1. Introduction
The Internet of Things (IoT), industrial machinery, and advanced analytics have come together to form the Industrial Internet. By enabling smarter, more effective operations through data-driven insights, it has completely changed traditional industries. Utilizing the massive volumes of data produced by industrial operations is essential to fostering innovation and competitiveness in the modern digital age. This is where the importance of industrial data science becomes clear.
The use of data science methods to glean insightful information from industrial data is known as industrial data science. Industrial data scientists can find patterns, trends, and correlations that drive process improvements, predictive maintenance, and optimization in a variety of industrial settings by analyzing complicated datasets. The need for Industrial Data Science knowledge is only going to increase tremendously as more companies adopt automation and connectivity. We will go into greater detail about the importance of Industrial Data Science in the context of the Industrial Internet in this blog post, as well as the reasons why it is crucial for contemporary industries to prosper in the data-driven world of today.
2. Evolution of Industry 4.0
Following Industry 4.0, the transition from traditional industries to smart factories represents a significant change in the way things are made and produced. The incorporation of cutting-edge technologies into industrial processes, including robotics, AI, machine learning, and the Internet of Things, is what defines this shift. Smart factories are networked, highly automated systems that use real-time data to maximize production, efficiency, and cost-effectiveness.
This shift to smart manufacturing facilities is mostly being driven by data-driven technologies. Through the utilization of extensive data produced by networked apparatus and gadgets, establishments can derive significant knowledge that propels well-informed choices and enhances operational procedures. Businesses can foresee maintenance needs, improve product quality, simplify processes, and eventually reach greater levels of operational excellence with the help of industrial data science.
The way industries function and innovate has been completely transformed by the convergence of digital technology and physical systems. The intelligent factories of today are capable of self-optimization and adaptive decision-making, thanks to the smooth integration of sensors, actuators, and data analytics tools. A paradigm shift toward networked cyber-physical systems that allow for real-time monitoring, analysis, and control of a variety of industrial processes is represented by Industry 4.0.
The importance of industrial data science grows as more sectors embrace the age of digital transformation and automation brought forth by Industry 4.0 concepts. For businesses to remain competitive in the fast-paced business world of today, they must be able to use data successfully to spur innovation and operational efficiencies. In addition to facilitating predictive analytics for demand forecasting, quality control improvements, energy management optimization, and other strategic endeavors, industrial data science also makes predictive maintenance possible.
Furthermore, as previously stated, the transition from conventional industries to smart factories serves as the foundation for the revolutionary path towards Industry 4.0. This shift is facilitated by data-driven solutions, which provide enterprises with useful insights from industrial data analytics. In an increasingly digitalized industrial ecosystem, businesses looking to seize new chances for growth, efficiency improvements, and long-term competitive advantage must embrace industrial data science.
3. Importance of Data in Industrial Settings
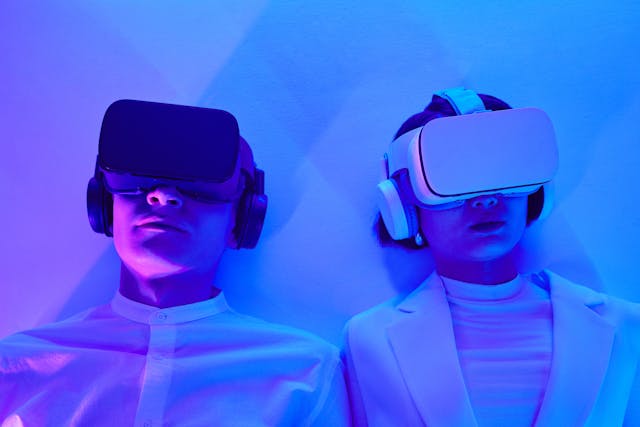
In industrial contexts, data is essential for promoting productivity, efficiency, and innovation. Industries can enhance overall operational performance, optimize processes, and find patterns for predictive maintenance by utilizing data through advanced analytics and machine learning algorithms. Better decisions may be made based on facts rather than conjecture thanks to real-time data monitoring, which lowers costs and boosts productivity in a variety of industrial sectors.
