1. Introduction
Big Data has been a game-changer in a number of industries throughout the digital transformation period, changing how businesses function and make decisions. Large-scale information must be gathered, processed, and analyzed in order to gain insightful knowledge and enhance decision-making. This is known as big data. Big Data is changing conventional methods and creating new avenues for innovation in the banking, finance, and credit sectors.
Big Data is essential to the banking, financial, and credit industries because it gives these businesses a better understanding of the risks, preferences, and behaviors of their customers. Financial organizations can generate more accurate predictions, evaluate creditworthiness more efficiently, identify fraud proactively, and customize services for specific clients if they have the capacity to examine massive datasets in real-time. Banks may improve client experiences by using Big Data analytics to power focused marketing campaigns and specially designed products that cater to niche markets.
By examining patterns and trends in enormous datasets, big data gives financial organizations the ability to spot possible problems early on in the context of risk management and compliance. By taking a proactive stance, banks may successfully manage risks, maintain regulatory compliance, and prevent fraudulent activity. Institutions can improve overall operational efficiency, forecast market swings, and optimize investment strategies with the predictive powers made possible by Big Data analytics.
Taking into account everything mentioned above, we can say that the application of big data analytics to the banking, finance, and credit sectors is revolutionizing conventional business models by offering previously unheard-of chances for data-driven decision-making. Organizations may create better value propositions to customers and obtain a competitive edge in today's dynamic marketplace by leveraging the power of big data technologies. Financial institutions that want to stay ahead of the curve and meet the difficulties of an increasingly complex digital landscape must embrace this data-driven mindset.
2. Big Data Applications in Banking
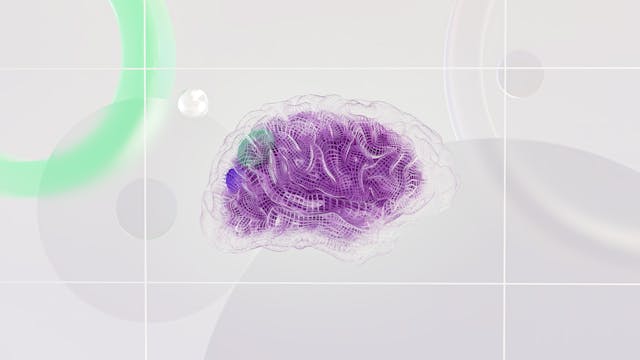
Big data is transforming the banking industry in a number of ways, including bettering fraud detection and risk assessment as well as client experiences. Banks may provide clients with individualized experiences by using big data analytics to create financial products and services that are tailored to their tastes and behavior patterns. This promotes enduring loyalty in addition to raising client happiness.
Big data is essential to risk assessment because it allows institutions to evaluate enormous volumes of data instantly, seeing any problems before they become more serious. Financial institutions can improve their fraud detection skills, identifying fraudulent actions quickly and protecting consumer assets, by utilizing advanced algorithms and machine learning approaches. By taking a proactive approach to risk management, banks are able to reduce possible losses and uphold client trust.
Big data applications are being used in banking to improve decision-making and expedite operations. Financial organizations can obtain important insights into consumer behavior, market trends, and possible hazards by utilizing big data analytics. With this tactical edge, banks can respond swiftly to changing market conditions and increase productivity and profitability by making well-informed decisions.
3. Big Data Impact on Financial Markets
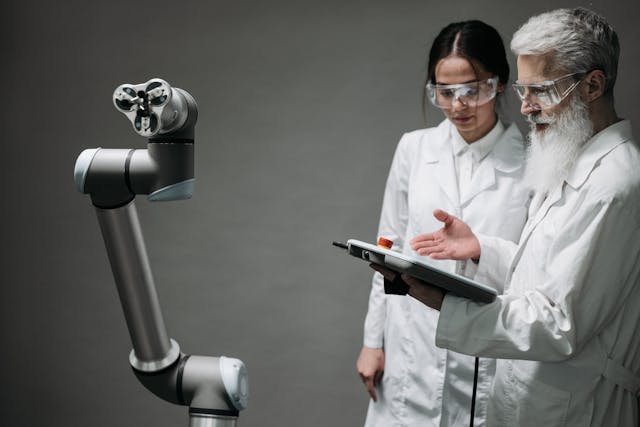
Big Data is improving algorithmic trading and market analysis, which is transforming the financial markets. Financial organizations may use complex algorithms to evaluate market trends, forecast stock values, and make remarkably accurate split-second trading decisions because to the massive amounts of data that are generated every second. Because of this automation, trading processes are now much faster and more efficient, enabling quick reactions to changes in the market.
