1. Introduction
In hypothesis testing, the word "statistical significance" is used to assess the likelihood that an observed effect is the result of a relationship between variables or is just the product of random chance. It assists researchers in drawing inferences from their data by calculating the likelihood that the outcomes are not the product of chance alone. Nevertheless, practical or business significance is not always a direct correlate of statistical significance.
The distinction between business importance and statistical significance is seen in the areas in which they are focused. Statistical significance, which frequently makes use of measures like p-values, highlights the dependability of study findings based on probability computations. However, business significance explores how those discoveries might be applied in the actual world. Even while a finding is statistically significant, it may not have much of an effect on how a firm operates or makes decisions, which makes it less useful from a business perspective.
To put it simply, statistical significance tells us a lot about how likely it is that results will come from chance alone, but in business settings, what really counts is whether the conclusions have practical applications and the potential to benefit an organization. This contrast emphasizes how crucial it is to take into account the larger ramifications and real-world uses of research findings in a business setting rather than only depending on statistical values.
2. Importance of Statistical Significance
A key idea in research and experimentation is statistical significance, which tells us whether an observed result is more likely to be the consequence of a true effect or just random variation. By showing the likelihood that the results are not random, it provides researchers confidence in their findings. Usually, this significance threshold is set at 0.05, which indicates that there is a 5% possibility that the outcomes were the product of chance.
Statistical significance is essential to decision-making in many different disciplines. For example, statistical significance is used in clinical trials in medicine to determine whether a new treatment is more effective than an existing one. A/B testing in marketing uses statistical significance to identify which campaign version performs better. Statistical significance is frequently used in the social sciences to support hypotheses and derive insightful conclusions from data analysis.
For data-driven experiments and research projects to yield reliable findings, a thorough understanding of statistical significance is necessary. Although statistical significance is a valuable tool for researchers and analysts, it is vital to keep in mind that statistical significance does not always equate to practical or business relevance. A thorough understanding of statistical methodologies and their wider application context is necessary for successful result interpretation and application.
3. Limitations of Statistical Significance
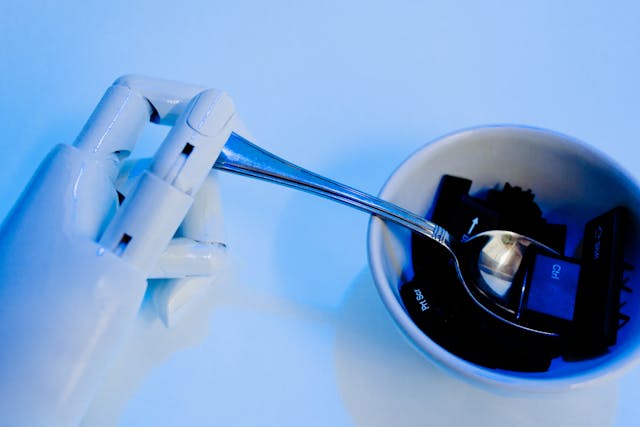
Even while statistical significance is an important study metric, there are situations in which it cannot reveal practical implications. A potential constraint is sample size; whereas a larger sample size may enhance statistical significance, it may not always translate into practical importance. Statistical tests by themselves could miss interactions or confounding factors that affect business results.
Making poor decisions might arise from interpreting data results without considering the business environment. For instance, a marketing plan that increases website clicks and produces statistically significant results could not result in better sales conversions. Ignoring the larger corporate objectives in favor of statistical data alone can lead to misaligned priorities and ineffectual initiatives.
It is impossible for statistical significance to fully convey the subtleties of market dynamics or customer behavior. Even when a study reaches statistical significance, real-world outcomes might be greatly impacted by human variability in preferences and outside variables like rivalry. Businesses must be aware of these constraints in order to base their strategy decisions on both practical relevance and statistical rigor.
