1. Introduction
The term "Big Data" has gained popularity in today's data-driven world to describe the enormous volume of organized and unstructured data that is produced daily by organizations, people, and devices. This data has a wealth of insights that could spur creativity, enhance decision-making, and enhance many facets of our life. Big Data presents opportunities and problems for companies seeking to harness its potential due to its volume, velocity, and variety.
One less well-known but no less significant component of Big Data is the idea of "Mixed Data." The term "mixed data" describes the variety of data sources—such as text, photos, videos, sensor data, social media posts, and more—that companies gather and examine. Mixed data is unstructured or semi-structured in form, in contrast to standard structured data seen in databases or spreadsheets. To extract useful insights, modern technologies like machine learning and natural language processing are needed.
In the context of big data, understanding mixed data is essential because it allows businesses to access a greater variety of information sources and obtain a more complete understanding of their consumers, operations, and market trends. Businesses can find hidden patterns, correlations, and anomalies that might not be seen when concentrating only on structured data sets by implementing mixed data analysis into their data strategies. In today's fast-paced corporate world, this comprehensive approach to data analysis enables firms to stay competitive and make better decisions.
2. Understanding Big Data
The four primary characteristics of big data, referred to as the "4 Vs," are volume, velocity, variety, and veracity. Volume describes the enormous volume of data produced per second. The pace at which this data is generated and must be processed is referred to as its velocity. Variety draws attention to the different kinds of data that are accessible from different sources, including organized and unstructured data. The correctness and dependability of the data gathered are issues of veracity.
Traditional data processing technologies provide considerable obstacles when handling enormous volumes of data. The sheer amount of data may be too much for traditional databases to handle efficiently, which could result in scalability and query performance concerns. Large-scale dataset storage and analysis can put a burden on resources, requiring increasingly sophisticated tools and methods designed for big data analytics. Processing jobs get more complex when quality and integrity of several data kinds are ensured. Therefore, embracing cutting-edge solutions made to manage big data effectively is necessary to react to these challenges.🤏
In a world where information is being created at an unprecedented rate, it is critical for enterprises to comprehend the features and difficulties of big data if they are to successfully leverage its potential benefits.
3. What is Mixed Data?
Within datasets, mixed data is the mixing of unstructured and structured data types. High levels of organization characterize structured data, which usually fits neatly into tables with rows and columns, similar to database information. On the other side, unstructured data is anything that isn't in a set format and includes things like social media postings, texts, photos, and videos. These two are combined in mixed data, which provides a more thorough view that, when examined, can yield more profound insights.
Examples of diverse data sources in real-world situations abound. Consider social media platforms as an example. They produce both unstructured elements like comments and hashtags and structured data like user profiles and interaction analytics. In a similar vein, structured sensor readings are gathered by IoT devices and combined with unstructured user feedback or environmental reports. Businesses can obtain a deeper insight of their operations, client preferences, market trends, and other topics by combining the two types of data.
4. Importance of Mixed Data in Big Data Analytics
Due to its ability to enhance traditional data analytics procedures, mixed data is essential in the field of big data analytics. Text, photos, videos, and social media interactions are examples of mixed data, whereas structured data, such as numbers and categories, is the main focus of traditional data analysis. Businesses can get a more complete picture of their operations and customer interactions by integrating mixed data into analytics processes.👗
The ability of mixed data to offer a more comprehensive knowledge of complicated events is one of its main advantages. Businesses can find hidden patterns and connections that would go unnoticed when examining structured data alone by integrating several types of data sources, such as sales numbers, social media sentiment analysis, and consumer feedback comments. Organizations are able to make better decisions because to this deeper level of knowledge, which is based on a deeper comprehension of market trends and their target audience.
Organizations can extract important insights from unstructured datasets by utilizing advanced analytics techniques such as image identification, machine learning algorithms, and natural language processing (NLP) with mixed data. These tools enable firms to more accurately forecast client behavior, find trends in image or video data, and evaluate textual material for sentiment analysis. Businesses may increase customer happiness, drive innovation based on real-time information, and optimize their marketing tactics by utilizing the potential of mixed data analytics.
From the foregoing, it is clear that integrating mixed data into big data analytics procedures is necessary to acquire a deeper understanding of the situation and make wise choices in the cutthroat business environment of today. Embracing the variety of data sources at their disposal and utilizing cutting-edge analytical tools can help firms seize new chances for expansion, productivity increases, and improved client experiences. In the digital age, mixed data is not only a supplement to traditional analytics, but a revolutionary force that drives businesses to make better decisions and achieve long-term success.
5. Processing Mixed Data
In a Big Data environment, processing heterogeneous data requires a number of preprocessing and analysis techniques. Data standardization is a popular strategy that involves transforming many data types into a uniform format to facilitate analysis. Data cleansing, which includes locating and addressing outliers, inconsistent data, and missing values in the data set, is another crucial tactic.
