1. Introduction to Retail Pricing and Machine Learning
In the retail industry, a company's ability to set prices effectively determines its success. In order to maximize earnings and maintain competitiveness, retailers must continually adjust their prices. In the shifting market conditions of today, traditional pricing approaches frequently prove to be inadequate.
Retailers trying to maximize their pricing strategies have access to a potent tool in machine learning. Retailers may decide on prices more intelligently by using algorithms that can quickly and efficiently assess large amounts of data based on competition prices, customer behavior, seasonality, and more.
In this blog post, we'll examine how machine learning is transforming retail pricing tactics and boosting profits for companies that adopt this cutting-edge technology. We will examine the advantages of using machine learning to retail pricing and offer suggestions on how companies may make the most of these cutting-edge resources to remain competitive in today's market.✌️
2. Understanding the Basics: What is Machine Learning in Retail Pricing?
In retail pricing, machine learning is a data-driven methodology that uses algorithms to evaluate massive amounts of historical and current data in order to forecast customer behavior, enhance pricing tactics, and increase revenue. Retailers may find patterns, trends, and insights with machine learning models that are practically hard for humans to find through manual means. Retailers may now dynamically modify prices in response to a range of factors, including demand, competition, seasonality, and customer preferences, thanks to this technology.
Dynamic pricing is a popular way that machine learning is used in retail pricing. With this method, pricing for goods or services are set flexible according to the state of the market at any given time. Retailers can formulate the best rates to optimize revenue while maintaining market competitiveness by taking into account variables such as inventory levels, rival pricing, and historical sales data. These characteristics can be continuously analyzed by machine learning algorithms, which can then alter prices in real time to take advantage of shifting market dynamics.
Retailers can also use machine learning to implement customized pricing plans. Retailers can customize rates for individual consumers by using customer data, including purchase history, browsing habits, demographics, and even psychographic traits. This degree of customization boosts consumer loyalty and engagement in addition to improving the entire buying experience. In order to effectively offer targeted discounts or promotions, machine learning algorithms are essential for identifying relevant client segments and forecasting price sensitivity.
To put it simply, machine learning for retail pricing gives companies the power to decide on price points with greater knowledge. In today's fast-paced retail climate, retailers can stay ahead of the competition and satisfy the constantly changing demands of consumers by automating the analysis of massive amounts of data and delivering actionable insights in real-time.📚
3. Benefits of Implementing Machine Learning in Retail Pricing Strategies
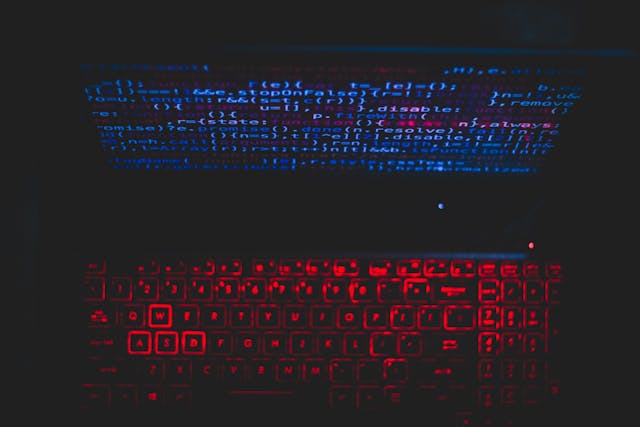
There are several advantages to integrating machine learning into retail pricing strategies, which can provide companies with a competitive advantage in the marketplace. The capacity to swiftly and precisely evaluate enormous volumes of data is a key benefit. With the help of machine learning algorithms, one may optimize pricing decisions by analyzing rival pricing, past sales data, customer behavior, and a variety of market trends. 📏
Retailers can now tailor their pricing tactics depending on the unique interests and behaviors of each customer thanks to machine learning. Retailers can enhance sales and customer loyalty by offering targeted discounts or promotions through the use of algorithms that can classify customers into different categories or forecast their purchase tendencies.
The capacity of machine learning to instantly adjust retail prices to shifting market conditions is another important advantage. Retailers may make sure they stay competitive and optimize profits by having the ability to automatically modify prices based on variables like inventory levels, demand fluctuations, or competitor actions.
Price elasticity for various products or client segments can be identified via machine learning, which can aid in the optimization of pricing strategies. This makes it possible for merchants to carefully set pricing, optimizing income and preserving their position as market leaders.
Incorporating machine learning into retail pricing strategies improves decision-making procedures and helps companies remain flexible and adaptable in the fast-paced retail landscape of today.
