1. **Introduction**
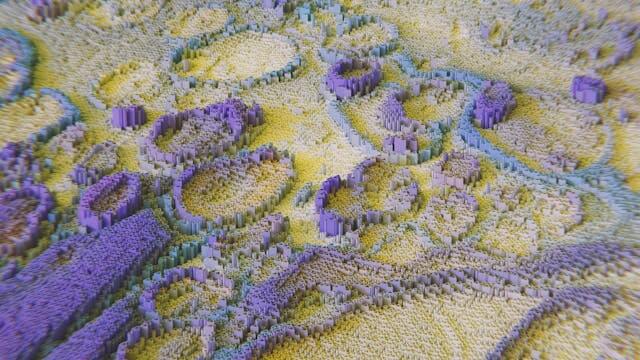
Cloud computing has completely changed the scope of what data scientists can accomplish and how they work in the field. Data scientists can now access enormous quantities of processing power and storage through cloud computing, which makes it possible for them to analyze large data sets more quickly and efficiently. Because of this, data scientists can now execute intricate algorithms, create intricate models, and extract insightful information at a scale that was previously unthinkable. This blog article will examine how data scientists and cloud computing are still connected, and how this relationship is influencing data-driven decision-making in the future.
2. **Evolution of Cloud Computing**
The creation of ARPANET in the 1960s, which laid the foundation for the internet, is when cloud computing began. On the other hand, the phrase "cloud computing" became well-known in the early 2000s. Elastic Compute Cloud (EC2), which Amazon Web Services (AWS) introduced in 2006, was a major factor in the trend towards scalable and adaptable cloud solutions.
With the development of the Infrastructure as a Service (IaaS), Platform as a Service (PaaS), and Software as a Service (SaaS) models, cloud computing developed over time. This development made powerful computer capabilities more accessible to a wider range of enterprises, allowing them to take use of cutting-edge technologies without having to make significant infrastructure investments. Cloud services' cost-effectiveness and scalability have further accelerated their adoption across sectors.
Serverless computing and containerization were made possible by the advancement of cloud computing. These developments reduced application deployment procedures and maximized resource use. In order to meet a variety of business requirements, hybrid and multi-cloud strategies—which integrate on-premises infrastructure with public and private cloud services—have evolved.
Cloud computing's continuous innovation is changing the way businesses handle their data, apps, and IT resources. In addition to giving companies the ability to spearhead digital transformation, this ever-evolving technological landscape offers data scientists fresh chances to glean insightful information from massive volumes of data kept in diverse cloud settings.
3. **Role of Data Scientists in Cloud Computing**
Data scientists are essential to making the most of cloud computing's potential. Their proficiency in examining extensive datasets enables enterprises to derive significant insights from cloud-based data. Data scientists are able to extract actionable insight that powers organizational decision-making processes through the use of machine learning algorithms and advanced analytics.📉
Data scientists are essential to the design and implementation of scalable data solutions in the field of cloud computing. They provide reliable designs that enable easy access to information, effectively manage big datasets, and optimize data storage. In cloud environments, data scientists are essential to maintaining data security and governance and reducing the dangers connected with sensitive data kept on distant servers.
At the vanguard of cloud technology innovation are data scientists. By utilizing their expertise in statistical analysis, AI-driven apps, and predictive modeling, they help organizations seize fresh chances for expansion and a competitive edge. Together with cloud engineers, data scientists create complex solutions that boost productivity, streamline workflows, and propel industry-wide digital transformation projects.
Cloud computing and data scientists work together in a symbiotic relationship where data scientists apply their analytical skills to extract valuable insights that drive business success, while cloud technology provide the infrastructure and scalability required to analyze massive volumes of data. This continued relationship emphasizes how important data scientists are to helping businesses all over the world realize the full benefits of cloud computing.
4. **Challenges Faced by Data Scientists in Cloud Computing**
When working with cloud computing, data scientists encounter a number of difficulties that may affect their productivity and output. The difficulty of managing massive datasets across dispersed cloud storage systems is one major problem. In order to maximize performance, complex solutions are needed to prevent processing delays and inefficiencies caused by data movement and synchronization between various components.
Ensuring data security and regulatory compliance while storing and processing sensitive data on cloud systems presents a considerable challenge. The complexity of data scientists' tasks is increased by the need to manage encryption techniques, access controls, and privacy policies to preserve data integrity and comply with legal obligations. 📖
For data scientists, managing cloud resource costs is an ongoing concern. A thorough understanding of the price structures provided by different cloud providers, resource allocation techniques, and diligent monitoring are necessary to balance the use of processing power, storage, and other services to control costs while fulfilling project needs. While underestimating resource requirements may cause problems or delays in performance, overestimating might result in wasteful spending.
