1. Introduction to IoT Data Analysis
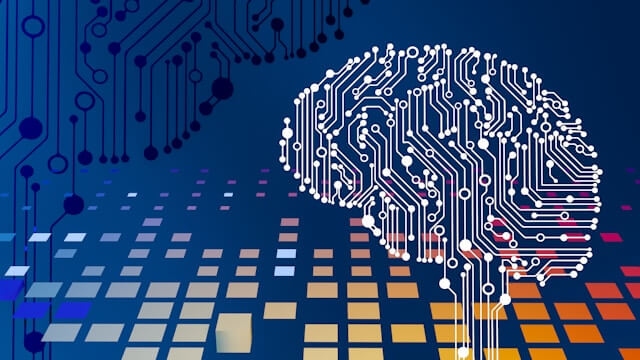
The analysis of data created by networked devices presents distinct problems because of the large volume and variety of data supplied by IoT devices. IoT devices gather and send data over networks, producing enormous datasets that need to be interpreted using advanced analytical methods. This blog post highlights the difficulties in extracting significant insights from this important data by examining five major issues with IoT data analysis.
Industry revolutions have been brought about by the development of IoT technology, which has made real-time monitoring, automation, and predictive maintenance possible. Nevertheless, there are obstacles unique to this abundance of data that must be overcome in order to extract useful insight. IoT data analysis requires specialized tools and tactics to efficiently manage many challenges, including managing the velocity and volume of data streams and guaranteeing data security and privacy.
We will go further into each problem in this series to comprehend the complexities of IoT data analysis. Organizations may better position themselves to take advantage of IoT analytics' full potential and use these insights to spur innovation and operational efficiency by identifying these obstacles. As we examine the five obstacles that influence the field of IoT data analysis, stay tuned.
2. Importance of IoT Data Analytics
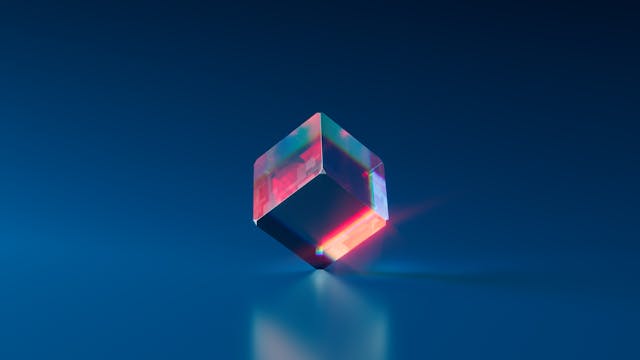
Because Internet of Things (IoT) data can yield significant insights, its analysis is essential. Massive volumes of data are produced by IoT devices, including location data, sensor readings, and user interactions. Businesses can learn more about their operations, consumer behavior, and market trends by examining this data. With this knowledge, they may make data-driven decisions that boost productivity, cut expenses, and stimulate creativity.
One important component of predictive maintenance is IoT data analytics. Businesses may anticipate when equipment is likely to break and take proactive steps to reduce downtime by evaluating historical data patterns and real-time device performance monitoring. This increases overall productivity while also reducing the expense of unplanned repairs.
Analytics from IoT data are crucial to improving customer experiences. By evaluating data from numerous touchpoints like as mobile apps, wearables, and smart home devices, businesses can personalize their products depending on individual preferences and habits. Increased client happiness and loyalty follow, which eventually spur revenue development.
For organizations to get the most out of their IoT investments, IoT data analytics is essential. They can gain insightful knowledge, increase operational effectiveness, maximize resource usage, and improve client experiences thanks to it. Using IoT data analytics can help businesses stand out in the current digital economy by allowing them to make better decisions more quickly than in the past.
3. Challenge 1: Handling Big Data in IoT Analyses
The first challenge in interpreting data from the Internet of Things (IoT) is managing the enormous volumes of data that are produced. Because IoT devices are always watching and sensing, they generate enormous amounts of data. There are problems with storage, processing power, and analytic capacities due to this massive intake of data. The volume and complexity of IoT data may be too much for traditional data management systems to handle, necessitating creative solutions for effective processing and the extraction of useful insights.
