1. Introduction
**Introduction:**📖
Data is now essential to businesses in the digital age because it fosters innovation, competitive advantage, and decision-making. Big Data is the term used to describe the enormous amount of both organized and unstructured data that constantly floods a company. It includes not just the data itself but also the methods, procedures, and instruments employed in its analysis and subsequent insight-gathering.
One cannot stress the importance of big data for enterprises. Businesses can learn important lessons from it about consumer behavior, industry trends, operational effectiveness, and much more. Effective use of big data can result in more innovative products, better decision-making, tailored consumer experiences, and eventually higher profitability. 🤭
The Big Data Business Model Maturity Index is one methodology that companies may use to evaluate how mature they are in using big data (BDMMI). This index offers a methodical way to assess a company's readiness to use Big Data to improve many facets of its business operations. We will then go into further detail about the elements that make up this index and how it might assist organizations in determining how prepared they are to use Big Data analytics.
2. Understanding the Big Data Business Model Maturity Index
In the context of big data, "business model maturity" refers to how well an organization integrates big data into all of its business operations. It evaluates how well a company has incorporated big data into its operations, general strategy, and decision-making procedures. A well-developed big data business model shows that the company is leveraging the insights from data analysis and collection to spur innovation, enhance customer experiences, and maximize performance.
Organizations can assess their level of big data maturity using a formal framework that is provided by the Big Data Business Model Maturity Index. Typically, this index is made up of a number of indicators or criteria that assess multiple facets of a business's big data capabilities, including data gathering techniques, analytical tools employed, system integration, and application of findings for strategic decision-making. Organizations can determine their big data handling strengths and weaknesses, target areas for improvement, and create goals for improving their big data skills by evaluating where they are on this maturity index.
The Big Data Business Model Maturity Index can help organizations by giving them a clear picture of how mature their big data adoption is at the moment. Through this assessment, they may compare their performance to rivals' or industry standards, identify any gaps in their big data capabilities, and decide which projects or investments to target in order to enhance their big data maturity. Organizations can assess their progress in efficiently utilizing big data by tracking changes in their maturity level over time through this index, which is updated on a regular basis.
3. The Five Stages of Big Data Business Model Maturity
The Big Data Business Model Maturity Index offers a methodology for evaluating the big data adoption level of a company. The five distinct stages of this approach are Managed, Opportunistic, Initiative, Repeatable, and Optimized.
1. **Initiative Stage**: Organizations are just starting to use big data in this early stage. They can be running some pilot programs or irregular initiatives to test out big data technologies. Ad hoc procedures, few resources allocated to big data initiatives, and a lack of a well-thought-out plan are among the salient features. At this point, tasks include learning the fundamentals and investigating possible applications for big data.
2. **Opportunistic Stage**: During this phase, businesses begin to recognize the benefits of big data and take advantage of new opportunities. They might not have a cohesive strategy and be working on multiple separate initiatives at the same time. Increased spending on big data skills and tools is one of its characteristics, but there is still a lack of a comprehensively organized methodology. Expanding the scope of initiatives and finding more focused business cases for big data exploitation are among the activities involved.
3. **Repeatable Stage**: Organizations create more organized procedures around big data activities as they go to this stage. Standardization and consistency between projects are prioritized. Dedicated teams for handling big data efforts, well-defined workflows, and data governance standards are some of the characteristics. Implementing best practices, developing reusable frameworks, and coordinating big data initiatives with corporate objectives are the main activities.
4. **Managed Stage**: In this stage, companies have advanced to a more strategic handling of big data operations and proactive, efficient asset management. Advanced analytical capabilities, integrated data management systems, regulatory compliance (e.g., GDPR, HIPAA), and cross-functional collaboration inside the firm are important attributes. Activities include making sure data security and quality criteria are fulfilled consistently throughout all processes and efficiently utilizing analytics findings.