It is difficult for data scientists to stay current with new tools, capabilities, and best practices due to the cloud's rapid expansion. To effectively harness the latest innovations and improve efficiency in cloud-based data analysis workflows, a constant state of learning and adaptation is required.
Furthermore, as I mentioned above, data scientists who operate in cloud environments face a variety of complex issues pertaining to cost optimization, security, data management, and technical improvements. To overcome these obstacles and maximize productivity in analytics projects that use cloud infrastructure, a mix of core knowledge in cloud computing and data science is required. Keep an eye out for the upcoming section where we'll talk about practical techniques to overcome these challenges.
5. **Benefits of Cloud Computing for Data Scientists**
For data scientists, cloud computing offers a plethora of advantages that transform their work processes and make them more productive in their endeavors. Scalability is a major benefit of cloud platforms; data scientists may simply increase or decrease their computing power according to project demands. Because of this flexibility, they may acquire the required processing power without being constrained by the infrastructure on-premises. 👶
Enhanced productivity and teamwork are two other important advantages of cloud computing for data scientists. Regardless of where team members are physically located, cloud-based solutions enable smooth collaboration. Data scientists may collaborate in real time on projects, improving workflows and communication. Data scientists can expedite their efforts by utilizing a plethora of pre-built machine learning models and resources offered by cloud platforms.
Cost-effective options for processing and storing data are provided by cloud computing. Pay-as-you-go pricing approaches allow data scientists to only pay for the resources they really use, removing the need for large upfront hardware purchases. In the end, this economical method democratizes access to sophisticated computing powers by allowing data scientists to experiment with big datasets and intricate algorithms without worrying about infrastructure expenses.
For data scientists, cloud computing offers benefits like cost-effectiveness, scalability, and collaboration along with improved data security. Prominent cloud service companies make significant investments in strong security protocols to safeguard private information kept on their systems. Data scientists may concentrate on deriving insights from their data without worrying about any breaches or vulnerabilities by utilizing these secure settings.
For data scientists, cloud computing offers profound and revolutionary advantages. Data scientists may drive innovation and advancement in the field of data science by utilizing the cloud to uncover new possibilities in their research and analytical processes.
6. **Case Studies: How Leading Data Scientists Leverage Cloud Computing**
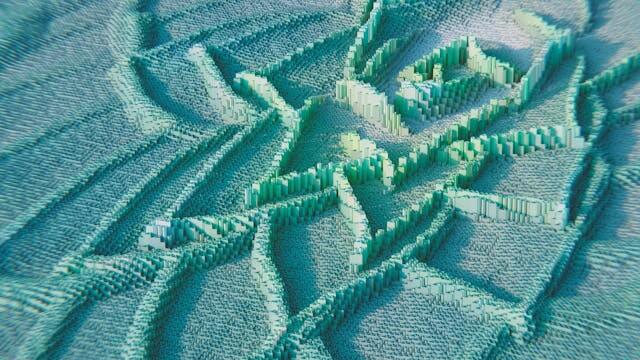
Case Studies provide insightful information about how leading data scientists use cloud computing to improve workflows. For example, well-known data scientist DJ Patil uses cloud platforms to analyze large datasets and extract useful information. His ability to solve complicated issues quickly and easily thanks to the use of scalable cloud resources highlights the value of cloud computing in contemporary data science techniques.
The work of well-known data science expert Hilary Mason is another excellent case example. Mason shows how she can experiment with machine learning models at scale without worrying about infrastructure limitations by utilizing cloud computing services. She can swiftly iterate on different methods and methodologies because to her versatility, which helps her push the boundaries of innovation in the data science sector.
Grandmaster Abhishek Thakur of Kaggle demonstrates how to strategically employ cloud computing to conduct demanding model training jobs. He can train complex models on large datasets in a fraction of the time it would take to use conventional on-premises solutions by effectively utilizing cloud resources. This example shows how cloud computing enables data scientists to maximize resource utilization and speed up their R&D operations.
These case studies highlight how crucial cloud computing is to enabling top data scientists to push the envelope of what's practical in their industry. These specialists are able to scale their operations, optimize performance, and drive innovation at a pace that was previously unattainable with traditional approaches because they are adept at adopting cloud technology. 😉
7. **Future Trends: The Intersection of Cloud Computing and Data Science**
Prospective developments in cloud computing and data science indicate a growing convergence of these two domains. A number of trends are expected to change the way data scientists use cloud technologies to improve analysis and insights in the near future.