Businesses that don't use data well have serious problems. Businesses may experience unintended downtime as a result of inadequate predictive maintenance insights, ineffective resource allocation that raises operating costs, and lost possibilities for process innovation and optimization if they do not properly utilize data. The incapacity to make well-informed judgments grounded in real-time data might impede overall corporate growth and lead to a decrease in market competitiveness. As a result, businesses hoping to prosper in the fiercely competitive market of today must embrace industrial data science.
4. Industrial Internet Technologies
In the context of the Industrial Internet, a number of important technologies are revolutionizing whole industries. The Internet of Things, or IoT, makes it possible for machines and other objects to seamlessly connect to one another and gather and share data in real time. Artificial intelligence, or AI, enables systems to analyze this data intelligently and make judgments and predictions without the need for human input. By using data patterns to learn from, machine learning algorithms enhance this procedure and increase its efficiency and accuracy. Large volumes of industrial data may be safely stored, processed, and accessed thanks to cloud computing's architecture.
Because it allows objects to communicate with centralized systems and with each other, the Internet of Things (IoT) forms the basis of the Industrial Internet ecosystem. Continuous monitoring of energy consumption, temperature fluctuations, equipment performance, and other critical parameters that affect operations is made possible by this connectivity. AI uses this flood of data to find patterns, abnormalities, or possible problems early on and provide insights that help with proactive decision-making. By continuously evaluating data streams to optimize processes, forecast repair requirements, or increase overall operational efficiency, machine learning algorithms boost this capability.
The foundation of these technologies is cloud computing, which offers the processing power and flexible storage options required to manage massive amounts of industrial data. It helps businesses to safely centralize their data repositories and allows for real-time access from any location in the world. Without having to make large expenditures in on-premise equipment, businesses may obtain useful business intelligence from their industrial data by utilizing cloud-based analytics tools.🗜
When combined, these technologies create a potent framework that opens up new possibilities for gathering, processing, and using industrial data. By using real-time insights and predictive skills obtained from interconnected systems, they help firms make the shift from reactive to proactive initiatives. Businesses that want to remain competitive in the quickly changing industrial landscape of today—where innovation, efficiency, and agility are critical success factors—must adopt these technologies.
5. Role of Industrial Data Science
Leveraging data in the industrial Internet environment requires the application of industrial data science. Utilizing data science methodologies, it entails drawing conclusions, trends, and forecasts from the massive volumes of data produced in industrial environments. Predictive maintenance helps businesses prevent expensive downtime and save maintenance expenses by using past equipment data to identify possible problems before they happen. In order to optimize processes, businesses can reduce costs and increase productivity by identifying inefficiencies and bottlenecks through the analysis of real-time production data. To ensure high-quality output, quality control uses data from sensors and machine learning algorithms to identify anomalies or flaws in items during the production process.
The conversion of unprocessed data into useful insights that improve industrial operations decision-making is a critical function of industrial data science. It offers useful information on production procedures, product quality, and trends in equipment performance by evaluating both historical and current data. These insights enable companies to decide on maintenance plans, operations, and quality control procedures with knowledge. By utilizing sophisticated analytics methods such as machine learning and predictive modeling, Industrial Data Science helps businesses to optimize their operations in a proactive rather than reactive manner, resulting in higher productivity and lower operating expenses. It gives industrial firms the knowledge they need to improve overall productivity and competitiveness in the fast-paced market environment of today.
Industrial data science, in short, is a strategic ally that helps industries by using data to spur innovation and continual improvement in a variety of operational domains. Through revealing latent patterns in intricate datasets, it reveals optimization opportunities that might not be discernible using conventional techniques alone. By being proactive, businesses can minimize risks and maximize efficiency by anticipating possible problems like production delays or equipment malfunctions. Industrial Data Science, which makes use of Artificial Intelligence (AI) and Machine Learning algorithms, can quickly adjust to changing needs and circumstances, giving firms the adaptability that is essential in today's industrial landscapes.