5. **Optimized Stage**: The last stage is the Optimized phase, in which businesses have completely integrated big data into their main business plan. To spur innovation and increase their competitiveness in the market, they continuously improve their procedures based on information gathered from data analytics. A culture that prioritizes data-driven decision-making across the board and a continuous improvement approach toward using emerging technologies like artificial intelligence (AI) and machine learning in conjunction with big data solutions to maximize business outcomes are among the characteristics.
Comprehending these five phases can assist establishments in assessing their present status regarding the implementation of big data practices and provide guidance on the necessary actions to advance along the maturity index.
4. Assessing Your Organization's Current Level
You can use a self-assessment tool based on the Big Data Business Model Maturity Index to determine the current big data adoption level in your firm. This is an example of a questionnaire that you can use to assess how mature your company is at using big data to drive commercial success:
1. Organizational Strategy:
- Is there a clear alignment between big data initiatives and overall business strategy?
- Are key stakeholders involved in setting strategic direction for big data projects?
2. Data Management:
- Do you have defined processes for collecting, storing, and managing data?
- Is data quality regularly monitored and improved upon?
3. Analytics Capabilities:
- Are advanced analytics techniques (e.g., machine learning) used to derive insights from data?
- Are analytical tools accessible across different departments?
4. Data Monetization:
- Have you identified opportunities to monetize data through new products or services?
- Is there a formal strategy in place for extracting value from data assets?
5. Cultural Readiness:
- Is there widespread understanding and acceptance of big data initiatives within the organization?
- Are employees encouraged to experiment with data-driven approaches?
By answering these questions honestly, you can gauge where your organization stands in terms of big data maturity and identify areas that need improvement.
When assessing your organization's current level using this tool, it's essential to look out for strengths that can be leveraged further and areas where improvements are needed:
Strengths:
1. Clear Strategic Alignment: If your organization demonstrates alignment between big data initiatives and business goals, this indicates a strong foundation for leveraging data effectively.
2. Robust Data Management Processes: Having well-defined processes for managing data suggests that your organization is equipped to handle the complexities of large datasets efficiently.
3. Advanced Analytics Capabilities: Utilizing advanced analytics techniques shows that your organization is moving beyond basic reporting towards deriving valuable insights from data.
4. Data Monetization Opportunities: Identifying ways to monetize data signifies a forward-thinking approach that can lead to new revenue streams.
5. Supportive Culture: A culture that embraces experimentation with data-driven decision-making fosters innovation and continuous improvement.
Areas for Improvement:
1. Strategic Planning: If there is a lack of alignment between big data projects and organizational strategy, investing time in revisiting strategic planning processes could yield better results.
2. Data Quality Assurance: Poor data quality can undermine the effectiveness of analytical efforts, so focusing on improving and monitoring data quality would be beneficial.
3. Skill Development: Ensuring that employees have access to training programs for enhancing their analytical skills can bolster the overall analytics capabilities of the organization.
4. Enhanced Collaboration: Promoting cross-departmental collaboration in utilizing analytical tools can lead to more holistic insights and shared learnings across teams.
5. Change Management: Addressing any resistance or lack of understanding towards big data initiatives through targeted change management strategies can help build a more supportive culture.
Organizations can strengthen their maturity in using big data efficiently for competitive advantage and sustainable growth by identifying both their areas of strength and where they need to improve. This can be done by creating focused action plans.
5. Strategies for Advancing to Higher Maturity Levels
Several best practices must be put into place if you want your company's big data business model to reach higher stages of maturity. First and foremost, it is imperative that the entire firm cultivate a data-driven culture. Encourage staff members to comprehend data and apply it to their decision-making at all levels. Putting money into ongoing training initiatives to improve employees' data literacy can be a big help with this cultural change.
Second, advancement requires establishing data governance and quality. Putting in place strong governance and data quality procedures will increase the organization's trust in the data while also enhancing the accuracy of insights. Maintaining high standards for data quality and governance can be aided by routine audits and compliance checks.