Big Data-driven predictive analytics is revolutionizing financial industry investing choices. Predictive analytics systems are capable of making more accurate predictions about possible outcomes and hazards than ever before by utilizing historical data, investor behavior patterns, and market trends. As a result, investors are better equipped to minimize risk and optimize investment strategies for higher returns by making well-informed decisions based on data-driven insights.
In summary, the incorporation of Big Data into financial markets is transforming the way that trading occurs and decisions about investments are made. In the fast-paced world of finance, the application of sophisticated algorithms and predictive analytics has not only increased productivity but also made it possible to make better decisions. The financial sector is rapidly going digital, and in the future, data-driven insights will be crucial in determining investment plans and market dynamics.
4. Utilizing Big Data for Credit Scoring
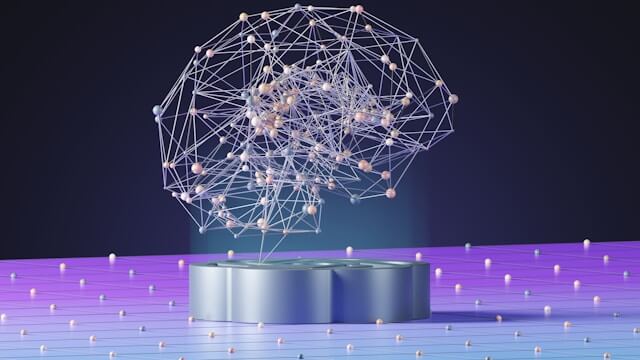
The assessment of a person's creditworthiness has been transformed by the use of big data in credit scoring. Financial companies can increase their accuracy in determining a borrower's ability to repay a loan by utilizing large data sets. Conventional credit scoring methods frequently depend on restricted historical financial data, whereas Big Data enables a more thorough and dynamic analysis that takes into account a greater number of variables.
The use of non-traditional data sources is a major benefit of utilizing Big Data in credit assessment. In addition to standard techniques like income and credit histories, lenders can also access non-traditional data such as utility payment records, social media activity, and even GPS information from cellphones. With a more comprehensive understanding of a person's financial behavior and risk profile provided by this multidimensional approach, credit assessments can be more accurate and nuanced.
Banks and other financial organizations can lower the risk of defaults while improving lending decisions by utilizing Big Data analytics in credit scoring. An individual's financial situation and ability to repay debt are more accurately reflected when a variety of data sources are taken into account. This move toward using big data benefits lenders by lowering risk, and it also gives previously disadvantaged people access to financial products that are customized to meet their unique needs.
5. Challenges and Ethical Considerations in Big Data Usage
The banking sector is using big data more and more, which has brought up a number of issues and ethical questions. When managing enormous volumes of sensitive data, privacy and data security concerns are crucial. Financial institutions are required to maintain compliance with rules such as the CCPA and GDPR and to implement stringent safeguards to protect consumer data from breaches and unauthorized access.
The possibility of prejudice and unfairness in algorithmic decision-making processes driven by big data is another crucial factor to take into account. In domains like credit scoring or loan approvals, algorithms have the potential to inadvertently reinforce preexisting biases or perpetuate discriminatory practices. It is imperative that banks and other financial institutions conduct routine audits of their algorithms, deal with concerns of bias, and guarantee equity and openness in the decision-making process. To stop biased algorithms powered by big data analysis from having negative effects on people or marginalized communities, ethical norms must be set.
6. Future Trends in Big Data's Influence on Banking, Finance, and Credit
We may anticipate much more AI integration in the credit, banking, and finance sectors in the future. AI technology will be essential in enabling deeper insights into consumer behavior and industry trends. Financial institutions will be able to automate tasks like fraud detection, risk assessment, and customized client care with AI algorithms and machine learning.
The adjustment of legal frameworks to keep up with technical improvements in big data analytics is another important trend that is likely to emerge. In light of these new technologies, regulators are realizing that data privacy, security, and transparency regulations need to be updated. Realizing the full potential of big data in a regulated setting would require striking a balance between innovation and compliance.
7. Case Studies: Successful Implementations of Big Data in Financial Services
Big data analytics has changed the game for many financial services organizations looking to improve their customer experience and operational efficiency. Let's examine a few case studies that highlight effective big data applications in credit, banking, and finance.