The ultimate objective of industrial data science is to translate information into actions that have real-world implications for businesses in the industrial sector, rather than merely processing it. Through the incorporation of data-driven insights into decision-making procedures across all organizational levels, ranging from strategic planning to shop floor operations, enterprises may augment their flexibility and responsiveness in the current dynamically fast-paced market. Predictive analytics' accuracy in predicting trends enables businesses to allocate resources wisely and continuously improve operational efficiencies. For businesses hoping to prosper in the age of digital transformation, industrial data science thus acts as a catalyst for fostering innovation, enhancing competitiveness, and guaranteeing sustainable growth.
To sum up what I mentioned, industrial data science plays a critical role in enabling the industrial Internet to reach its full potential by transforming raw data into actionable insight that informs choices across an organization's operations. Industry may more effectively manage their assets and meet quality standards and productivity requirements by using Industrial Data Science in predictive maintenance, process optimization, and quality control applications. By adopting this revolutionary strategy for using data analytics to achieve operational excellence and competitive advantage at scale, Industry 4.0 includes current issues as well as opportunities that companies may seize to fully realize connected future potential.
6. Challenges and Opportunities
There are a number of obstacles that prevent smooth adoption in the field of industrial data science. Due to the complexity involved in combining and processing data from many sources, data integration continues to be a challenge. Because industrial data is sensitive, security is critical and requires strong measures to protect against cyber threats. Organizations frequently face challenges due to skill shortages, as experts with expertise in both data science and industrial processes are typically scarce. 🫣
Amid these difficulties, there are encouraging chances for development and creativity. Organizations can gain important insights that promote operational efficiency and well-informed decision-making by successfully utilizing industrial data. Accepting modern analytics provides opportunities for production process optimization, predictive maintenance, and performance enhancement. Businesses that use industrial data well can stay ahead of the curve and provide better products and services, giving them a competitive advantage.
As I mentioned above, there are significant benefits to implementing industrial data science approaches, even while there may be barriers such as data integration, security issues, and a lack of skilled workers. By employing a strategic approach to leverage industrial data efficiently, companies can surmount these obstacles and prosper in a progressively cutthroat market. Those who recognize the revolutionary potential of industrial data science and leverage it to advance their operations will lead the way in the future.
7. Case Studies
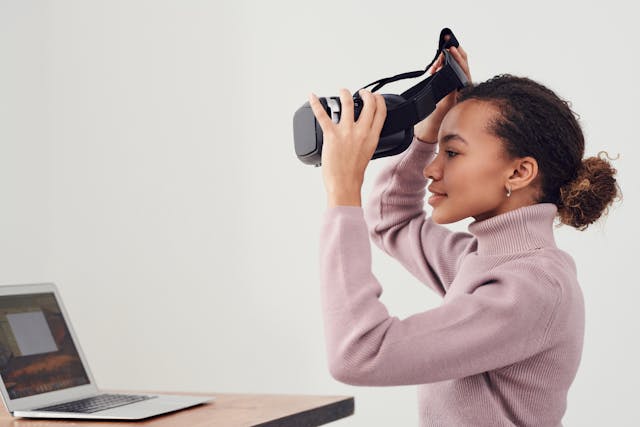
The application of industrial data science has transformed numerous industries recently by allowing businesses to glean insightful information from their operational data. Let's look at a few interesting case studies where businesses have effectively applied industrial data science to improve their operations and increase their profits.
One noteworthy instance is when a large manufacturing corporation used machine learning algorithms to apply predictive maintenance to the data from its production equipment. Through the examination of past data trends, they were able to anticipate certain equipment malfunctions before they happened. In the end, this proactive strategy resulted in considerable cost savings and an increase in operational efficiency by reducing unplanned downtime and optimizing maintenance schedules.