4. Common Challenges and Pitfalls in Using Machine Learning for Retail Pricing
Using machine learning for retail pricing might present a number of typical problems and hazards. Data complexity is one of the main obstacles. Large volumes of data, such as sales history, client demographics, rival pricing, and seasonal patterns, are frequently possessed by retailers. It can be difficult to manage and interpret this data in order to get insightful information.
Accurate data labeling is a further problem. For supervised learning to work, the model needs labeled training data. Large dataset labeling, however, can be costly and time-consuming. Pricing decisions may be impacted by biased or erroneous assumptions resulting from poorly labeled data.
One common mistake in machine learning for retail pricing is overfitting. When a model works well on training data but is unable to generalize to fresh, untried data, this is known as overfitting. This may result in pricing tactics that are not as effective as they may be in practical situations.
Finally, it can be difficult to interpret and communicate machine learning models to stakeholders. specific models are intricate "black boxes," making it challenging to comprehend how they determine specific suggested prices. Getting the support of decision-makers becomes difficult in the absence of interpretability and transparency.
Strong subject expertise, reliable data preprocessing methods, cautious model selection, and successful stakeholder engagement strategies are all necessary to overcome these obstacles. Retailers may leverage machine learning to optimize pricing strategies and propel business growth by tackling five typical issues head-on.
5. Best Practices for Successful Implementation of ML in Retail Pricing
To guarantee a successful integration of machine learning in retail pricing, there are a number of recommended approaches. First and foremost, establish a specific goal, be it increasing customer satisfaction, optimizing inventory turnover, or maximizing profitability. To properly train your models, next collect high-quality data from multiple sources, such as sales history, market trends, and customer behavior.
Selecting the appropriate algorithms to meet your unique pricing requirements is essential. Your retail pricing strategy's performance will be greatly impacted by the tools you choose, whether it's clustering algorithms for market segmentation or regression models for price elasticity analysis. Update and retrain your models often to keep up with shifting consumer and market trends.
Combine human knowledge with machine learning skills. Although machine learning (ML) is capable of processing large volumes of data quickly, human judgment is still necessary for understanding the results and formulating strategic decisions based on the insights the models provide. Before implementing your ML models on a large scale, make sure they are accurate and reliable in real-world situations by conducting extensive testing and validation.
Finally, keep a close eye on key performance indicators (KPIs) to assess how well your ML-powered pricing plan is working. Based on the feedback from KPIs, adjustments could be required to fine-tune your strategy and get the best outcomes in boosting profitability and competitiveness in the retail industry.
By following these best practices diligently, retailers can harness the power of machine learning to enhance their pricing strategies effectively and stay ahead in today's dynamic marketplace.
6. Case Studies: Real-World Examples of ML Transforming Retail Pricing
Case studies provide insightful information about how machine learning affects retail pricing tactics in the real world. A notable instance may be seen in the use of ML algorithms by a well-known fashion shop to optimize pricing in real-time according to variables such as inventory levels, rival price, and customer demand. They were able to set prices dynamically by utilizing predictive analytics, which raised sales and profitability.
A major grocery chain that used machine learning models to examine past sales data and consumer behavior is the subject of another case study. The business was able to boost consumer loyalty, strategically modify prices, and push personalized offers by gaining insight about buying trends and preferences. Customer satisfaction and revenue generation both increased as a result of this data-driven strategy.
By using ML algorithms to forecast price elasticity and maximize markdowns, an online electronics merchant enhanced its pricing strategy. The store was able to maximize revenues on high-demand items while avoiding costly price reductions on slow-moving inventory by precisely predicting how customers would react to various pricing tactics. With this dynamic pricing strategy, their market competitiveness was greatly increased.
The observable advantages of incorporating machine learning into retail price choices are illustrated by these case studies. Retailers can respond quickly to changes in the market, satisfy customer expectations, and promote sustainable growth in a highly competitive sector by utilizing data-driven insights and predictive analytics.
7. Optimizing Dynamic Pricing Strategies with Machine Learning Algorithms
Machine learning algorithms' capacity to optimize price decisions in real time has led to a notable surge in the adoption of dynamic pricing methods in the retail industry. Retailers can dynamically modify prices based on a range of criteria, including demand, competition, and customer behavior, by utilizing sophisticated algorithms. This enables retailers to set the right price at the right moment in order to optimize earnings.
Massive data sets can be analyzed by machine learning algorithms, which can also identify patterns that human analysts would miss. Because retailers can now forecast customers' reactions to various price points and modify prices accordingly, they are able to make more informed pricing decisions. Machine learning-driven dynamic pricing makes sure that prices are constantly reasonable and representative of the market.
