1. Introduction
The precision, dependability, and applicability of the data that insurers use to evaluate risks, make decisions, and provide customer service are referred to as data quality in the insurance sector. For insurers, having high-quality data is essential since it affects many parts of their business, from precisely underwriting and pricing policies to spotting fraud and guaranteeing regulatory compliance. Data is a key component used by insurers to assess risks, develop customized policies, and improve client satisfaction. Insurance companies may have higher financial risks, operational inefficiencies, compliance problems, and a loss of market advantage in the absence of high-quality data.
Good data quality helps insurers make better decisions, analyze risks more effectively, run their businesses more efficiently, and satisfy customers. Insurers can more precisely price their insurance products based on individual risk profiles and market conditions when they have access to accurate and trustworthy data. By examining trends and discrepancies in the data, it also aids in the early detection of fraudulent activity. Because it enables insurers to provide individualized services based on each client's needs and preferences, high-quality data improves the customer experience. Superior data quality can be a crucial differentiator that helps successful insurers stand out from their rivals in the fiercely competitive insurance market.
2. Implications of Poor Data Quality
In the insurance sector, low-quality data can have far-reaching effects that affect insurers in several ways. Because of inefficiencies in the underwriting and claim processing processes, inaccurate or missing data might result in increased operational expenses. Inaccurate risk assessment can also lead to inappropriate policy pricing and the possible loss of clients to rivals that provide better-fitting goods.
Inadequate data quality can impede efforts to comply with regulations, resulting in penalties and harm to insurance businesses' reputations. Executives may be working with inaccurate information that influences strategic planning and corporate outcomes when dealing with inconsistent or untrustworthy data, which can further obstruct decision-making processes.
Poor data quality can cause a number of problems, such as delays in processing claims because of incomplete or inaccurate information, which can leave consumers unhappy and increase customer churn. Inaccurate client profiles can affect insurers' income streams by causing them to miss out on cross-selling or upselling opportunities.
Insurance businesses may suffer financial losses if fraud goes undiscovered as a result of underwriting choices made using inaccurate data. Inadequate data quality management may also put insurers at risk of cybersecurity breaches and undermine policyholder trust by jeopardizing the protection of critical client data.
3. Why Improve Data Quality?
The insurance business needs to improve the quality of its data for a number of important reasons. Increased accuracy and dependability in risk assessment, pricing strategies, and claim processing are directly correlated with improved data quality. Consequently, this raises client contentment and confidence in the insurance company. By having high-quality data, insurers may cut errors, fraud, and operational expenses dramatically.
Insurance businesses may make better decisions as a result of high-quality data. Making more informed strategic decisions based on insights derived from complete and accurate information is made possible by having access to timely and dependable data. This may lead to more effective risk management plans, focused marketing campaigns, and optimized operational procedures. Improved data quality can lead to better decision-making, which can offer insurers a competitive advantage in the market.
Insurance companies gain from efficient data management techniques because they simplify underwriting, claims processing, and customer support. Increased data quality guarantees information availability when needed, cutting down on delays and promoting a more efficient workflow. Insurance companies may automate repetitive processes, lower manual errors, and allocate resources more effectively by utilizing high-quality data. Long-term cost optimization for insurance businesses is also facilitated by this enhanced efficiency, which saves time.
4. Strategies for Improving Data Quality
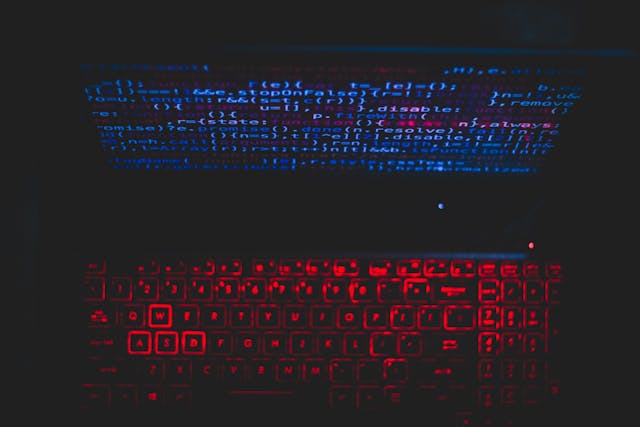
Enhancing the quality of data in the insurance sector is essential to guaranteeing precise risk evaluation and decision-making. To find and fix data mistakes early on, it's essential to put strong data validation procedures into place. To improve overall data quality, this entails confirming data accuracy, consistency, and completeness.