1. **JP Morgan Chase**: One of the biggest banks in the world, JP Morgan Chase uses big data to detect fraud, enhance risk management, and provide clients with customized offers. Through real-time analysis of massive volumes of transaction data, JP Morgan is able to anticipate possible threats and protect its customers' banking experience.
2. **Ant Financial Services Group (Alibaba)**: Through its platform Alipay, Ant Financial, a unit of Alibaba Group, is revolutionizing financial services by leveraging big data. Ant Financial offers millions of customers personalized financial solutions like microloans and investment opportunities by leveraging client data such as social interactions, payment history, and purchasing preferences. This has shaped the development of digital banking in China.
3. **Capital One**: Capital One uses big data technology to accurately identify credit risks and provide customized financial solutions. Capital One is a pioneer in using data analytics to drive decision-making processes. Capital One consistently optimizes its processes to be competitive in the ever-changing financial business while personalizing offers for consumers with predictive analytics and machine learning algorithms.📖
4. **American Express**: American Express uses big data analytics to learn about the preferences and spending habits of its customers. Through the examination of transactional data in conjunction with outside sources like as social media patterns and economic factors, Amex creates creative loyalty programs, quickly identifies fraudulent activity, and improves customer satisfaction by effectively anticipating their needs.
5. **PayPal**: PayPal's clever application of big data analytics is largely responsible for its success in the digital payment market. PayPal offers millions of customers worldwide secure transactions and personalized suggestions based on individual preferences, all while preventing fraud in real-time by utilizing machine learning algorithms to evaluate transactional behavior patterns.
These illustrations show how big data has revolutionized traditional banking and financial services by empowering businesses to promptly make well-informed decisions, efficiently manage risks, tailor services to individual clients, and maintain an advantage over rivals in a constantly changing market. Through encouraging innovation, increasing operational effectiveness, and eventually improving the entire customer experience across numerous industrial sectors, the incorporation of big data analytics continues to change the future of finance.
8. The Role of Machine Learning in Transforming Financial Services
By empowering banks and other financial institutions to make better informed decisions, machine learning has completely changed the financial services sector. Fraud detection is one important area where machine learning is having a big influence. Banks are analyzing massive volumes of transaction data in real-time and looking for trends that point to possible fraudulent behavior using machine learning algorithms.
Credit scoring is a crucial area of application for machine learning in finance. Accurately determining an individual's creditworthiness is typically hampered by traditional credit scoring algorithms, particularly for people with short credit histories. To create more accurate credit assessments, machine learning models can examine data from other sources, like social media activity, online buying habits, and even usage patterns of smartphones.
Personalizing client experiences in banking is another application of machine learning. Banks are able to provide customized product suggestions, focused marketing efforts, and proactive customer care by examining consumer data and behavior. In addition to raising consumer pleasure, this degree of customisation also helps to boost loyalty and retention rates.
In financial organizations, machine learning is essential for automating procedures and cutting operating expenses. Machine learning algorithms can be used to expedite processes like document verification, risk assessment, and regulatory compliance, giving people more time to work on more difficult jobs that call for human judgment.
In banking and finance, machine learning technologies are being used at a rate that is increasing efficiency, optimizing decision-making, improving consumer experiences, and eventually changing the way financial services are provided.
9. Benefits and Risks Associated with Utilizing Big Data Analytics
There are several benefits to using big data analytics in banking, finance, and credit. An important advantage is the increased effectiveness of decision-making procedures. Financial organizations can make better decisions in real-time, more tailored client experiences, more effective risk management, and more focused marketing campaigns by quickly evaluating large amounts of data.
These advantages do, however, come with hazards, which enterprises must carefully manage. When handling significant amounts of sensitive data, data breaches are a serious worry. The greater the volume of data gathered and examined, the greater the probability of being targeted by cybercriminals who want to take advantage of weaknesses in systems. For businesses using big data analytics in the financial industry, safeguarding this data has emerged as a critical concern.
In today's digital world, success requires striking the correct balance between leveraging big data for innovation and reducing the dangers involved. To securely utilize the full potential of big data analytics, a business must implement strong cybersecurity safeguards, adhere to laws like GDPR and PCI DSS, and cultivate a culture of data protection. In order to protect important data assets and take advantage of the benefits that big data analytics can provide, businesses must continue to be watchful and proactive.
