1. Introduction
Thanks to machine learning (ML), computers can now learn from experience and become better at what they do without needing explicit programming, which has changed a number of fields. ML algorithms examine data to find trends, forecast outcomes, and offer insightful information in a variety of domains, including marketing, finance, healthcare, and more. The combination of machine learning and microbiology offers promising opportunities to advance research in this important field of science.
Machine learning is being applied in the field of microbiology to provide new and efficient methods for quickly and accurately analyzing large amounts of biological data. Machine learning (ML) techniques like deep learning and neural networks are changing the way we approach microbiological research. These techniques can be used to discover unknown species of bacteria, anticipate patterns of antibiotic resistance, or analyze complicated microbial relationships. This technology not only simplifies data analysis procedures but also creates new opportunities for improving diagnostics, developing new treatments, and deciphering the workings of microbial ecosystems.
2. Importance of Machine Learning in Microbiology
Because machine learning provides strong tools to handle and interpret complicated biological data, it is essential to the advancement of microbiology research and analysis. ML algorithms can effectively analyze the massive amounts of data generated in microbiology by genome sequencing, metagenomics, and other high-throughput techniques. These algorithms can reveal patterns, relationships, and hidden information that may be difficult to uncover using traditional methods. This capability makes it possible for researchers to more accurately and quickly get deeper insights into the relationships, communities, and activities of microbes.
The use of machine learning (ML) techniques in microbiology has several advantages, including better illness diagnosis by the creation of predictive models based on microbial signatures or genetic markers. Scientists can improve the accuracy and efficiency of detecting pathogenic bacteria or mechanisms of antibiotic resistance in clinical samples by utilizing machine learning (ML) algorithms. This can lead to more focused treatment plans and improved patient outcomes. Through the analysis of large-scale genomic and proteomic data sets, machine learning makes it easier to anticipate novel therapeutic targets, antimicrobial compounds, or biotechnologically relevant enzymes. These forecasts have the potential to quicken the process of finding new drugs and encourage the creation of creative treatments for infectious illnesses.
Researchers can now understand complex microbial communities and their dynamics in a variety of settings, including soil, the ocean, and human bodies, thanks to machine learning. Scientists can uncover biological interactions between microorganisms, identify keystone species controlling community structure, or forecast reactions to environmental changes by using clustering algorithms or network analysis techniques on microbiome data sets. Understanding microbial diversity patterns, ecosystem resilience mechanisms, and possible applications in bioremediation, agriculture, or tailored medicine all benefit greatly from these knowledge. In summary, the use of machine learning in microbiology is revolutionizing research methods and creating new opportunities for discoveries with significant consequences for environmental sustainability and human health.
3. Types of Machine Learning Models Used in Microbiology
In the field of microbiology, various types of machine learning models are applied to analyze and interpret complex microbial data.
1. **Supervised Learning**: Models are trained on labeled data using supervised learning, which enables them to classify or predict depending on input features. By learning from labeled datasets including microbial features, this kind of model can help in the classification of various bacteria in the field of microbiology.
2. **Unsupervised Learning**: In the absence of labeled data, unsupervised learning is useful for finding patterns and structures in microbiological data. When grouping microorganisms according to shared phenotypic or genetic traits, this model can be useful.
3. **Reinforcement Learning**: This technique teaches models how to choose actions in a way that maximizes the sum of their rewards. This model could be used in microbiology to guide the selection of molecules with antibacterial properties through virtual screening approaches, hence optimizing processes like drug discovery.
4. **Deep Learning**: Neural networks in particular, or deep learning models, are adept at handling high-dimensional data, such as genetic sequences or extensive omics datasets used in microbiology research. When used for tasks like genome annotation or metagenomic research, these models are highly effective in identifying complex patterns and correlations within microbial data.
5. **Transfer Learning**: This technique uses the information from a model that has already been trained on one task to enhance performance on a related task that has less labeled data. By using pre-trained models for tasks like protein function prediction or antibiotic resistance detection, transfer learning in microbiology helps expedite research.