The capacity to automate the pricing process is a crucial component of using machine learning to optimize dynamic pricing strategies. In order to make modifications in real time, machine learning algorithms can continuously evaluate competition prices, market trends, and customer behavior. While keeping prices profitable and competitive, this automation gives merchants back significant time to concentrate on other critical elements of their business.
Retailers can use dynamic pricing strategies with machine learning algorithms as a valuable tool to remain ahead of the competition in today's fiercely competitive industry. Retailers may enhance their pricing strategies, increase profitability, and provide customers individualized price solutions by utilizing machine learning's predictive powers.
8. Ethical Considerations in Utilizing AI for Retail Pricing Decisions
Ethical considerations are critical to guaranteeing justice and openness when using AI to determine retail prices. Retailers need to be aware that machine learning algorithms may contain biases that result in unfair pricing practices. Regular auditing and monitoring of the algorithms is necessary to avoid unforeseen repercussions that could negatively impact customers or unfairly disfavor specific groups.
When utilizing AI for pricing tactics, transparency is essential to earning the trust of consumers. Retailers should make sure that pricing judgments are explicable and justified, and they should make it apparent to customers how AI is used to determine prices. AI-driven price disputes and complaints should be handled through established channels, giving customers a way to get justice if they feel mistreated.
When using AI for retail pricing, protecting data privacy is yet another crucial ethical factor to take into account. Retailers are responsible for managing consumer data, making sure that data protection laws are followed, and protecting private information from security breaches. Maintaining ethical standards in retail AI implementations requires being open and honest about data gathering methods and getting customers' express agreement before using their data.
It is the duty of retailers to respect moral principles when using AI to determine prices. Through the prioritization of justice, transparency, and data privacy, organizations can effectively leverage the potential of machine learning, establish consumer trust, and cultivate enduring partnerships founded on integrity and respect. 🔆
9. Future Trends and Innovations in Machine Learning for Retail Pricing
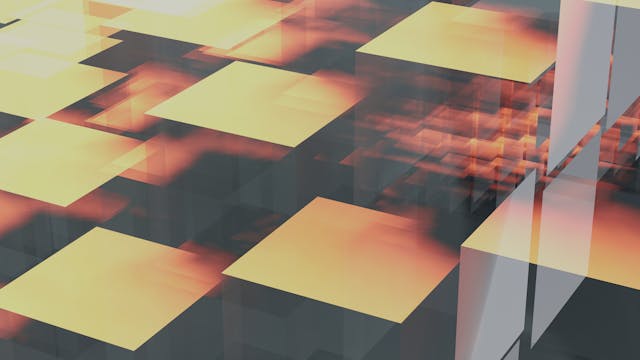
The method that businesses set prices is about to undergo a revolution thanks to upcoming trends and advancements in machine learning for retail pricing. The growing integration of AI-driven dynamic pricing models, which enables merchants to modify prices in real-time depending on a variety of factors like demand, competition, and even consumer behavior, is one of the major developments. Maintaining market competitiveness while maximizing profitability is possible with this degree of automation and optimization.
Pricing strategy optimization and customer demand forecasting will continue to be greatly aided by machine learning algorithms-powered predictive analytics. Retailers can set prices that satisfy customer expectations and optimize revenue by examining historical data and recognizing patterns. Retailers are able to keep ahead of market trends and make proactive strategy adjustments because to this advanced forecasting capabilities.
Customizing pricing models using machine learning algorithms to meet particular business demands is another new trend. Retailers can use these tailored solutions to develop price plans that are specifically targeted to their target market, increasing consumer loyalty and satisfaction. Retailers will be able to refine their pricing plans by extracting important information from social media data and customer comments thanks to advancements in natural language processing (NLP) and sentiment analysis.
We anticipate more developments in machine learning technologies for retail pricing in the near future. One such development is the application of reinforcement learning algorithms for adaptive pricing strategies that adapt and change in response to real-time feedback. Retailers will be able to provide more customized product recommendations with dynamic pricing alternatives based on individual preferences thanks to improved picture recognition and recommendation algorithms.
Machine learning has a lot of potential to improve profitability, competitiveness, and consumer satisfaction in retail pricing in the future. Retailers who use these cutting-edge technology will have a major advantage in a market that is changing quickly due to data-driven insights and clever decision-making techniques.