By automating procedures like data cleansing, deduplication, and anomaly detection, the use of cutting-edge technology like artificial intelligence (AI) and machine learning can greatly improve the quality of data. By effectively managing huge datasets, spotting trends, and anticipating any problems before they happen, these technologies can ultimately improve the accuracy of data used by the insurance sector.
Sustaining high standards for data quality requires training staff on data management best practices. Accurate data entry and maintenance, adherence to standard operating procedures, and the ability to identify frequent mistakes can all help keep errors out of the system. Employees are kept up to date on the latest developments in data quality management best practices through ongoing training.
Insurance businesses can increase their operational efficiency, lower the risks associated with erroneous data, and ultimately offer better services to their clients by putting these ideas for data quality improvement into practice. In an increasingly data-driven sector, insurance companies will surely be better positioned for success if they take a proactive approach to improving the quality of their data.
5. Data Governance Framework
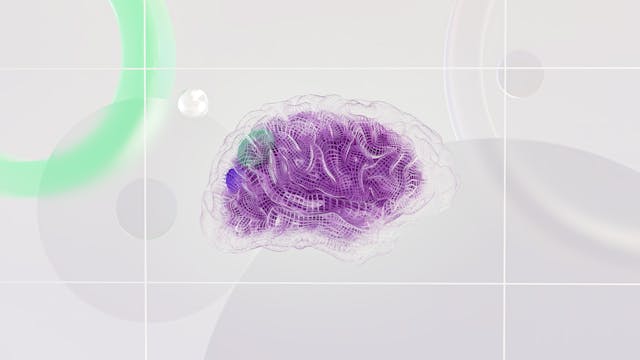
Improving data quality in the insurance sector requires a robust data governance structure. It offers an organized method for effectively managing, utilizing, and safeguarding data assets. Governance makes sure that data is reliable, consistent, safe, and easily available by defining the rules, practices, roles, and duties associated with data management.
Clearly establishing data ownership is essential to an effective data governance approach because it establishes accountability for problems with data quality. Positions in data stewardship are crucial for managing particular datasets and guaranteeing their dependability and correctness. Consistency between systems and applications is maintained by the implementation of data quality standards and controls.
By establishing data access and security policies, confidential information is protected from misuse or security breaches. Assessing the efficacy of the governance system and swiftly correcting any deviations need regular monitoring, auditing, and reporting procedures. Working together with stakeholders from IT, business units, compliance, and risk management is also necessary to guarantee a comprehensive approach to data governance and to align goals.
6. Importance of Data Cleaning and Standardization
The insurance sector relies heavily on data cleaning and standardization to improve the quality of its data. Data cleaning produces more accurate and dependable datasets by locating and fixing mistakes, inconsistencies, and duplicates. By maintaining consistent formats, structures, and norms across various sources, standardizing data facilitates information comparison and analysis.
In the insurance industry, clean, standardized data is essential for accurate reporting and analysis since large volumes of data are gathered from multiple sources, including policies, claims, and client information. Inaccurate or inconsistent data can produce faulty insights and judgments if appropriate data cleansing procedures aren't followed. By bringing different datasets into harmony, standardization enables analysts to draw meaningful inferences and make defensible business judgments.
In addition to improving data quality, standardizing and cleaning it also helps insurance companies' reporting and analytical procedures run more smoothly. Clean data makes it easier for stakeholders to trust the conclusions drawn from analytics by lowering the possibility of mistakes or misinterpretations in reports. Insurance firms can increase productivity, compliance efforts, and overall decision-making based on reliable information by establishing clear rules for data collecting and storage processes.