The increasing integration of machine learning (ML) and artificial intelligence (AI) capabilities into cloud platforms is a significant development that lies ahead. With the help of this integration, data scientists will be able to process enormous volumes of data more quickly and effectively, leading to the extraction of insightful knowledge.
It is anticipated that the emergence of serverless computing will change the way data scientists approach the creation and application of models. Data scientists can boost productivity and workflow agility by focusing more on analysis and less on managing infrastructure by utilizing serverless architectures in the cloud.
The democratization of data science through cloud-based platforms and tools is another noteworthy trend. Aspiring data scientists from a range of backgrounds may now more easily undertake difficult analyses and create novel solutions thanks to the cloud's pre-built machine learning models and scalable computing capabilities.
The future is essentially promising a dynamic landscape in which cloud computing will work as a catalyst for data science innovation, allowing experts to push boundaries, investigate new areas of knowledge, and effect meaningful change across industries.
8. **Security Concerns in Cloud Computing for Data Scientists**
When using cloud computing services, data scientists must ensure data security. To protect the integrity and confidentiality of the data, it's critical to address a number of security issues while working with sensitive data on cloud platforms.
One essential technique that can safeguard data whether it's in transit or at rest is data encryption. Data scientists may stop unwanted access to their priceless data assets by encrypting sensitive information before storing it in the cloud and making sure safe communication channels are established during data transfers.
In cloud systems, access control measures are essential for preserving data security. Robust authentication and authorization mechanisms should be implemented by data scientists to manage who has access to particular cloud-stored datasets or resources. This aids in preventing sensitive data from being altered or extracted by unauthorized users.
To identify any suspicious activity or unauthorized access attempts, cloud operations must be routinely monitored and audited. Cloud service providers offer logging and monitoring tools that data scientists should use to monitor interactions with their data and quickly address any possible security concerns.
When working in cloud environments, data scientists must stay up to date on the newest security threats and best practices. Through regular updates on developing cyber dangers and implementation of industry-standard security measures, they may enhance data protection and guarantee adherence to pertinent requirements.
After reviewing the material above, we can say that although cloud computing presents enormous advantages for data scientists, security issues must be addressed in order to reduce the dangers involved in managing sensitive data. Data scientists may create a safe working environment in the cloud by putting strong security measures in place, such as monitoring, access control, encryption, and staying up to date on cybersecurity developments.
9. **Training and Skills Development for Data Scientists in Cloud Computing**
To succeed in a world where cloud computing is king, data scientists must invest in training and skill development. It's crucial to comprehend cloud computing systems like AWS, Azure, and Google Cloud. It will also be beneficial to have experience with big data tools like Spark or Hadoop and programming languages like R or Python. To fully utilize cloud computing for the effective analysis and interpretation of large datasets, data scientists should gain a solid understanding of cloud-based databases, management services, and data warehousing.
Upskilling and constant learning are essential for data scientists who want to succeed in the cloud computing industry. To stay up to date with industry developments and best practices, professionals can benefit from training programs that concentrate on tools, platforms, and technologies relevant to the cloud. Improving practical skills requires working on real-world projects involving machine learning and cloud-based analytics. This requires hands-on experience. Within enterprises, working with cross-functional teams can help refine critical soft skills like project management, communication, and problem-solving.
Data scientists should develop a mindset that is oriented on creativity, adaptability, and continuous development in a dynamic cloud computing environment in addition to their technical knowledge. Professionals may stay adaptable and responsive to changing business needs by adopting a proactive approach to learning about and exploring emerging trends in cloud technologies. Through conferences, webinars, or online groups, data science professionals can network with others in the field and gain insightful information that can lead to chances for collaboration and skill development.
Developing a comprehensive skill set that includes both technical expertise in cloud computing tools and soft skills like creativity, collaboration, and critical thinking is essential for data scientists who want to play a major role in effectively utilizing cloud resources for advanced analytics projects. Through the prioritization of continuous training programs designed to meet the demands of the ever changing world of cloud computing, data scientists may establish themselves as valuable strategic resources capable of extracting meaningful insights from large, complicated datasets using state-of-the-art technology found on cloud platforms.
An exciting paradigm change is occurring at the convergence of data science and cloud computing, which is changing how businesses use data to spur innovation and gain a competitive edge. With more companies shifting their analytics requirements to cloud-native architectures, there is an increasing need for qualified data scientists who understand how to use cloud technologies. Through the provision of scalable compute resources by top cloud providers, data scientists can unlock the transformative potential of advanced analytics and improve their career prospects by investing in ongoing training and skill development tailored to the intricacies of working with big data on the cloud.🫠
10. **Best Practices: Optimizing Data Science Workflows on the Cloud**
A number of best practices are involved in cloud-optimized data science workflows that increase productivity and efficiency. First off, utilizing cloud-native services and tools for data processing, analysis, and storage can greatly expedite work. Data scientists can concentrate more on drawing conclusions than on maintaining infrastructure by using managed services like Google BigQuery for large-scale dataset querying and Amazon S3 for storage.