6. **Bayesian Models** : Bayesian models are probabilistic frameworks that can deal with uncertainty in microbial studies, such as predicting evolutionary trajectories or analyzing ecological interactions within microbial communities. They can incorporate prior knowledge and update beliefs based on new evidence.
Every kind of machine learning model has special benefits that address many facets of microbiology research, showcasing its adaptability and potential to improve our knowledge of microbial systems globally.
4. Application of Machine Learning in Disease Detection
Because machine learning (ML) algorithms allow for the early identification and diagnosis of microbial diseases, they have completely changed the discipline of microbiology's approach to disease detection. Large data sets can be analyzed by these algorithms to find patterns and signs that are occasionally invisible to human observers. The capacity of machine learning (ML) to handle massive datasets fast and accurately, resulting in prompt intervention and treatment, is a major benefit when it comes to disease identification.
Numerous case studies demonstrate the effective use of machine learning in microbiology for illness identification. For example, by examining genetic data, scientists have used ML algorithms to identify bacterial strains resistant to antibiotics. With this strategy, medical practitioners can anticipate possible epidemics and modify patient care accordingly, leading to better patient outcomes and a slowdown in the spread of illnesses resistant to drugs.
Another application of machine learning (ML) is the identification of certain biomarkers linked to infectious diseases like influenza or tuberculosis. Through the examination of diverse biological specimens, machine learning algorithms are able to distinguish between distinct pathogens and forecast the probability of infection by taking into account many variables. With early intervention, this tailored approach improves diagnostic accuracy and expedites the start of relevant therapy, eventually saving lives.
The application of machine learning to microbiology's illness identification presents a possible path toward more effective and efficient medical procedures. By employing powerful algorithms to scan through complicated data sets, researchers and healthcare professionals can stay one step ahead in diagnosing and combating microbial illnesses before they progress into greater health concerns.
5. Predictive Analysis Using Machine Learning in Microbial Ecology
New pathways for studying microbial interactions and ecosystems have been made possible by predictive analysis in microbial ecology utilizing machine learning (ML). Researchers can learn more about the intricate relationships that exist among microbial communities by utilizing predictive modeling techniques. With the aid of machine learning algorithms, scientists can predict how various microorganisms will interact and affect their surroundings by analyzing large datasets and finding patterns that may not be seen using more conventional analytical techniques. 👥
Predicting alterations in the architecture of microbial communities is a crucial use of machine learning in microbial ecology. Through model training using past data on microbial compositions in different settings, scientists can forecast potential changes in these communities over time or in reaction to outside influences. Through these projections, scientists may better anticipate changes in ecosystem dynamics and develop strategies for managing microbiomes in a variety of environments, including soil, oceans, and human bodies.
ML is essential to improving our comprehension of microbial populations and their complex interactions. Researchers can better understand microbial ecosystems and enable more informed decision-making in microbiology and related domains by utilizing predictive analysis.
6. Drug Discovery and Development with Machine Learning
The use of machine learning in the field of microbiology has completely changed the methods used in drug discovery and development. The detection of compounds' possible antibacterial capabilities has been greatly accelerated by machine learning techniques, leading to a more effective screening process for new antibiotics. ML models may predict which chemicals are likely to have antimicrobial properties by examining massive databases of chemical structures and their biological activities. This reduces the time and resources needed for traditional experimental procedures.
Novel antibiotics from natural sources have been identified in large part thanks to machine learning. Through the use of algorithms that have been trained on massive volumes of data from many sources, including chemical databases, environmental samples, and microbial genomes, researchers may sort through this data and choose candidates that show promise for additional investigation. By applying this technology, compounds with special antibacterial qualities that might not have been found using traditional screening techniques can be found. Numerous instances exist where machine learning (ML) has efficiently accelerated the discovery and development of novel medicines, demonstrating the technology's promise to effectively tackle antibiotic resistance and battle infectious diseases.