To put it succinctly, adopting strong data cleansing and standardization procedures is crucial for raising the caliber of data in the insurance sector. At every level of an insurance company, these procedures not only improve accuracy but also provide improved reporting capacities and more knowledgeable decision-making.
7. Leveraging Data Analytics Tools
Improving data quality in the insurance sector requires the use of data analytics techniques. Insurance companies can efficiently detect and address problems with data quality that could affect operations and decision-making procedures by employing sophisticated analytics technologies. With the help of these solutions, insurers may optimize their data management systems and guarantee the correctness, consistency, and dependability of the data they hold.
Insurance businesses can improve risk assessment procedures and their predictive modeling skills by investing in data analytics solutions. Insurance companies can gain important insights from large datasets through the use of complex algorithms and technologies, which improves prediction accuracy and facilitates well-informed decision-making. This approach enhances operational efficiency and empowers insurers to provide more customized goods and services to cater to the changing demands of their clientele.
Insurance businesses are better equipped to remain competitive in a market that is changing quickly by integrating data analytics tools into their everyday operations. Through the adoption of these technologies, insurance companies can seize fresh chances for expansion, creativity, and client fulfillment. In the insurance industry, proactive risk management plans and environmentally friendly business practices are made possible by the insights obtained by sophisticated analytics technologies.
8. Collaborations with Third-Party Services for Data Enhancement
The insurance business may significantly improve the quality of its data by working with third-party providers. In order to assure more accurate and current data, insurers can collaborate with external firms to perform activities like client information verification or make use of external databases. Through these relationships, access to a multitude of information that would not be easily obtained internally is made possible, which eventually improves risk assessment, creates more individualized policies, and enhances customer service.
Tools and technologies are available from third-party data augmentation providers that can help expedite the process of validating and enriching datasets. By using advanced analytics and modeling approaches, they may offer insightful information on the habits, preferences, and risk profiles of their customers. Insurance businesses may get a more complete picture of their clients and make better judgments by combining data from external sources with internal datasets.
Insurers can maintain compliance with privacy and data accuracy standards by working with third-party providers. In an industry as heavily regulated as insurance, external partners can be extremely helpful as they frequently possess the skills needed to handle sensitive data safely and ensure regulatory compliance. Under strict guidelines for data security and compliance, insurers can reduce the risks related to old or erroneous data by collaborating closely with reliable third-party suppliers.
Taking into account everything mentioned above, we can say that insurance businesses who want to raise the caliber of their datasets would be wise to collaborate with outside providers of data augmentation services. Through these partnerships, companies can have access to cutting-edge technologies, knowledge, and external resources that help improve decision-making, improve consumer experiences, and guarantee regulatory compliance. In an increasingly data-driven sector, insurers can gain a competitive edge by utilizing the potential of external data sources through strategic partnerships.
9. Monitoring and Continuous Improvement
Improving data quality in the insurance sector requires constant monitoring and development. Through the implementation of comprehensive monitoring protocols, such as periodic audits and feedback loops, insurers can guarantee long-term, consistent enhancements in their data quality standards. These procedures not only facilitate the early detection of inconsistencies or mistakes but also allow businesses to move quickly to implement remedial measures. Feedback loops give businesses useful information about areas that require improvement, enabling them to put focused initiatives into place to increase the accuracy and dependability of their data.
Ensuring that data quality criteria are continuously followed within an insurance organization is made possible through regular audits. These audits entail in-depth analyses of systems, procedures, and datasets to find any discrepancies or errors that can jeopardize the accuracy of the data. Insurers can proactively fix problems before they become more serious by carrying out these audits at predetermined intervals, which helps them maintain high standards of data quality throughout their operations. Audits function as a means of evaluating the efficacy of current data quality endeavors and pinpointing specific areas that require additional improvement.
Insurance businesses can dynamically obtain feedback on their data quality processes from a variety of stakeholders by utilizing feedback loops. Through the process of asking staff members, clients, and partners about their experiences with data-related procedures, insurers can obtain a thorough grasp of any potential problems or areas that need attention. This first-hand knowledge can then be applied to improve current tactics, revise regulations, or carry out training initiatives meant to successfully handle difficulties that have been discovered. Essentially, feedback loops establish an ongoing conversation about data quality inside a company, encouraging innovation and teamwork.