Thirdly, your firm can achieve higher degrees of maturity by utilizing cutting-edge analytics and cutting-edge technology like AI and machine learning. Accurate insights and strategic decision-making can result from investing in systems that support real-time data processing and predictive analytics.
Organizations frequently encounter significant obstacles in their pursuit of higher big data business model maturity levels, even in the face of these best practices. The shortage of qualified personnel in the big data and analytics fields is one prevalent issue. In order to get past this barrier, businesses can concentrate on finding new hires or upskilling current staff members with pertinent experience through training initiatives or collaborations with academic institutions.
Integrating multiple data sources from different departments or systems within the company is another difficulty. This problem can be successfully solved by putting in place a unified data architecture that permits smooth data integration while guaranteeing security and compliance.
The company culture's resistance to change might obstruct the advancement of employees to more advanced stages of maturity. Overcoming this resistance and promoting a climate that is more innovation-driven can be accomplished by promoting open communication, offering sufficient support throughout transitions, and highlighting success stories that emerge from adopting big data.
Organizations may effectively evolve to greater maturity levels in their big data business models by putting these best practices into practice and proactively addressing major obstacles. This will open up new avenues for growth and innovation in a world where data is becoming more and more important.
6. Case Studies on Successful Big Data Adoption
Examining real-world case studies of businesses with varying degrees of maturity is crucial when investigating effective Big Data adoption. Amazon is a well-known leader in using big data for business insights, and it is one such example. Amazon's ability to incorporate data analytics into all facets of their business operations allows them to specialize in marketing, supply chain management, and customer experience personalization. Netflix is an additional noteworthy instance of a company that strategically utilizes Big Data to provide tailored content to its consumers, hence improving customer satisfaction and engagement.😻
Let's move on to businesses with intermediate levels of development. Airbnb is a great example of this. In order to better understand user preferences, customize search results, and improve the overall booking experience, Airbnb has successfully used data analytics. Airbnb accurately forecasts demand trends and optimizes pricing tactics with the application of machine learning algorithms. Walmart is unique in that it uses big data to optimize store layouts, improve product recommendations, expedite inventory management procedures, and boost overall operational efficiency.
A startup called Stitch Fix is a great example of a company embracing Big Data at its infancy. This creative business offers clients individualized styling recommendations by fusing human knowledge with digital data research. Thanks to Stitch Fix's data-driven methodology, they are able to efficiently curate fashion options that are customized to each customer's interests. In a similar vein, Slack makes use of data analytics tools to improve teamwork by offering insights into communication trends and boosting output via astute integrations.
Examining the success stories of these businesses reveals recurring tactics that have facilitated their successful Big Data adoption. First and foremost, one important tactic is to make investments in strong data infrastructure and technologies that facilitate the effective gathering, storing, processing, and analysis of huge datasets. Secondly, it is imperative to cultivate a data-driven culture across the entire organization to guarantee that decision-making procedures are guided by practical insights obtained from data analytics.🧐
Setting data governance and quality as a top priority can help to guarantee that the insights produced are trustworthy and correct. Businesses are better positioned to reap the benefits of their Big Data projects when they set up explicit governance structures and data quality requirements. Encouraging cross-functional teamwork among data scientists, analysts, and business stakeholders creates a synergistic effect when it comes to using Big Data for cross-departmental strategic decision-making.
Businesses must prioritize ongoing education and adaptability by keeping up with new developments in big data analytics and emerging technologies. Organizations may find innovative ways to use data-driven insights to create growth and competitive advantage in today's fast-paced business environment by cultivating a culture of experimentation and innovation.