7. Machine Learning for Genomic Analysis in Microbiology
Machine learning approaches have transformed genomic analysis in the field of microbiology, particularly in the areas of genome assembly and annotation. By anticipating missing pieces and fixing mistakes, machine learning algorithms can quickly and accurately process enormous volumes of genomic data, improving the speed and accuracy of genome assembly. Through the identification of genes, regulatory elements, and other functional elements contained within DNA sequences, these techniques allow for the automated annotation of genomes.
The field of microbiology has advanced significantly as a result of the merging of genomics and machine learning technology. Researchers can gain new insights into microbial diversity, evolution, and functional genomics by utilizing machine learning to analyze genomic data. The identification of genetic markers for the classification of microbial species, the prediction of gene functions, and even the identification of putative therapeutic targets within microbial genomes are made possible by this synergy. The integration of genomics and machine learning expedites scientific endeavors and broadens our comprehension of microbial systems to unprecedented levels.
8. Automation of Laboratory Processes Using Machine Learning
The use of machine learning in microbiology has completely changed several facets of investigation and interpretation. Automating lab procedures is one important area where machine learning is having a big influence. Routine processes like bacterial identification and categorization can be reduced and refined to improve accuracy and productivity by utilizing machine learning techniques.
Large volumes of data can be used to train machine learning algorithms to identify patterns in bacterial samples, facilitating the quicker and more precise identification of various species. This automation eliminates the possibility of human error in laborious activities while simultaneously cutting down on the amount of time needed for manual processing. Consequently, instead of wasting hours on monotonous laboratory work, researchers may now concentrate more on data interpretation and analysis.
There are numerous advantages to using ML-driven automation in microbiology labs. The first benefit is that it greatly accelerates sample processing, enabling researchers to examine bigger datasets more quickly. This faster speed can result in more rapid insights and discoveries in the field of microbiology, which will ultimately advance our knowledge of different diseases and their traits.
By using machine learning to automate repetitive operations, labs can increase the overall accuracy of their findings. More accurate identification and classification of bacterial strains results from machine learning (ML) models, which constantly improve their predictive power by learning from past data inputs. In clinical settings, where prompt and accurate diagnoses are critical for patient care, this increased accuracy is essential.
To rephrase what I just said, there is a great deal of promise for improving research and diagnostic accuracy and efficiency through the use of machine learning to automate microbiological lab procedures. Scientists may speed up their workflows, lower errors, and find fresh insights that could have stayed undiscovered by using AI-driven technology. We anticipate more developments as this subject progresses, which will influence how microbiological research is conducted in the future and how it is applied in other businesses.
9. Challenges and Limitations of Applying Machine Learning in Microbiology
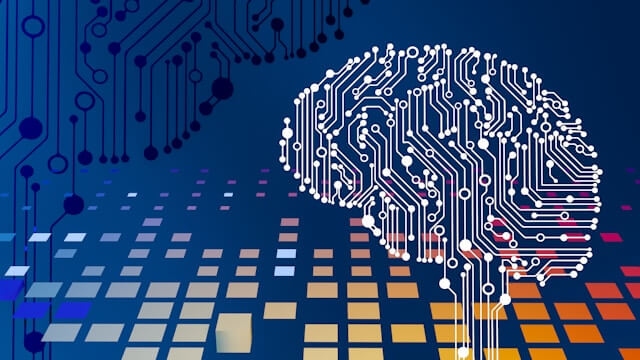
There are unique difficulties in incorporating machine learning (ML) into microbiological research. The scarcity of high-quality data for microbiological machine learning model training is one prevalent problem. The intricacy and fluctuation of microbiological data provide challenges to the acquisition of extensive and varied datasets, which are essential for the creation of resilient models.
The interpretability of ML models in microbiology is another difficulty. These sophisticated algorithms frequently operate as "black boxes," making it challenging for academics to comprehend the decision-making process. The adoption of ML solutions in crucial fields like drug discovery or illness diagnostics within microbiology may be hampered by this lack of transparency.
