: Assemble domain experts, data scientists, data engineers, and other pertinent stakeholders into cross-functional teams. Within the Data Mesh framework, these teams will work together to design and implement domain-specific data products.
4. **Develop Data Products**: Assist domain teams in creating customized data products that satisfy their own requirements, all the while following established standards for data discovery, sharing, and consumption. These products ought to be easy to integrate with other domains and self-serve.
5. **Implement Data Governance**: Build strong frameworks for data governance that guarantee privacy, security, compliance, and quality of data for all domain-specific data products. Sustaining system trust requires unambiguous policies about metadata management, access limits, and data ownership.
6. **Adopt Modern Technologies**: To meet the scalability, reliability, and performance requirements of your Data Mesh implementation, make use of modern technologies like cloud infrastructure, containerization, microservices architecture, and AI/ML tools.🗜
7. **Iterate and Improve**: Keep an eye on your Data Mesh implementation's performance in relation to predetermined KPIs and user input. Iterate the design in response to practical usage observations to foster innovation and ongoing improvement throughout your data ecosystem.
By carefully following these steps and customizing them to fit the particular requirements and obstacles faced by your company, you can create a strong basis for a smooth transition to a Data Mesh design that will enable your teams to efficiently utilize your data assets to the fullest.
8. Tools and Technologies for Building a Data Mesh Architecture
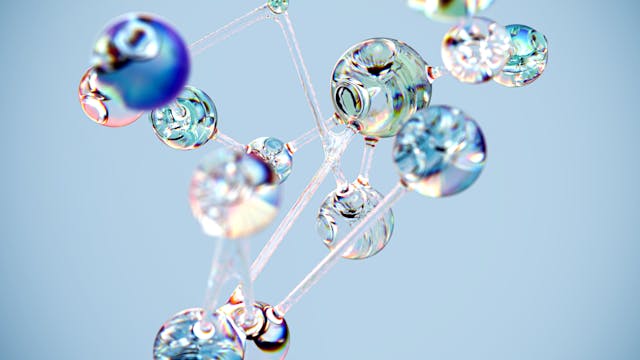
A number of new technologies and tools are being developed to aid in the deployment of a data mesh architecture. The following important software programs can assist businesses in creating and maintaining their data mesh:
1. **Apache Kafka**: This distributed streaming platform can act as the brains of your Data Mesh design thanks to its high-throughput, low-latency messaging mechanism. It enables smooth data integration between different systems and services.
2. **Apache Flink**: Flink is a strong stream processing framework that can manage enormous volumes of data quickly. It can be applied to real-time processing in a data mesh to facilitate prompt decision-making and insights.
3. **Apache Hadoop**: Although it's not a brand-new technology, a Data Mesh can nevertheless make use of Hadoop's ecosystem of tools, including HDFS, MapReduce, and Hive, to store and analyze massive amounts of data in a distributed fashion.
4. **Presto**: A distributed SQL query engine that is available for free, Presto can assist in bringing data access inside a Data Mesh together. It enables users to query data regardless of where it is stored, in more modern big data systems like Cassandra or Hadoop or in conventional databases.
5. **Apache Airflow**: Airflow is a workflow authoring, scheduling, and monitoring tool that uses programming. It can be applied to coordinate data pipelines in a data mesh, guaranteeing seamless data transfer between various domains.
6. **Databricks**: Databricks is a prominent big data processing platform that offers a unified analytics built on top of Apache Spark. It provides features for collaborative workspaces that help teams interact with data in a decentralized way, which is necessary according to the Data Mesh paradigm.
7. **Snowflake**: A cloud-based data warehousing platform, Snowflake allows domain-specific teams to autonomously manage their own datasets. As such, it is well-suited for growing data workloads in a Data Mesh environment.
8. **Delta Lake**: An open-source storage layer, Delta Lake ensures the quality and dependability of data across many domains in a Data Mesh by bringing ACID transactions to Apache Spark and big data workloads.
These solutions provide decentralized ownership, scalability, agility, and flexibility in handling heterogeneous datasets across an organization's ecosystem, which forms the basis for implementing a successful Data Mesh architecture.
9. Future Trends in Data Management: The Role of Data Mesh
Data Mesh is anticipated to become more important in data management in the future. A more decentralized strategy is required as long as businesses are to manage enormous volumes of data. By dividing up data ownership and processing duties among various teams or domains, Data Mesh provides a solution that allows for flexibility and scalability when managing intricate data ecosystems.
One prediction is that the Data Mesh framework will be adopted more widely by industries looking to better utilize the potential of their data. Better decision-making procedures inside companies, quicker innovation cycles, and higher data quality are all potential outcomes of this decentralization trend. Adopting the Data Mesh concepts could provide companies a competitive edge as they work to become more data-driven.
Technology developments like artificial intelligence and machine learning will probably accelerate the development of data meshes. In a decentralized data architecture, these technologies can assist in automating processes linked to data discovery, governance, and integration. Organizations can improve the efficiency of their data operations and gain important insights from dispersed datasets by utilizing AI capabilities.
Increased cooperation between domain experts, business stakeholders, and data engineers is another development that is anticipated to influence the direction of Data Mesh in the future. Establishing cross-functional teams tasked with overseeing domain-specific data products helps promote cooperation and ongoing development in an organization's data handling practices. By collaborating, it is possible to dismantle the silos that are typically seen in centralized data structures, improving the alignment of business goals with data strategy.
Prospective prospects for businesses seeking to adeptly handle the intricacies of contemporary data administration are presented by the developments surrounding Data Mesh. Businesses can fully utilize their data assets and propel innovation at scale in the digital age by embracing decentralization, utilizing cutting-edge technologies like AI, and encouraging cooperation among varied teams.
10. Comparing Traditional vs. Contemporary Approaches to Data Management
Conventional methods of managing data frequently depended on centralized data structures, in which information was kept in a single repository or location. Although this centralization made data management and control easier, it also created problems as data volume and variety increased. On the other hand, modern strategies like Data Mesh provide decentralized data architectures, in which data is dispersed over several business divisions or domains.
Since all data passes through a single location, centralized data systems usually have strong governance and control over data security and quality. Unfortunately, this monolithic structure can result in additional complexity when extending storage and processing capacity, lower agility when responding to changing business needs, and bottlenecks when processing huge volumes of data.
Conversely, Data Mesh's decentralized architectures place a strong emphasis on giving domain teams ownership of their data so they may freely maintain their own datasets. This methodology fosters adaptability, scalability, and agility while diminishing reliance on centralized groups to obtain vital data. Data Mesh seeks to free up central IT resources by decentralizing data management, enabling domain experts to make well-informed decisions based on local context.