An international logistics company that used industrial data science to improve supply chain operations is the subject of another case study. Through the use of sophisticated analytical methods to their extensive transportation and inventory data, they were able to pinpoint routing inefficiencies, shorten lead times, and enhance the accuracy of inventory management. As a result, the business observed a discernible increase in customer satisfaction levels, lower carrying costs, and higher rates of on-time delivery.
Industrial data science was applied by a utility business to improve energy distribution efficiency and grid dependability. By employing artificial intelligence (AI) algorithms to analyze weather forecasts and sensor data from smart meters, they might more precisely predict demand peaks and instantly modify the distribution of energy. This proactive strategy greatly decreased operational expenses while minimizing energy waste and enhancing overall grid stability.✉️
These case studies demonstrate how companies in a variety of industries can reap real benefits from Industrial Data Science. The strategic application of data science techniques has proven instrumental in improving operational efficiency, lowering costs, and ultimately enhancing the bottom line for companies embracing this transformative technology, from supply chain optimization in logistics and grid management in utilities to predictive maintenance in manufacturing.
8. Ethics and Privacy Concerns
Ethical concerns about the gathering and use of industrial data have become critical in the age of the Industrial Internet. Privacy, security, and fairness are among the many issues brought up by the massive volumes of data produced by networked industrial systems. To preserve integrity and confidence, companies must give ethical data handling procedures top priority.
Regulations protecting privacy are essential to guaranteeing the moral application of industrial data. Adherence to legal frameworks like GDPR, HIPAA, or particular industry norms serves to protect confidential data from unwanted access or mishandling. Adopting strong data anonymization strategies, encryption techniques, and access controls are critical industry best practices for safeguarding individuals' right to privacy in work environments.
Companies need to encourage a data-ethics culture among their employees and openly share with stakeholders their policies on the gathering and use of data. Upholding ethical norms in industrial data science requires establishing explicit methods for handling potential breaches, consent mechanisms, and data ownership. Companies may responsibly and sustainably traverse the complicated environment of the Industrial Internet by giving ethics and privacy concerns first priority.
9. Future Trends in Industrial Data Science
It is anticipated that edge computing utilization in Industrial Data Science would soar in the future. By bringing data processing closer to the source, edge computing minimizes latency and maximizes bandwidth utilization. This trend will increase operational efficiency and enable predictive maintenance by enabling enterprises to make decisions in real-time based on localized data.
The growing use of digital twins in industrial operations is another trend that is predicted. By building virtual versions of real assets or systems, digital twins enable virtual environment simulation, monitoring, and analysis. Industries can foresee problems before they arise, optimize performance, and simplify maintenance procedures by utilizing digital twins.
Blockchain integration is expected to improve data security, transparency, and traceability in transactions and supply chains, hence revolutionizing industrial data science. Industries can guarantee data integrity and immutability and build stakeholder trust by implementing blockchain technology. The integrity of industrial data systems will depend heavily on the increased security that blockchain offers.
These new developments in the field of industrial data science have the potential to completely transform industrial operations by promoting increased security, predictability, and agility. By enabling quicker data processing at the network's edge, edge computing can help industries make decisions in real time and minimize downtime. With sophisticated simulations and predictive analytics, digital twins will transform industries' asset visualization and performance optimization.
Ensuring data integrity, security, and accountability within industrial ecosystems will be made possible through the integration of blockchain technology into industrial data science. Blockchain's decentralized structure and cryptographic principles enable enterprises to reduce cybersecurity threats and create unchangeable records of operations and transactions. These upcoming trends portend a revolutionary change in industrial processes toward ones that are powered by cutting-edge data science technologies and are more intelligent, safe, and efficient.