After putting all of the above into perspective, we can say that studying case studies of businesses at different stages of maturity offers insightful knowledge about the tactics used to successfully implement big data in businesses. By taking note of these practical experiences and adopting important tactics like making technology infrastructure investments, cultivating a data-driven culture, guaranteeing data quality and governance, and encouraging cross-functional cooperation,
and prioritizing continuous learning and adaptation,
companies can enhance their own capabilities in harnessing the power of Big Data to drive innovation,
improve decision-making,
and achieve sustainable growth in an increasingly data-driven world
7. Future Trends in Big Data Business Models
The way businesses handle data-driven initiatives is being revolutionized by a number of emerging trends that will determine the future of big data adoption in organizations. The growing use of artificial intelligence (AI) technologies in Big Data analytics procedures is one notable development. With AI, businesses can handle enormous volumes of data more quickly and effectively while also gaining insightful knowledge and creating predictions from the data, which helps them make better decisions and provide more individualized experiences for their customers.
The rapid growth of Internet of Things (IoT) devices and sensors is another important trend influencing the use of big data in the future. Massive amounts of real-time data are produced by these networked devices, giving businesses previously unheard-of chances to improve operations, expand their product lines, and offer cutting-edge services. Businesses may increase operational efficiency, create new revenue streams, and have a better knowledge of consumer behavior by utilizing IoT data in conjunction with advanced analytics capabilities.
We anticipate a move toward more complex business models that put an emphasis on data-driven insights and agility as companies continue to adopt Big Data technologies and hone their analytics strategies. Businesses that successfully incorporate IoT and AI capabilities into their operations will be better able to anticipate customer wants, adjust to shifting market dynamics, and promote sustainable growth in an environment that is becoming more and more competitive. Big Data, IoT, and AI coming together will open up new avenues for creative business models that enable companies to get the most out of their data assets and remain ahead of the curve in the current digital economy.
8. Benefits of Achieving Higher Maturity Levels
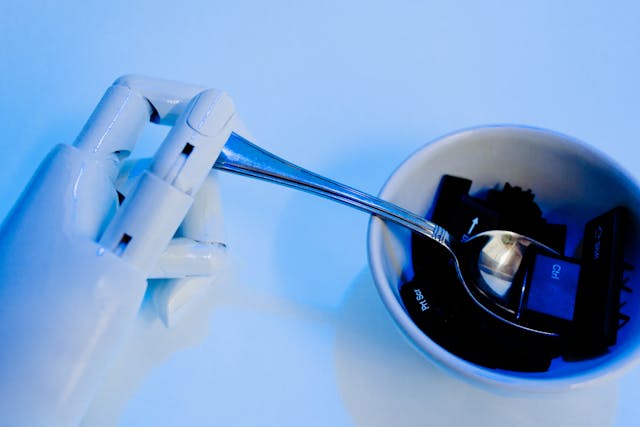
An organization can reap several advantages by advancing its Big Data use. Higher adoption maturity levels of Big Data can lead to better decision-making processes for businesses. This is because enormous volumes of data may be used to gain insights and forecasts that are more precise. Improved operational efficiency and strategic planning can result from such well-informed judgments.
Adoption of advanced big data increases a company's market competitiveness. Businesses that use big data well have a better grasp of their markets, consumers, and trends. With the ability to customize goods and services to fit certain requirements, this knowledge helps businesses establish themselves as leaders in the field.
Innovation inside a company is facilitated by higher adoption maturity levels of big data. Through the examination of data trends and consumer behavior, businesses might find untapped avenues for expansion. This creative thinking can lead to changes in processes, improved products, and total corporate transformation.
From the foregoing, it is clear that moving up the Big Data business model's maturity stages improves decision-making skills while also increasing an organization's competitiveness and fostering creativity. Businesses may remain ahead of the curve in the data-driven business environment of today by embracing big data.
9. Common Pitfalls in Adopting Big Data
Organizations can encounter challenges while implementing big data, such as poor data quality, data silos, ambiguous objectives, and a shortage of trained staff. Prioritizing data quality assurance procedures, integrating data from several sources to reduce silos, defining corporate objectives for big data efforts clearly, and spending money on staff training or recruiting qualified experts are all necessary to prevent these problems. For organizations to successfully manage the challenges of big data adoption, they need establish a culture of constant learning and adaptability.