10. The Evolution of Risk Management with Big Data
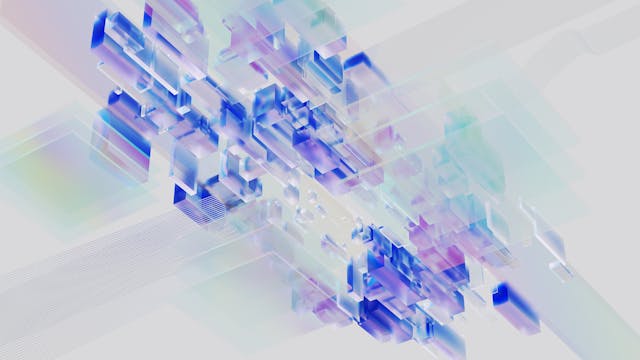
Big data has revolutionized traditional risk assessment approaches and changed risk management in the banking and finance industry. Financial institutions may now more correctly identify risks by analyzing consumer behavior, market trends, and economic indicators in real time thanks to their access to massive volumes of data. This helps banks to anticipate hazards and take proactive steps to reduce them before they become more serious.
Banks can improve their credit scoring algorithms by adding more variables than just traditional financial measures by utilizing big data analytics. Social media usage, internet purchasing habits, and even geolocation data can reveal important information on a person's creditworthiness. Because of this, banks are able to provide customized loan packages that are suited to the particular needs of each consumer, resulting in higher approval rates and lower default risks.
Banks can identify fraudulent activity more successfully thanks to big data, which flags questionable transactions based on anomalies found by computers that recognize patterns. Financial institutions can quickly respond to possible security issues and shield their clients from fraudulent schemes or illegal access using real-time dataset analysis. In addition to protecting the bank's assets, this proactive strategy increases client loyalty and trust.
Taking into account everything mentioned above, we can draw the conclusion that the development of big data and risk management has enabled banks and other financial organizations to make better judgments, customize their goods and services to meet the demands of their clients, and proactively reduce risks before they become more serious. Customers all across the world will be able to enjoy a more safe, effective, and customized financial experience thanks to the banking industry's embrace of big data analytics.
11. The Future Prospects: Innovations Driven by Big Data
In the realm of banking, finance, and credit, the future is being dramatically reshaped by the relentless progress of big data technologies. Emerging innovations are poised to revolutionize these industries in profound ways.
Big data analytics-driven innovations in banking and finance are poised to unleash hitherto unattainable levels of effectiveness, precision, and individualized service. Institutions will be able to reduce risks, improve consumer behavior understanding, and expedite operations through the application of artificial intelligence (AI), machine learning, and predictive analytics. In addition,
Blockchain technology is transforming transactions by offering transparent and safe procedures that allow for quicker payments at a lower cost. By producing an unchangeable record of every transaction, this decentralized system fosters greater confidence amongst participants. In addition,
Financial services are becoming more easily integrated into daily life because to the Internet of Things (IoT). Financial institutions may now more effectively customize services to meet the demands of individual customers because to the massive volumes of data that linked devices are collecting on consumer behaviors and preferences. In addition,
In essence, the evolving landscape influenced by big data promises a future where banking, finance, and credit are more inclusive, accessible, and customer-centric than ever before.
12. Strategies to Harness the Power of Big Data Effectively
To effectively leverage the power of big data for competitive advantage in banking, finance, and credit sectors, businesses can implement several key strategies:
1. **Data Integration**: To obtain a comprehensive understanding of customers and make better decisions, integrate data from several sources such as customer transactions, social media interactions, and market trends.
2. **Advanced Analytics**: To increase risk management and provide individualized services, leverage advanced analytics methods like machine learning and predictive analytics to glean insightful information from huge datasets.
3. **Real-time Monitoring**: Implement real-time monitoring systems that can instantly detect anomalies or patterns, enabling proactive responses to potential risks or opportunities.
4. **Enhanced Security Measures**: Make use of big data technology to safeguard confidential financial information and guarantee regulatory compliance while investing in strong cybersecurity measures.
5. **Customer Segmentation**: Create customized financial products and targeted marketing efforts by using big data analytics to segment clients based on their risk profiles, preferences, and behavior.
Organizations may use big data to spur innovation, increase operational effectiveness, and gain a competitive edge in the ever-changing banking, finance, and credit sectors by implementing these methods into their daily operations.