1. Introduction to Data Cleansing and Maintenance
Ensuring the integrity and dependability of data inside an organization is mostly dependent on data maintenance and cleansing. While data maintenance entails continuous procedures to keep the data accurate and up to date, data cleansing involves finding and fixing flaws and inconsistencies in the data. Enhancing decision-making, operational effectiveness, and overall business performance all depend on these approaches. We will discuss the significance of data management and cleansing in today's data-driven environment in this blog article.
2. The Consequences of Poor Data Quality
Organizations may be greatly impacted by a number of outcomes that might result from poor data quality. Inaccurate, partial, or inconsistent data can lead to poor business decisions that are predicated on false information. This may result in lower productivity, strained client relations, and revenue losses. Relying on inaccurate data could lead to decision-makers misinterpreting market trends, selecting the incorrect target audience, or making bad strategic decisions.
Risks to compliance and regulations might arise from poor data quality. Errors in data can result in harsh penalties or legal ramifications in sectors like healthcare and finance where correct record-keeping is essential for legal reasons. Dealing with dirty data increases the likelihood of data breaches and security issues as well because inaccurate information can expose systems to cybercriminals for exploitation.
An organization's capacity to launch effective marketing efforts or provide individualized client experiences is hampered by poor data quality. Businesses find it difficult to successfully segment their customers without clear and trustworthy data, squandering money on irrelevant or incorrectly targeted advertising. This might damage the reputation of the brand and eventually lower client happiness and loyalty.📘
Poor data quality has wide-ranging effects that affect many facets of how organizations operate. In today's data-driven business environment, the need of preserving clean and correct data cannot be emphasized, as it can jeopardize decision-making processes, increase operational expenses, risk legal compliance, and impede consumer engagement initiatives.
3. Benefits of Regular Data Cleansing
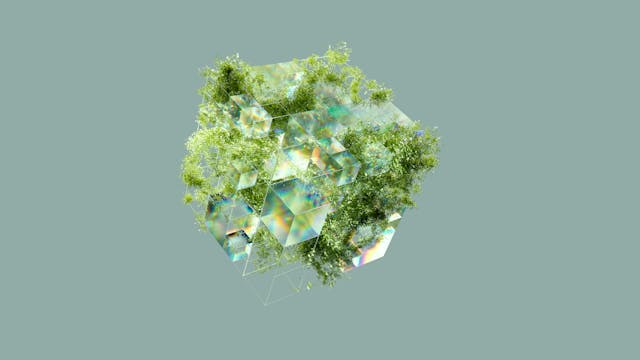
Organizations can reap many benefits from routine data cleansing. First of all, it guarantees data accuracy by locating and fixing any mistakes, discrepancies, or out-of-date information. Better decision-making processes based on accurate and current data result from this. Second, by cutting down on the time and effort required to find the right information in a jumbled database, cleansing improves operational efficiency. Businesses may improve productivity and expedite processes by keeping data clean and well-organized.
Better client interactions can be achieved through routine data cleansing. Clean data makes it possible to communicate with customers in a focused and personalized way, which increases engagement and retention rates. Businesses are able to customize their goods and services by using the insightful information it provides about consumer behavior and preferences. A detailed picture of corporate performance and trends over time is provided by accurate data, which also improves analytics and reporting.
An essential component of regulatory compliance is data cleansing. Strict laws governing data security and privacy apply to many businesses. Organizations may make sure they are in compliance with these requirements and avoid costly fines or legal difficulties related to obsolete or erroneous information by routinely cleansing their databases. Regular data cleansing expenditures protect the organization's integrity and reputation over time in addition to increasing operational efficiency.
4. Strategies for Effective Data Cleansing
Sustaining data correctness and integrity requires effective data cleansing. By putting the appropriate ideas into practice, you may expedite this process and guarantee that your database stays current and dependable.
Setting criteria for data quality is an important tactic. Describe for your company what clean, correct data looks like, including the formats, degrees of accuracy, and consistency needed. This will function as a standard by which you may evaluate the success of your data cleaning initiatives.
Process automation for data purification is another useful tactic. Make use of instruments and programs that can automatically detect flaws, duplications, and inconsistencies in your database. This minimizes the possibility of human error during the cleaning procedure in addition to saving time.
It is crucial to regularly check the quality of the data. Set up reports or alerts that show possible problems in real time so you can take quick action. You may prevent problems from getting worse by being vigilant about reviewing the quality of your data.
Departmental cooperation is essential to successful data cleansing. To make sure that everyone is aware of how important it is to have clean data, promote communication amongst teams who work with the data. You may make the organization's approach to data upkeep more unified by encouraging a culture of cooperation.
Using these techniques in your data cleansing process will help you make better decisions based on accurate and trustworthy data, as well as increase the quality of your database. Setting data cleaning as a top priority is essential to guaranteeing the prosperity and effectiveness of your company's operations.