10. Implementing a Successful Transition Plan
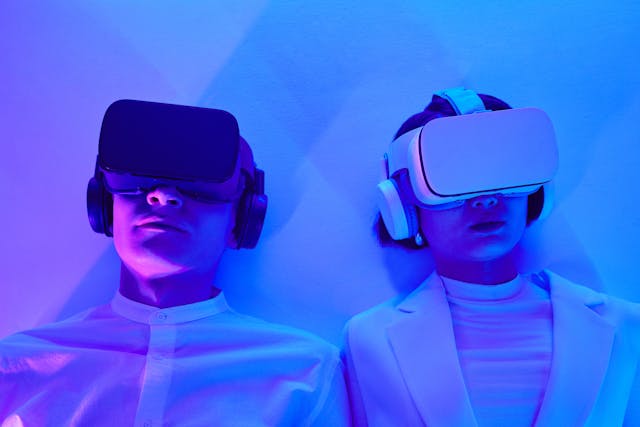
In order to achieve sustained growth and success, a big data business model must transition between several stages of maturity. A planned approach and careful execution are necessary for the successful implementation of a transition plan. In order to properly support this process, think about providing a detailed manual that lists the essential steps required to advance from one maturity level to the next. Detailed instructions for evaluating present capabilities, establishing precise goals for the transition, and determining resource needs can all be found in this booklet.
To ensure a seamless transition, change management tactics are just as important as a well-structured plan. It is imperative to tackle any hurdles or issues that may emerge during the transition process and devise plans to lessen resistance. Maintaining transparent and open channels of communication with stakeholders can assist develop understanding and buy-in for the transition. Communication is crucial when executing changes inside an organization.
Another crucial component of moving between big data business model maturity stages is stakeholder engagement. Early involvement of important stakeholders at various organizational levels can help to better match expectations and goals, which will produce better results. Encouraging stakeholders to actively participate and provide input at every stage of the transition process can guarantee that their viewpoints are taken into account and incorporated into the decision-making process.
Through the provision of a well-organized transition plan, the use of successful change management techniques, and the active involvement of stakeholders, businesses can adeptly traverse varying stages of big data business model maturity, fostering innovation and ongoing progress.
11. Measuring Success: Key Performance Indicators (KPIs)
Establishing Key Performance Indicators (KPIs) is essential for monitoring an organization's use of Big Data as it moves forward. These metrics function as quantifiable numbers that show how well a business is using big data to accomplish its main business goals. Organizations can make well-informed decisions to propel success by developing and tracking targeted key performance indicators (KPIs). This allows for useful performance insights.
By supplying quantitative information on many facets of the implementation process, measuring these KPIs enables organizations to assess how well they are implementing Big Data. KPIs that measure the effectiveness of analytics, data quality, or ROI on Big Data initiatives, for instance, can show if a business is on pace to reach its objectives. By monitoring these measurements over time, businesses can spot opportunities for development and modify their plans accordingly, ensuring that Big Data techniques are successfully implemented.
In summary, setting up pertinent KPIs and regularly monitoring them gives firms a clear roadmap for ongoing development in addition to assisting in tracking their adoption of Big Data. Companies may successfully monitor their performance and make data-driven decisions to drive innovation and growth in today's competitive business landscape by aligning these indicators with strategic objectives.
12. Conclusion
In summary, the Big Data Business Model Maturity Index is a useful instrument for assessing an organization's preparedness for implementing big data strategies. Businesses can determine their level of maturity and pinpoint areas for development by evaluating critical components like leadership, procedures, technology, and culture. To properly leverage the potential of data, firms must be aware of where they are on this index.
It is recommended that readers implement practical measures to augment their organization's data analytics capabilities, drawing from the knowledge gained from the maturity index evaluation. This could entail making investments in cutting-edge analytics tools, educating staff members about data literacy, encouraging a culture that is driven by data from the top down, and enhancing data governance procedures. Through consistent efforts to enhance these domains, entities can fully harness the power of big data and generate significant commercial results.