Any effective data quality strategy in the insurance sector must include monitoring tools and promote a continual improvement culture. Insurers should proactively address concerns regarding data accuracy and consistency while promoting continuous improvements throughout their operations by putting in place frequent audits and feedback loops. Putting money into strong monitoring procedures helps insurance businesses succeed in the long run in an increasingly data-driven environment while also ensuring compliance with regulatory obligations.
10. Regulatory Compliance and Data Security Considerations
In the insurance sector, ensuring data quality involves not just completeness and correctness but also data security and regulatory compliance. Strict criteria for processing personal data are outlined in regulations like GDPR and HIPAA in order to preserve people's privacy. Insurance businesses can prevent breaches and unauthorized access to sensitive consumer information by upholding rigorous security standards.
Insurance businesses must abide by laws like GDPR in order to avoid paying large fines and keep their consumers' trust. Having correct documentation, appropriate permission procedures, and safe storage techniques in place are necessary to ensure data quality. Incorporating these practices into their data management procedures can help insurers handle client data with greater responsibility and openness.
In the insurance sector, data security concerns are essential to raising overall data quality. To reduce the danger of data breaches or cyber attacks, encryption techniques, access controls, and frequent security audits should be put into place. Through the implementation of strong cybersecurity protocols, insurance companies can establish a strong framework for safeguarding client data and complying with legal mandates.
Based on the aforementioned, it is imperative to prioritize data security in conjunction with addressing regulatory compliance such as GDPR and HIPAA in order to enhance data quality within the insurance industry. Through the implementation of stringent privacy policies, investment in secure technology solutions, and cultivation of a compliance culture within their organizations, insurers can not only satisfy regulatory requirements but also gain the confidence and allegiance of their clientele by efficiently protecting their confidential data.
11. Case Studies: Successful Implementation Stories
Improving data quality has been a game-changer for several organizations in the insurance industry. Let us examine a few case studies that illustrate the concrete advantages that insurance companies have experienced as a result of improving their data quality protocols.
**Case Study 1: Company X**
Leading insurance provider Company X started a data quality improvement program with the goal of expediting the claims handling process. Through the use of automated data validation tools and frequent audits, they were able to drastically lower the number of errors and fraud cases in claim submissions. Faster claim settlements, higher customer happiness, and ultimately cheaper operating costs for the business were the results of this.🔷
**Case Study 2: Company Y**
Company Y understood how crucial it was to have precise consumer data for their underwriting procedures. They were able to improve the accuracy of risk assessments and identify previously hidden patterns of fraudulent activity by implementing strong data governance procedures and investing in data cleansing solutions. By taking a proactive stance, they reduced possible hazards and enhanced their industry reputation for dependability.
**Case Study 3: Company Z**
Company Z concentrated on using sophisticated analytics technologies to improve their customer segmentation-based marketing techniques. They improved their ability to address the specific needs of each consumer by customizing their product offers through continuous feedback loops and data enrichment procedures. This tailored strategy improved the company's income streams by increasing cross-selling opportunities and client retention rates.
In the fiercely competitive insurance market, these success stories demonstrate how putting data quality first can improve client experiences, reduce risks, and boost operational efficiency all the way to sustainable development.
12. Conclusion: Recap key points and future outlook.
After putting everything above together, we can say that the insurance business must prioritize improving the quality of its data if it is to succeed in the long run. Informed decision-making, risk assessment, fraud detection, and improving customer experience all depend on precise and reliable data. Insurance businesses may improve operations, lower costs related to errors and inefficiencies, and maintain their competitiveness in a market that is changing quickly by investing in data quality projects.
In order to spur innovation and improve customer service, the insurance sector will need to fully utilize the potential of data analytics, machine learning, and artificial intelligence. Insurance companies will be able to anticipate trends, get insightful information, tailor products, and successfully reduce risk by adopting new technologies. Insurance businesses are able to keep ahead of the curve by utilizing cutting-edge tools and focusing on improving data quality standards in order to respond to changing consumer wants and market dynamics.