4. Understanding Business Significance
The practical relevance and influence of a study conclusion on actual decision-making in the real world are referred to as business importance. Business significance is concerned with whether the results have practical ramifications for a business or organization, as opposed to statistical significance, which is concerned with the possibility that an observed result has not happened by accident. This idea is important since statistical significance by itself could not necessarily result in noticeable improvements or modifications in a business setting.
Making well-informed decisions with a significant impact requires taking statistical data into account in addition to business relevance. The practical significance or business ramifications of the findings are not always indicated by statistical significance, despite the fact that it offers insightful information about the likelihood of Type I errors and the dependability of the data. Decision-makers can assess whether the observed effects are significant enough to justify action or plan revisions by including considerations of business relevance.
Fundamentally, knowing the business value of research findings aids in bridging the gap between data analysis and practical insights, allowing firms to effectively use research findings to inform strategies, enhance workflows, and produce favorable results. Businesses are better able to make decisions that result in significant outcomes and long-term success when they combine statistical rigor with practical relevance.
5. Case Studies: Statistical vs. Business Significance
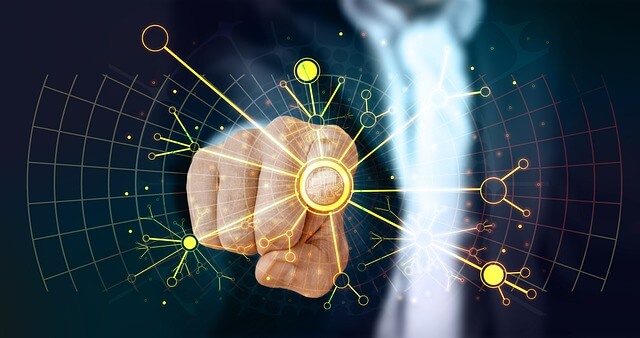
Statistical significance has not always translated into significant business implications. A/B testing produced some remarkable results, such as statistically significant increases in user engagement measures like click-through rates and average session durations following a website redesign. That being said, neither revenue nor client retention rates increased as a result of this. The statistically substantial gains did not translate into real commercial success.
On the other hand, there have been cases where statistically inconsequential results have had a huge impact on enterprises. Take into consideration a market research study that seeks to determine how a new product feature affects consumer happiness. Qualitative feedback from focus groups yielded important ideas that, even though they did not approach statistical significance, ultimately transformed the strategy for product development and greatly increased consumer loyalty. In this instance, the qualitative data revealed important economic implications even if the figures did not differ considerably.
6. Balancing Statistical and Business Considerations
For data analysis to be effective, statistical and business issues must be balanced. Setting specific business objectives before beginning data analysis is one tactic to make sure statistical results support these aims. To ascertain the actual relevance of statistics results, take into account their practical consequences within the business context.
It is imperative that stakeholders from many domains participate in the analytic process in order to incorporate both statistical and business significance. Working with people who are familiar with the nuances of business can yield insightful information on how statistical results can be used in practical contexts.
Employing a combination of statistical tools and business acumen is one of the best methods for making educated decisions. When evaluating statistical results, take into account not just their numerical importance but also how they may influence important business KPIs and provide actionable insights. Frequently convey results in an easily comprehensible format to close the knowledge gap between statistical analysis and real-world application.
7. Communicating Results Effectively
It's crucial to emphasize the commercial implications of statistical findings rather than merely the significance levels when presenting them to stakeholders. Convert statistical findings into practical understandings that tackle important business issues. Speaking clearly and succinctly is preferable to overusing technical jargon.
Charts, graphs, and infographics are examples of visualization techniques that can be used to clearly present complex data. Stakeholders may more quickly understand the main points when there are visual aids available. Clearly illustrate trends and changes by putting current results in context by contrasting them with benchmarks or historical data.
To engage stakeholders, use the data to tell a story. Describe the implications of the findings for the organization, including any opportunities, hazards, or areas that require improvement. Adapt your message to suit the needs of various audiences by emphasizing the points that will have the biggest influence on each group inside the company. To make sure everyone is aware of and in agreement with the next steps based on the data analysis, ask for input.