Feature engineering and other sophisticated techniques can be used to properly handle mixed data. The goal of this method is to extract meaningful patterns from the data and enhance model performance by generating new features from the existing ones. Principal component analysis (PCA), one technique for reducing dimensionality, can assist in decomposing large mixed datasets into simpler forms while preserving crucial information.
In the field of tools and technologies used for managing mixed data in a Big Data context, platforms like Apache Hadoop and Apache Spark are attractive alternatives due to their scalability and capacity to handle varied data types. These technologies include features for effectively handling both organized and unstructured data. Specialized applications such as KNIME and RapidMiner provide user-friendly interfaces that facilitate the preprocessing and analysis of mixed data without requiring substantial programming experience. By incorporating these technologies into a big data architecture, it is possible to manage different datasets more efficiently and derive valuable insights.
6. Applications of Mixed Data Analysis
By gleaning insightful information from a blend of structured and unstructured data sources, mixed data analysis has transformed a number of industries. In healthcare, studying mixed data has increased patient care through predictive analytics, enabling early disease detection and individualized treatment regimens. Financial companies use mixed data to improve investment strategies, identify fraud tendencies quickly, and evaluate credit risk more precisely. Through the exact targeting of marketing efforts to specific demographics, businesses may greatly increase customer engagement and sales conversions by combining transactional data, social media sentiment analysis, and customer feedback.
The impact of mixed data analysis on firms is considerable across numerous industries. In healthcare, the integration of electronic health records with genomic data has prepared the path for precision medicine, where therapies are tailored to individual genetic profiles for improved patient outcomes. Financial firms benefit from mixed data analysis by recognizing market movements sooner utilizing a combination of traditional financial measures and nontraditional data sources like social media sentiment or weather patterns. Marketing efforts have grown more targeted and effective through the study of customer behaviors across many touchpoints such as online interactions, in-store purchases, and feedback surveys.
Mixed data analysis has also altered supply chain management by offering real-time visibility into inventory levels, demand variations, and supplier performance. By merging structured data from ERP systems with unstructured data from IoT sensors or social media discussion about products, organizations may improve their supply chains for efficiency and cost savings. In the retail industry, evaluating mixed data enables companies to personalize customer experiences both online and offline by understanding shopping preferences, browsing history, demographic information, and sentiment analysis from social media platforms.
The applications of mixed data analysis are numerous and impactful across varied areas like healthcare, finance, marketing, supply chain management, and retail. By embracing the potential of structured and unstructured data integration approaches, businesses may acquire actionable insights that fuel innovation, boost decision-making processes...💡
7. Challenges and Future Trends
Challenges in dealing with Mixed Data encompass privacy problems coming from the complexity of merging diverse data kinds, causing issues linked to data security and compliance. Different data sources have different forms and structures, which makes it difficult to combine and use them efficiently. This leads to integration difficulties. Overcoming these barriers needs robust data governance frameworks, powerful encryption mechanisms, and extensive data integration strategies to ensure seamless operations while securing sensitive information.
Looking ahead, harnessing Mixed Data for improved decision-making and predictive analytics is projected to continue rising in significance. Future trends imply the growth of AI-powered technologies to streamline data processing, enabling enterprises to extract useful insights from heterogeneous datasets more quickly. Advancements in machine learning algorithms will boost predictive modeling accuracy, helping organizations to make informed decisions based on extensive analyses of different data kinds. Embracing these trends will be vital for staying competitive in a data-driven future where Mixed Data plays a pivotal role in generating innovation and maximizing corporate outcomes.
8. Case Studies on Successful Implementation
Case Study 1: Retail Giant A leading retail chain implemented mixed data analysis to revamp its marketing strategy. By combining customer transaction data with social media trends and weather patterns, the company optimized product offerings and timing of promotions. This resulted in a significant increase in sales and customer satisfaction.
Case Study 2: Healthcare Provider
A healthcare provider exploited mixed data analysis by combining patient records, treatment outcomes, and demographic information to tailor patient care. By understanding each patient's specific needs better, the practitioner improved treatment outcomes and cut hospital readmission rates dramatically.
Case Study 3: Financial Institution
A large financial institution employed mixed data analysis to strengthen fraud detection systems. By evaluating transaction histories alongside user behavior patterns and geolocation data, the institution may spot potential fraudulent activity in real-time, securing its customers' assets effectively. Significantly less money was lost as a result of fraud because to this preventive strategy.
These case studies highlight how organizations across diverse industries have leveraged the potential of mixed data analysis to generate important insights, improve decision-making processes, and produce meaningful business results.
9. Tools for Managing Mixed Datasets
When it comes to handling mixed datasets, there are various tools and platforms that can help streamline the process for those wishing to explore further. Apache Hadoop is a popular choice due to its capacity to process massive amounts of assorted data types efficiently. Another robust tool is Apache Spark, noted for its speed and agility in handling varied datasets.