In microbiology, researchers encounter challenges in guaranteeing the dependability and replicability of machine learning results. To prevent biases or overfitting problems, models must be validated and optimized over a variety of datasets and experimental settings.
When using machine learning in microbiology, restrictions pertaining to data quality can present formidable obstacles. Problems like noise, missing values, or inconsistent data gathering methods can have a detrimental effect on machine learning models' performance and generalizability.
Interpretability issues present yet another barrier to machine learning applications in microbiology. Accurate forecasting and useful insights require an understanding of the intricate interactions that exist between the various elements in microbial ecosystems. But some advanced machine learning algorithms may compromise interpretability in favor of improved performance, posing a trade-off that researchers need to carefully consider.
The extensive application of machine learning techniques in microbiological research environments may be restricted by limitations on computational resources and knowledge. Having access to specialized knowledge and computer resources is essential for creating and implementing machine learning solutions that are specifically designed to tackle microbiological problems.
10. Ethical Considerations in the Use of Machine Learning for Microbiological Research
The proper application of AI/ML algorithms in microbiology is contingent upon ethical considerations while implementing machine learning. Since sensitive information gathered for research needs to be protected to avoid abuse or breaches, data privacy is a major concern. Another important concern is bias mitigation, since algorithms may unintentionally reinforce preexisting biases in the data. To guarantee that judgments are just and accountable, responsible AI deployment requires openness in the development and application of algorithms. In order to promote honesty and integrity in machine learning applications in microbiological research, it will be essential to address these ethical issues.
11. Future Prospects: Innovations at the Intersection of Machine Learning and Microbiology
Future possibilities in the nexus of microbiology and machine learning are bright, with new trends and possible discoveries in the works. It is anticipated that further ML integration with microbiology would transform medical procedures and scientific findings in a variety of ways.
Antimicrobial resistance prediction modeling is one fascinating field of study. Large-scale datasets can be analyzed by machine learning algorithms to forecast the evolution of antibiotic resistance in bacteria, facilitating the creation of more potent countermeasures against this danger to public health.
A greater comprehension of the intricate microbial communities found within the human body may result from the application of machine learning (ML) in microbiome research. Researchers could revolutionize personalized medical techniques by using powerful data analytics to unearth new insights about the significance of these microbiomes in human health and disease.
Machine learning in bioinformatics is expected to improve our capacity for accurate and timely genetic data analysis. This might greatly speed up the process of identifying new infections, ease the process of finding new drugs, and enhance methods for diagnosing infectious disorders.
We can expect a future where cutting-edge technology enable scientists and healthcare practitioners to make ground-breaking discoveries that have a positive influence on global health outcomes, as machine learning advances continue to transform microbiological research.
12. Conclusion
In summary, there has been a great deal of promise and potential demonstrated by machine learning applications in microbiology. We have discussed the applications of machine learning in personalized medicine, drug development, and microbiological identification throughout this blog post. More effectively and precisely than ever before, researchers may now examine enormous volumes of microbiological data by utilizing cutting-edge algorithms and computational tools.
It is impossible to exaggerate how revolutionary machine learning can be for the field of microbiology. By facilitating quicker and more accurate analysis, this technology has the potential to completely transform the area and result in novel discoveries and improvements in our knowledge of microbes. Future research orientations in microbiology will undoubtedly be greatly influenced by machine learning, opening the door to creative solutions to challenging problems in environmental science, healthcare, and agriculture, among other fields.
Machine learning in microbiology is a novel approach that has the potential to significantly advance scientific research and spark new discoveries in the discipline. Through leveraging artificial intelligence and big data analytics, scientists can uncover important discoveries that were previously obscured by the immense intricacy of microbiological systems. We anticipate seeing even more amazing applications arise as we investigate this nexus between biology and technology, which will ultimately fundamentally alter how we study and engage with microbes.