8. Real-world Applications
Imagine a situation in marketing when a business launches an advertising campaign that leads to a statistically significant rise in website visitors. But when they dig deeper into the statistics, they find that the traffic bump was not actually accompanied by any sales or conversions. This emphasizes how crucial it is to look beyond statistical significance in order to ascertain the true impact on organizational goals.
Consider a scenario in finance where a backtesting or simulation environment produces statistically significant returns from an investing strategy. Even with these encouraging statistical findings, the strategy's failure to produce predicted gains in real market conditions is mostly attributable to unanticipated market dynamics or outside influences. Had we known the business relevance, we may have avoided taking unnecessary risks based only on statistical results.
Let's say a new medication in the medical field goes through clinical trials and demonstrates statistically significant gains in treating a certain ailment. But it soon becomes clear from a closer look at patient outcomes and quality of life metrics that the drug's advantages do not outweigh its drawbacks or possible adverse effects. Here, the business significance is in evaluating the real-world effects of statistical findings on patient outcomes and the effectiveness of the healthcare system.
9. Future Trends and Challenges
The link between statistical significance and business relevance is probably going to change significantly as data analytics progresses. The emergence of advanced technologies like artificial intelligence (AI) and machine learning will provide enterprises with greater access to sophisticated tools capable of extracting insights from intricate datasets. This could result in a more sophisticated comprehension of the relationship between statistical results and real-world business effects.
Predictive analytics may become more important as a result of these developments, enabling businesses to foresee trends and take preemptive measures. Businesses can leverage data to find opportunities and manage hazards before they fully materialize by going beyond simple descriptive analysis. The interpretation of statistical significance in relation to accomplishing strategic goals may be redefined by this move toward predictive modeling.
But these prospects also present difficulties in terms of successfully coordinating statistical insights with strategic goals. Making sure that the measurements used to assess statistical significance closely match the KPIs that determine business success is one of the main challenges. If the objectives of the business and the analytical outputs are not aligned, organizations may have trouble turning statistical findings into workable plans.
The increasing complexity of data analytics raises the possibility of information overload and analytical paralysis. Companies need to find a balance between delving deeply into analysis for solid insights and staying away from unimportant elements that don't really affect their bottom line. Therefore, bridging the gap between statistical significance and economic impact will require investment in efficient data visualization technologies and open lines of communication.
While data analytics innovations have the potential to open up a wide range of commercial opportunities, companies also need to be aware of the difficulties in using statistical insights to inform strategic decision-making. Companies may put themselves ahead of the curve in adopting data-driven strategies for sustainable growth in a more competitive environment by proactively tackling these issues and adopting a comprehensive strategy that combines statistical rigor with business savvy.
10. Conclusion
The aforementioned leads us to the conclusion that it is critical to realize that business relevance does not always equate to statistical significance. Even while statistical analysis is essential for deriving conclusions from data, it's also critical to take into account the findings' practical applications in a corporate environment and the larger context. P-values and confidence intervals should not be the only considerations in business decisions; insights from customer behavior, market trends, subject expertise, and other qualitative factors should also be taken into account.
Decision-makers need to take a comprehensive approach that incorporates both statistical rigor and practical relevance in order to avoid making the mistake of confusing statistical significance with business value. This entails being aware of the limitations of statistical testing, concentrating on pertinent KPIs that support organizational objectives, and taking choices' long-term effects into account in addition to their immediate effects. Organizations may make better decisions that lead to significant outcomes and long-term growth by fusing quantitative analysis and qualitative insights.
In order to make decisions that are both statistically legitimate and strategically good for the overall business objectives, it is necessary to strike a balance between the practical and statistical data. Businesses can more skillfully negotiate complexity, uncertainty, and ambiguity by adopting this holistic strategy, which will help them produce significant outcomes that will give them a competitive edge in the fast-paced market of today.