10. Implementing Predictive Analytics for Better Price Optimization
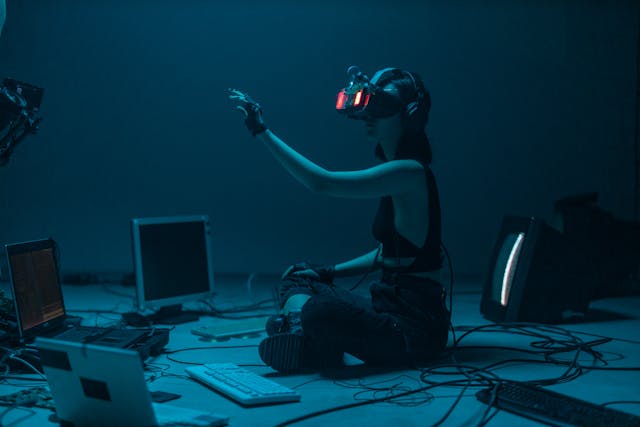
The way that companies optimize their prices can be completely changed by incorporating predictive analytics into retail pricing. Retailers may forecast consumer behavior, competing pricing tactics, and market trends by utilizing machine learning algorithms to evaluate enormous volumes of data. They are able to maintain their competitiveness and set ideal prices that optimize profitability.
Accurate demand forecasting is a major advantage of predictive analytics. Retailers can more accurately estimate future demand by examining previous sales data, seasonality, and external factors like weather patterns or economic situations. This enables them to dynamically modify their prices in order to satisfy customer demand without overpricing or understocking.
Retailers can use dynamic pricing strategies based on real-time data by utilizing predictive analytics. Retailers may quickly change their prices to keep ahead of the market and take advantage of new trends by regularly analyzing competitor prices, consumer attitude, and other factors. In today's fast-paced retail industry, when market conditions are always shifting, this flexibility is essential.
Predictive analytics for price optimization, in its simplest form, empowers merchants to make data-driven choices that maximize profits and improve customer satisfaction. Businesses can obtain a competitive edge in the market by providing the right items at the right price points, optimizing revenue and customer happiness concurrently, by utilizing the power of machine learning and predictive modeling.
11. Leveraging Big Data to Enhance Retail Pricing Strategies
Using big data to improve strategies is revolutionary in the retail price space. Through the utilization of extensive data on consumer behavior, industry trends, and rival pricing, merchants can acquire insightful knowledge that facilitates better decision-making. This data is sorted through by machine learning algorithms, which then use the results to spot trends, forecast customer preferences, and instantly improve pricing tactics.🤭
Retailers can learn about seasonality trends and purchase behaviors by analyzing past sales data. Retailers may more precisely predict demand and dynamically modify pricing by merging this data with outside variables like sentiment research on social media or economic statistics. Retailers can remain flexible in reacting to shifts in the market and pressure from competitors by taking a proactive strategy.
Retailers can tailor their pricing plans depending on the unique interests and purchase habits of their customers with the use of big data analytics. Retailers can give customized pricing discounts that resonate with particular demographics or buying behaviors by segmenting their client base into micro-targeted groups. For retail firms, this degree of personalization increases total sales and profitability while also improving consumer pleasure.
Retailers may improve their pricing strategies by studying customer behavior, properly forecasting demand, maintaining market competitiveness, and providing individualized pricing incentives by utilizing big data and machine learning. Using these technologies gives retailers a major competitive edge in the ever-changing retail market of today.
12. Measuring Success: Key Metrics and KPIs for Evaluating ML-driven Retail Pricing
A number of important measures and KPIs can offer insightful information about how well machine learning-driven retail pricing methods are working. **Revenue growth** is a crucial indicator that quantifies the total revenue gain attributable to the use of ML pricing models. **Profit margins** are yet another important metric that shows how well pricing tactics maximize earnings.
Monitoring **retention and acquisition rates** can provide a comprehensive understanding of how ML-driven pricing affects consumer behavior. Retailers can determine whether their pricing strategies are successful in drawing in new customers while keeping hold of their current clientele by examining changes in these rates after implementation.
Furthermore, when evaluating the degree to which demand is responsive to price fluctuations, **price elasticity** is an essential KPI. Retailers can optimize their pricing strategy for optimum profitability by utilizing ML-driven pricing models to gain a better knowledge of price elasticity.
**Basket analysis** offers additional metrics along with insights into how customer buying habits have changed when dynamic pricing was implemented. Tracking variations in shopping basket size, purchase frequency, or cross-selling efficiency can demonstrate how well machine learning algorithms influence consumer spending and engagement.
Retailers may obtain a thorough grasp of how machine learning affects their pricing strategies and make data-driven decisions to continuously enhance their strategy for sustainable development and profitability by regularly tracking and evaluating these important indicators and KPIs.