Organizations need to invest in scalable infrastructure that can handle the exponential expansion of IoT devices and the data they generate in order to solve the problem of handling big data in IoT analytics. Platforms for cloud computing provide computational power and adaptable storage to meet the changing demands of IoT environments. Large datasets can be processed in parallel using distributed computing frameworks like Hadoop or Spark, providing quicker analytics and real-time insights from IoT data streams.
Using data compression methods like differential or predictive coding can assist in reducing IoT dataset sizes without sacrificing crucial information. For more effective storage and analysis, raw sensor inputs can be condensed into relevant patterns or trends using data aggregation and summarization techniques. Overcoming the difficulty of managing big data in IoT analyses requires a comprehensive strategy that incorporates scalable cloud services, dependable hardware infrastructure, and optimal data processing methods.
4. Challenge 2: Ensuring Data Security and Privacy in IoT
The second challenge in IoT data analysis is protecting privacy and security of data. IoT devices generate enormous volumes of sensitive data, including location information, behavioral patterns, and personal information. As a result, it is imperative to protect this data against breaches or unwanted access.
The potential vulnerability of Internet of Things devices is one of the main causes for concern. Numerous Internet of Things devices are susceptible to security flaws, which makes them a prime target for cyberattacks. These flaws provide hackers access to the data that these devices are transmitting or storing.
The vast amount of data generated by Internet of Things systems presents another difficulty. Effectively handling this enormous inflow of data while maintaining strict security protocols might be challenging. To reduce security threats, encryption technologies, safe authentication procedures, and frequent security audits are necessary.
Managing data security and privacy gets significantly more challenging as more devices get connected within IoT ecosystems. Establishing explicit standards about who can access what data, how it is stored and communicated, and how long it should be kept becomes essential for companies.
In the field of IoT data analysis, compliance with data protection standards also poses a considerable difficulty. Laws governing the gathering and use of personal data differ throughout jurisdictions. A strong foundation for securely managing user data and painstaking attention to detail are necessary to guarantee that IoT operations comply with these regulations.🗒
Furthermore, resolving the issues of data security and privacy in the Internet of Things is critical to building stakeholder and user trust, as I said above. Organizations may successfully traverse the challenges of analyzing IoT data while protecting sensitive information by proactively deploying strong security measures, remaining up to date on developing risks, and adhering to regulatory regulations.
5. Challenge 3: Implementing Real-time Data Processing for IoT
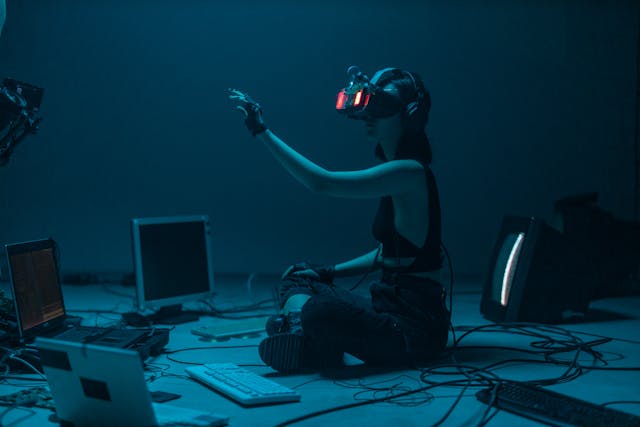
Putting real-time data processing into practice is the third challenge in IoT data analysis. Real-time data processing can be difficult and resource-intensive due to the large volume of data generated by Internet of Things devices. For timely insights, organizations want resilient systems that can manage constant data streams with low latency.
Sophisticated algorithms and infrastructure are needed for real-time processing in order to swiftly filter, compile, and evaluate incoming data. It includes effectively handling large amounts of data, which frequently calls for sophisticated database technology and potent computer resources like cloud or edge computing solutions. 😄
Making sure scalability can handle increasing data loads without compromising speed is a major challenge in putting real-time data processing for IoT into practice. Systems that can grow dynamically to handle more data must be designed by organizations in order to handle growing volumes of data as IoT installations get larger over time.