10. Implementing Industrial Data Science Strategies
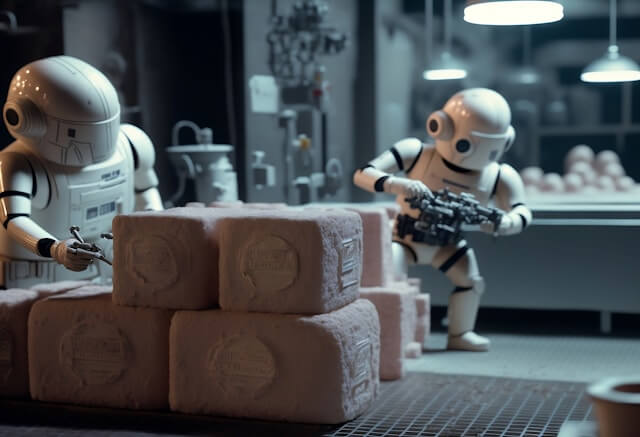
Adopting industrial data science initiatives necessitates a methodical approach customized to each organization's particular requirements. Companies should start this process by outlining their objectives and key performance indicators (KPIs) in detail. Success depends on their ability to comprehend the particular issues that data science is intended to address.
Organizations should then concentrate on putting together a knowledgeable data science team with the appropriate tools and technology. Effective data initiatives can be started with a solid basis by hiring engineers, analysts, and data scientists with experience in industrial applications. Investing in cutting-edge analytics platforms and technologies can expedite the modeling and data processing phases.
For industrial data science to succeed in the long run, organizations must develop a data-driven culture. Starting at the top, the leadership sets the example by highlighting the significance of making decisions based on facts. A more creative and analytical work environment can be achieved by promoting departmental collaboration and igniting curiosity about data insights.
Companies should give priority to continual training and upskilling programs for staff members at all levels in order to promote continuous improvement. Giving teams access to data science methodologies workshops, courses, and resources guarantees that they are aware of the most recent advancements in the industry. Motivating staff members and recognizing their contributions to expanding the organization's data capabilities might help to raise spirits.
As previously mentioned, the successful execution of industrial data science strategies necessitates a blend of well-defined goals, proficient personnel, resilient technological frameworks, and a nurturing corporate culture. Organizations may leverage data science to promote innovation and gain a competitive edge in the current digital landscape by adhering to a roadmap that suits their unique objectives and problems and fostering a data-driven attitude at all organizational levels.
11. Collaboration Between IT and OT
In today's corporate world, cooperation between Information Technology (IT) and Operational Technology (OT) is essential. The integration of data from machines, sensors, and control systems with conventional IT systems like databases and analytics tools is made easier in industrial settings by the convergence of IT and OT. A more comprehensive understanding of operations is made possible by this integration, which boosts production, efficiency, and safety.
Success in the field of industrial data science depends on the IT and OT teams working together effectively across functional boundaries. IT specialists contribute their expertise in analytics, data management, cybersecurity, and software development. Conversely, OT specialists provide information on the finer points of industrial procedures, machinery performance, safety guidelines, and operational needs. Organizations may fully utilize their data to spur innovation and accomplish business goals by fusing these distinct skill sets and viewpoints.
Beyond just integrating technology, IT and OT must work together to build a culture of communication and cooperation between departments that have traditionally functioned in isolation. Supporting candid communication, recognizing objectives and obstacles together, and taking responsibility for project results together are crucial components of an industrial data science initiative's effective execution. Adopting this cooperative strategy enables companies to efficiently utilize their combined experience and open up new avenues for growth and optimization in the Industry 4.0 era.
12. Conclusion
After putting everything above together, we can say that by utilizing data analytics, industrial data science is vital to improving industry efficiencies and promoting innovation. Businesses may quickly make well-informed decisions and optimize operations by extracting important insights from their operational data through the use of sophisticated algorithms and machine learning techniques. In the data-driven world of today, this not only results in cost reductions but also creates competitive advantages.
To remain competitive in the Industrial Internet era, industries must adopt and invest in Industrial Data Science technology. Organizations can find hidden trends, anticipate maintenance needs, and optimize operations for optimal efficiency by further exploring and implementing these techniques. Beyond short-term rewards, long-term sustainability and growth are also possible outcomes. Thus, in an ever-changing digital ecosystem, the incorporation of Industrial Data Science into corporate strategies is essential to success and relevance.