5. Tools and Technologies for Data Maintenance
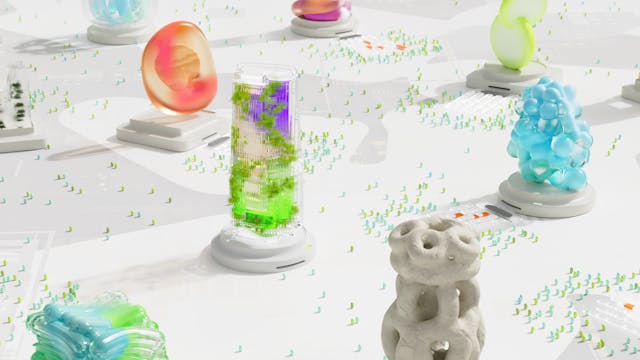
Businesses can simplify the process of maintaining data integrity and quality by utilizing a range of tools and technology. Organizations are assisted in maintaining a single, consistent version of their data across many sources by data management platforms like Master Data Management (MDM) systems. These systems support the resolution of discrepancies, the removal of duplicates, and the correctness of data.
Tools for data quality are yet another crucial element of data upkeep. These technologies aid in the detection and correction of mistakes, discrepancies, and duplications in datasets. To improve the quality of the data, they offer features like enrichment, standardization, cleaning, and profiling. Informatica Data Quality, Talend Data Quality, and IBM InfoSphere Information Server are a few well-liked data quality products.
Data Integration Tools are essential for ensuring data consistency since they make information transfer across various applications and systems easier. By combining data from multiple sources into a single, cohesive view, these solutions aid in making sure that all systems are operating with correct and up-to-date data.
Tools for automated monitoring are quite helpful in maintaining data over time. These technologies continually and instantly scan data streams for anomalies or disparities. Organizations can swiftly handle concerns as they arise, preventing them from developing into larger ones, by setting up alerts and notifications.
Furthermore, as I mentioned above, companies who want to maintain the dependability and integrity of their data assets must make the necessary investments in the appropriate tools and technologies for data maintenance. Organizations may guarantee the accuracy, consistency, and value of their data for making well-informed business decisions by putting in place reliable data management platforms, quality tools, integration solutions, and automated monitoring systems. 😽
6. Best Practices for Sustainable Data Management
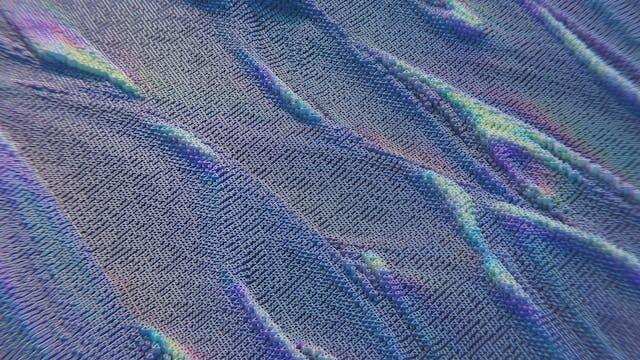
6. Best Practices for Sustainable Data Management:
Implement Regular Data Audits: Perform regular audits to check the quality, accuracy, and relevance of your data. This helps in identifying any inconsistencies or errors that need to be corrected.
Clearly define your data governance policies: Clearly define roles, duties, and protocols for data management inside your company by creating data governance policies. This guarantees that all individuals comprehend the proper methods for managing and preserving data.📂
Invest in Data Quality technologies: You can automate the process of finding and fixing mistakes in your data by using data quality technologies. By using these tools, you can make sure that your data is correct and up to date while also streamlining the process of data cleansing.
Educate Your Staff on Best Practices for Data Management: Your staff should receive training on data management best practices, including how to gather, store, and analyze data correctly. This gives your team the ability to decide wisely using reliable data.
Record Your Data Processes: By keeping track of your data management procedures, you can ensure uniformity and transparency in the way data is handled throughout your company. It also acts as a point of reference for upcoming evaluations or upgrades.
Track Data Usage and Performance: Keep a close eye on the way data is used in your company to see any possible problems or areas that may need better. Over time, you can improve your data management procedures by monitoring performance metrics.
Maintaining your organization's data assets' integrity and utility requires following certain best practices. You can make sure that your data is accurate, dependable, and useful for making wise business decisions by putting these ideas into practice.
7. Role of Automated Processes in Data Cleansing
Because they expedite and simplify the process of finding and fixing problems in datasets, automated techniques are essential to data cleansing. These procedures make use of rules and algorithms to find duplicates, formatting mistakes, inconsistencies, and other problems that could lower the quality of the data. Organizations can save time and money while maintaining the accuracy and dependability of their data by automating this procedure.
The efficacious handling of substantial data quantities is a primary benefit of automated operations in the context of data purification. Cleaning data by hand can be laborious and prone to mistakes, particularly when working with large datasets. Organizations can swiftly and reliably clean their complete dataset thanks to automation's assistance in performing these operations at scale.