Second, automating complicated procedures is made easier by putting in place appropriate data pipeline orchestration technologies like Apache Airflow or Amazon Managed procedures for Apache Airflow. This guarantees effective management of dependencies, efficient process execution, and suitable task scheduling without the need for human interaction.
Using cloud platforms such as Microsoft Azure or AWS to adopt scalable compute resources enables variable provisioning of processing capacity according to workload demands. By automatically modifying resources to match the requirements of data processing jobs in real-time, auto-scaling capabilities ensure cost-effectiveness.
Workflow efficiency can be increased by optimizing storage settings and selecting the right types, such as block or object storage, based on performance needs and access patterns. Compression algorithms, indexing strategies, and data partitioning are also essential for improving query performance and cutting down on latency in data processing pipelines.
Using containerization technologies such as Docker or Kubernetes makes data science workflows more portable and reproducible in various cloud environments. Code packaging, when combined with its dependencies, guarantees consistency in output, streamlines deployment procedures, and facilitates teamwork.
To sum up everything I've written thus far, data scientists may streamline their workflows to provide insights more quickly, simplify operations, and spur innovation inside their companies by adhering to these best practices and making good use of cloud computing services.
11. **Collaboration between Data Scientists and Cloud Service Providers**
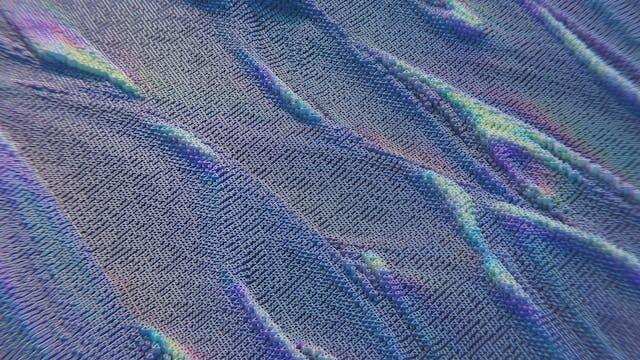
To maximize project success, cloud service providers and data scientists must work together. To effectively analyze massive amounts of data, data scientists rely on the scalability, storage, and processing capacity of cloud platforms. Through tight collaboration with cloud service providers, data scientists can take advantage of cutting-edge tools and technology to perform sophisticated data processing.
Cloud-based machine learning services are one way cloud providers and data scientists work together. These services facilitate the creation of predictive models by providing pre-built models and frameworks. Data scientists can accelerate innovation and model deployment by concentrating more of their attention on optimizing algorithms rather than infrastructure management.
Cloud service companies offers affordable ways to handle and store big datasets. Working together, data scientists may select the best cloud services for their projects' needs and optimize resource allocation. Data scientists are guaranteed to be informed about any new features and advancements that can enhance their analytical work through ongoing connection with cloud providers.
The collaboration of cloud service providers and data scientists can facilitate effective data analysis, expand the availability of computing power, and easily incorporate state-of-the-art technology into projects. Both sides can improve their capacities and spur innovation in the data science and cloud computing domains by cultivating this relationship.
12. **Conclusion: The Ever-Evolving Relationship Between Cloud Computing and Data Scientists**
After a summary of the material presented, we can say that innovation in the IT sector depends on the symbiotic relationship that exists between data scientists and cloud computing. Cloud platforms' accessibility, scalability, and flexibility have enabled data scientists to quickly and effectively evaluate enormous volumes of data and extract insightful information. In exchange, data scientists push for improvements that address their changing needs, which propels the development of cloud technologies.
This relationship will only get stronger as data volumes increase dramatically and technology develops. To properly address complicated issues, data scientists will need more sophisticated cloud tools, and cloud service providers will keep giving top priority to features that facilitate data-intensive jobs. The continued cooperation and adaptability between these two fields emphasizes how important it is to work together to shape technology's future.
The partnership of data scientists and cloud computing opens the door to revolutionary discoveries and game-changing solutions in a variety of industries. We may anticipate more developments that completely change the way we approach data analysis, machine learning, and AI-driven inventions as these two domains continue to develop together. In today's digital landscape, firms looking to maintain their competitiveness and maximize the value of their data assets must embrace this synergy.