Maintaining data integrity and quality in real-time processing systems is a challenge for organizations. There is a greater chance of mistakes, discrepancies, or missing information as data comes in regularly. To guarantee the dependability of real-time insights obtained from IoT data, it becomes imperative to implement methods for data validation, error handling, and redundancy.
Overcoming the problem of real-time data processing for IoT requires a multifaceted strategy that includes rigorous data quality assurance procedures, scalability planning, algorithmic efficiency, and technology infrastructure. In today's fast-paced digital landscape, enterprises may produce actionable insights and economic value by fully utilizing IoT data analytics by overcoming these barriers.
6. Challenge 4: Dealing with Data Variety and Quality in IoT Systems
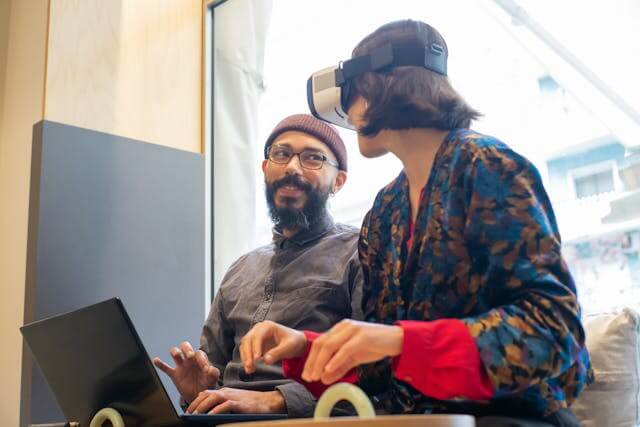
Challenge 4: Dealing with Data Variety and Quality in IoT Systems
Managing the enormous diversity and guaranteeing the quality of the data is one of the major issues in IoT data analysis. IoT systems provide data from a wide range of sources, including social media feeds, gadgets, sensors, and more. For analysis to be effective, a wide range of data kinds, formats, structures, and semantics are produced by this heterogeneity, which must be harmonized.
Problems with data quality can be caused by a number of things, including malfunctioning sensors, poor communication, the environment, or even malevolent manipulation. Since erroneous or incomplete data can seriously affect the validity of analytical findings and decisions based on them, it becomes imperative to ensure data quality.
To overcome these obstacles, methods such as data cleansing, normalization, standardization, and validation procedures are used to guarantee the dependability, quality, and consistency of the data from various sources. To increase the overall quality of the data, abnormalities, outliers, and inconsistencies can be found and removed with the aid of sophisticated analytics tools and algorithms. Strong data governance procedures can offer guidance for upholding high-quality data standards across the Internet of Things network.
Managing a variety of potentially inconsistent IoT data necessitates a comprehensive strategy that combines organizational tactics and technology solutions. The maintenance of high-quality data standards inside IoT systems can be facilitated by implementing stringent rules for data collection, storage, processing, and analysis. Additionally, automation technologies can be utilized for regular checks and validations.
7. Challenge 5: Extracting Actionable Insights from IoT Data
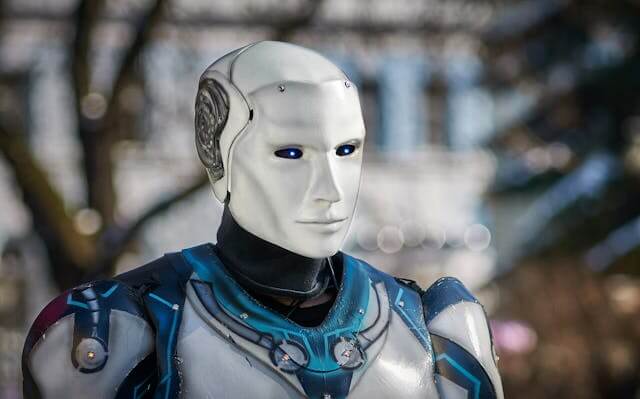
Challenge 5: Extracting Actionable Insights from IoT Data
Finding valuable insights that can inform decisions and actions is the last difficulty after conquering the ones related to data collection, integration, cleaning, and processing in Internet of Things systems. Efficiently deriving useful insights from IoT data is difficult due to its vast volume and complexity.