The possibility of human error involved in manual data cleansing is decreased by automation. Organizations can reduce the chance of adding new errors to the information during the cleaning process by automating repetitive processes like standardizing formats or eliminating duplicates. Overall data reliability and accuracy are increased as a result of this.
It is possible to program automated procedures to execute on a predetermined timetable, guaranteeing that data is accurate and up to date throughout time. Organizations can prevent problems from developing by proactively addressing them as soon as they appear through the establishment of automated protocols for data upkeep. This proactive strategy helps to preserve high-quality data, which improves operational effectiveness and decision-making.
Taking into account everything mentioned above, we can say that automated processes are essential to data cleansing since they facilitate proactive maintenance procedures, enhance accuracy, and streamline operations. In addition to saving time, using automated technologies for data purification guarantees that businesses have access to dependable, high-quality data for their operational requirements.
8. Security Considerations in Data Maintenance
Security issues are crucial when it comes to data maintenance. Maintaining the trust of your stakeholders and consumers, in addition to safeguarding sensitive information, depends heavily on data security. Data maintenance should include security safeguards in all areas, including transmission, storage, and access.
Improving data security is largely dependent on data cleansing. You may detect and get rid of any possible security threats, such out-of-date or erroneous information that can jeopardize the integrity of your database, by routinely organizing and cleaning your data. You may lower the risk of breaches or unauthorized access by using data cleansing to make sure that only authorized individuals have access to sensitive information.
Using encryption methods is just another crucial step in keeping your data safe while performing maintenance. By transforming your data into a secure format that cannot be read without the right decryption key, encryption helps protect your information. This provides an additional degree of security, particularly when moving data between several systems or storage sites.
In order to quickly identify any suspicious activity or illegal access attempts, regular monitoring and auditing of data maintenance activities is essential. You can keep track of who has access to the data, when they have access to it, and what changes have been done by logging and analyzing all database adjustments. This supports adherence to laws pertaining to data security and privacy as well as the detection of any security breaches.
Strict access restrictions and user permissions must be implemented in addition to technology safeguards to ensure the security of your data throughout maintenance procedures. Restricting access to sensitive data according to roles and responsibilities guarantees that only individuals with the proper authorization can access and alter particular datasets. Your data maintenance procedures' overall security posture is strengthened even more when these access controls are routinely reviewed and updated.
Protecting sensitive data, stopping illegal access, and upholding regulatory compliance all depend on giving security considerations first priority while managing data. Strong security features like data encryption, cleansing, monitoring, auditing, and access controls can help you reduce risks and safeguard the confidentiality and integrity of your important company data.
9. Impact of Clean Data on Decision Making
Organizational decision-making processes are greatly impacted by clean data. Businesses may make better decisions based on dependable information if they make sure the data is accurate, consistent, and up to date. Clean data reduces the possibility of mistakes or inconsistencies in analysis, improving forecasting and strategic planning.
Decision-makers can trust the insights gleaned from data when they have access to clean, trustworthy data. Because of their increased confidence, they are able to make decisions more quickly and precisely, which eventually improves operational efficiency and gives them a competitive advantage in the market. A clear and accurate view of corporate performance and trends is provided by clean data, which lays a solid platform for decision-making.
Reports and presentations prepared for investors or stakeholders have more credibility when they provide clean data. Trustworthy data sources guarantee the accuracy of key performance indicators, which promotes confidence in the organization's capacity to monitor advancement and accomplish objectives. Accurate data enables decision-makers to communicate effectively, which is crucial for forging strong bonds with stakeholders.😐
In summary, it is impossible to overestimate the influence of clean data on decision-making. It serves as the cornerstone of strategic planning, promotes flexible decision-making, strengthens reporting credibility, and eventually propels the success of organizations. Long-term success in business depends critically on investments made in data management and cleansing, as companies continue to rely on data-driven insights for growth and competitiveness.
10. Measuring the ROI of Data Cleansing Efforts
Understanding the influence and efficacy of your data maintenance techniques requires measuring the return on investment (ROI) of your data cleansing activities. Businesses can justify the resources used for data cleansing operations and make future decisions by calculating the return on investment.
It is crucial to set up key performance indicators (KPIs) that correspond with your business goals in order to calculate the return on investment (ROI) of data cleansing. These key performance indicators (KPIs) can be higher operational efficiency, decreased duplication of records, improved data accuracy, or improved decision-making based on clean data.
The process of calculating return on investment (ROI) entails weighing the actual advantages of data cleansing operations against their associated costs. Software tools, employee time, training, and any other resources needed to clean and maintain the quality of the data may all incur costs.
Benefits, on the other hand, are evident in a number of areas, including increased customer satisfaction, more accurate and clean data supporting streamlined processes, higher conversion rates from marketing campaigns with better targeting, fewer errors in reporting and analysis, and overall organizational efficiency.
You can further hone your data cleansing techniques based on what functions best for your company as you monitor the outcomes throughout time. You can be sure that your data maintenance activities will always be valuable in the long run and in line with business objectives by using this iterative method of measurement and improvement.