Tools like Tableau and Microsoft Power BI provide easy-to-use solutions for visualizing and analyzing mixed datasets without requiring a lot of coding expertise, making them ideal for customers looking for a more user-friendly interface. Talend is a sophisticated platform that provides complete data integration capabilities for managing complicated mixed datasets effectively.
Those diving into mixed data may also benefit from tools such as IBM InfoSphere Information Server and Alteryx, which offer advanced features like data profiling, cleansing, and blending to ensure accuracy and consistency across varied data sources. When dealing with mixed datasets, the appropriate tool selection is contingent upon particular requirements and preferences.
10. Pros and Cons
A balanced perspective on Big Data, including its benefits and drawbacks, can be obtained by embracing mixed data. Combining different data kinds yields a more thorough understanding of processes and events, which is a plus. Organizations can gain more profound understanding, reveal obscure trends, and make well-informed decisions by utilizing a variety of information sources thanks to this comprehensive approach.🖊
But managing mixed data can be complicated, which can be a big disadvantage. To assure accuracy and reliability, integrating varied data sets from multiple sources calls for advanced tools and procedures. Managing the volume, diversity, and velocity of mixed data can be intimidating, leading to issues in data quality assurance, processing speed, and storage capacity.
It takes a deliberate strategy that makes use of machine learning algorithms, advanced analytics, and sound data management techniques to balance the benefits and drawbacks of mixed data. Organizations may fully realize the promise of Big Data to drive innovation, improve decision-making processes, and gain a competitive edge in today's data-driven world by addressing the complexity and using the benefits of different data sources.
11. Ethical Considerations
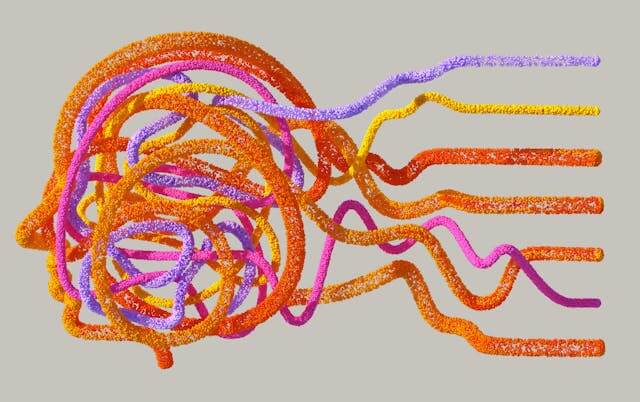
Because working with mixed data entails managing a range of information gathered from many sources, ethical considerations are crucial. Prioritizing the rights and privacy of the people whose data is being combined is vital. Essential ethical principles for managing mixed data include adhering to legal limitations, gaining consent, maintaining data security, and anonymizing sensitive information. 🤝
Organizations must be open and honest about their data collection procedures and goals while gathering mixed data. People who entrust their data to us do so because of the transparency that helps them understand how it will be utilized. Promoting ethical practices when handling mixed data is facilitated by making sure the data obtained is correct and pertinent to the desired analysis.
Ethical mixed data analysis calls for the application of suitable techniques that preserve the accuracy of the data collected. Data manipulation or misinterpretation can have serious repercussions for both people and society as a whole. Thus, it is imperative that researchers and analysts follow ethical guidelines, which include eliminating biases in their analysis and providing accurate results.
When using mixed data responsibly, one must take precautions to prevent misuse or harm from resulting from its distribution. Clear standards on data usage, sharing, and retention should be established by organizations to stop unethical actions like discrimination or exploitation based on insights from mixed data. Maintaining ethical standards when using mixed data requires giving priority to the welfare of those affected by decisions made using data.
12. Conclusion
In summary, mixed data—which combines various forms of structured and unstructured data—plays a critical role in big data analytics by offering an all-encompassing perspective for analysis. Businesses are able to make better decisions, obtain deeper insights, and find important trends that might not be apparent when examining a single type of data alone. Through the integration of many data sources, including text, photos, videos, and sensor data, enterprises may fully leverage the potential of big data analytics to spur innovation and maintain their competitive edge in the current data-driven business environment.
Businesses hoping to glean valuable insights from their massive volumes of data must comprehend mixed data. As technology develops and new types of data appear, it will be more crucial than ever to handle and evaluate mixed data efficiently. Adopting mixed data improves decision-making procedures and helps businesses respond more quickly to shifting customer and market trends.
Going forward, mixed data will become even more important in big data analytics as businesses look for more comprehensive perspectives on their clients and operations. Through the acknowledgement of the significance of amalgamating diverse data sources and allocating resources towards technologies that facilitate mixed data analysis, enterprises might unleash novel prospects for expansion and novelty. In the age of big data analytics, embracing mixed data is not simply a trend—it's a necessary tactic.