Organizations require sophisticated analytics tools and algorithms that can handle the variety of data kinds and structures found in IoT datasets in order to effectively address this challenge. Real-time streaming data processing capabilities are essential for these tools in order to provide prompt decision-making based on current knowledge.
Another difficulty that organizations encounter is guaranteeing the dependability and precision of the insights gleaned from IoT data. Incomplete or inaccurate information might result in poor decisions that have serious repercussions. It is imperative to establish comprehensive procedures for data quality verification and validation in order to preserve the accuracy of insights obtained from Internet of Things data.
Putting IoT data into context is crucial to producing insights that can be put to use. Drawing relevant conclusions requires an understanding of the connections between various data points and how those connections affect business operations. Businesses must make investments in data visualization strategies that enable stakeholders to efficiently understand complicated IoT datasets.
Analyzing IoT data presents a huge barrier in terms of converting technical insights into business value. Maximizing the effectiveness of IoT projects and fostering organizational buy-in require effectively and clearly communicating findings to non-technical stakeholders.
It takes a strategic approach that combines deep subject knowledge, efficient communication tactics, and powerful analytical skills to overcome the issue of obtaining meaningful insights from IoT data. Through the resolution of these fundamental issues, enterprises can fully realize the benefits of their IoT investments and produce observable business results by making well-informed decisions based on insightful data obtained from their networked devices.
8. Tools and Technologies for Effective IoT Data Analysis
Effective insights from Internet of Things (IoT) data analysis depend on having the appropriate tools and technologies. The variety of IoT data sources, which can contain both structured and unstructured data from different devices, is one of the difficulties in this field. Tools that can efficiently process and analyze many data types are needed to handle this variety.🙂
The scalability of IoT data analysis is another problem. Large volumes of real-time data may be too much for typical analytics tools to handle as the number of connected devices increases dramatically. By facilitating quicker processing and analysis of large datasets, scalable technologies like cloud-based platforms and edge computing solutions can assist in overcoming this obstacle.
When working with IoT data, ensuring data security and privacy is a major problem. To guard against cyber threats and unauthorized access, it's critical to use strong security protocols and encryption processes when sensitive information is transferred between devices, networks, and cloud services.
Data integration and standardization are challenging when integrating heterogeneous datasets from multiple Internet of Things sources. Coherently consolidating and analyzing information can be challenging since multiple devices may output data in different forms or standards. Accurate analysis and useful insights depend on the use of data integration solutions that can harmonize different datasets into a common format.
Finally, using real-time analytics for Internet of Things data comes with its own set of difficulties. Processing real-time streaming data from sensors or devices demands strong technology that can handle high-velocity data with low latency. Using real-time analytics frameworks such as Spark Streaming or Apache Kafka can help quickly extract useful insights from continuously streaming IoT data.
9. Case Studies on Successful IoT Data Analysis Implementations
Case studies of effective IoT data analysis deployments provide insightful information about how businesses can overcome obstacles and realize the full potential of IoT technology. Through the examination of real-world instances, businesses can get practical ways for properly interpreting IoT data and benefit from the experiences of others.
A smart manufacturing facility that used IoT sensors to track equipment performance in real time is one example of a case study that is worth considering. The organization was able to foresee maintenance needs and detect patterns by gathering and evaluating data on machine productivity and health. This led to a reduction in downtime and an increase in overall efficiency.
An additional noteworthy instance is a smart city initiative that utilized Internet of Things data analysis to enhance transportation systems. Through the amalgamation of traffic flow data from diverse sensors and sources, urban planners have effectively bolstered traffic regulation, mitigated gridlock, and elevated the general standard of living for inhabitants within cities.
These case studies demonstrate how, when applied strategically and with a firm grasp of the particular business objectives, IoT data analysis may have a transformative effect. Organizations can more effectively manage the difficulties involved in evaluating IoT data and produce significant results in their own operations by taking note of successful implementations.
10. Future Trends in Analyzing IoT Data
The analysis of Internet of Things (IoT) data will be shaped in the future by a number of trends, which presents enterprises with both opportunities and challenges. Data generation will expand rapidly in terms of volume, diversity, and velocity as IoT devices continue to proliferate across many industries. It will take sophisticated analytics tools and methods to effectively extract actionable insights from this data flood.
Second, it's anticipated that edge computing technologies will become increasingly important for IoT data analysis. Organizations may improve real-time decision-making skills, lower latency, and allay privacy and security worries related to sending sensitive data to centralized servers by processing data closer to the point of generation.
The ways in which IoT data is gathered, stored, and analyzed are about to change dramatically as a result of the convergence of IoT with other technologies like blockchain and artificial intelligence (AI). With the use of AI algorithms, it is possible to find patterns in IoT data that people might miss, opening up useful applications like anomaly detection and predictive maintenance. Blockchain technology can protect IoT device-to-IoT device transactions, guaranteeing data integrity and building stakeholder trust.
Organizations will need to implement strict procedures to maintain compliance while responsibly drawing insights from IoT data as regulations surrounding data privacy and security become increasingly demanding globally, as evidenced by efforts like the GDPR and CCPA gaining prominence. In this context, using appropriate anonymization methods and strong cybersecurity procedures will be essential.
Lastly, how businesses evaluate IoT data will be impacted by the move towards sustainability and green activities. Businesses will need to adopt eco-friendly practices and energy-efficient algorithms in order to reduce their carbon footprint and maximize the potential of the Internet of Things to improve operations. As previously mentioned, it will be imperative for enterprises to stay up to date on these developing trends if they want to fully leverage IoT data analytics in the dynamic digital ecosystem.
11.Conclusion: Overcoming Challenges and Maximizing the Potential of IoT Data
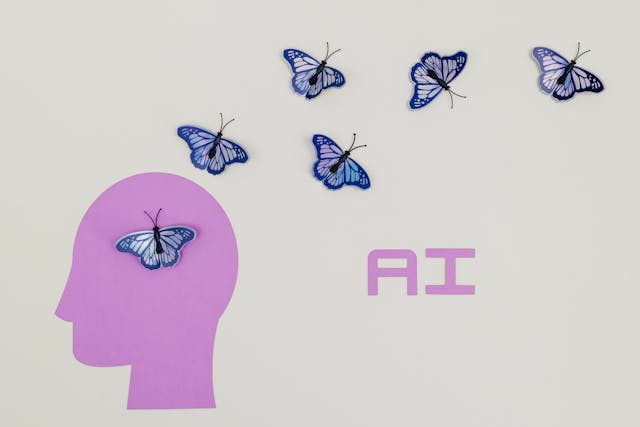
Conclusion: Overcoming Challenges and Maximizing the Potential of IoT Data
To put it briefly, in order for enterprises to fully utilize the promise of Internet of Things (IoT) data, they must properly solve the unique issues that come with its analysis. Businesses can gain important insights, strengthen decision-making procedures, increase operational effectiveness, and spur innovation by comprehending and solving these challenges.
Organizations should invest in strong data management methods, including data collecting, storage, integration, and security measures, to optimize the benefits of IoT data analysis. Making use of cutting-edge analytics technologies like AI and machine learning can aid in extracting valuable knowledge from massive IoT data sets.
Organizations can facilitate effective IoT implementations by tackling obstacles such as data quality issues, scalability concerns, interoperability issues, security threats, and regulatory compliance requirements. By conquering these obstacles, companies will be able to leverage the revolutionary potential of IoT data and gain a competitive edge in the current digital environment.