1. Introduction
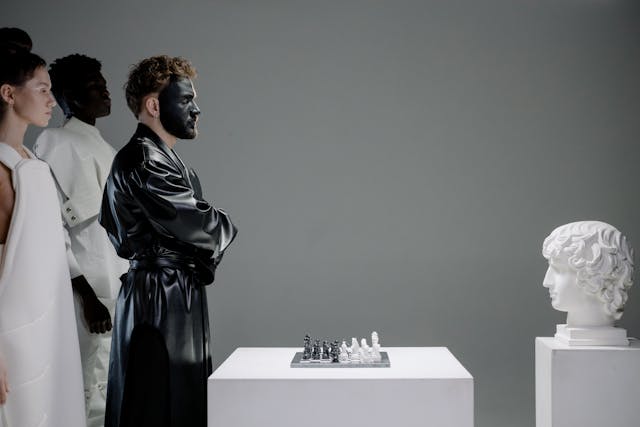
The idea of "self-service data" enables consumers to access and manipulate data without the need for sophisticated technical knowledge or IT support. It seeks to democratize data inside businesses by enabling people from different departments to independently research and evaluate data in order to make wise decisions. This method is frequently praised for encouraging flexibility, creativity, and effectiveness by lowering dependency on central IT teams for standard data chores.
Self-service data is appealing because it allows business users to easily change and understand data as needed, leading to faster insights and actions. It facilitates speedier decision-making processes, improves team collaboration, and fosters a culture of data-driven decision-making by providing a direct gateway to organizational data assets. Because of this, self-service data has been incredibly popular in recent years as businesses try to adjust to the ever-faster-paced, data-centric corporate climate.
2. The Concept of Empowerment
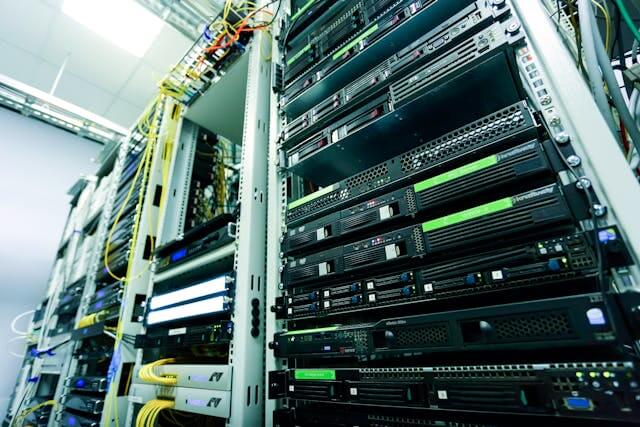
Self-service data is a concept that is frequently presented as empowering consumers by letting them access and analyze data on their own without the help of IT or specialist teams. This autonomy points to the democratization of knowledge, allowing people to freely investigate and draw conclusions on their own, so creating a sense of control over how they use data. Businesses promote self-service tools as a way for staff members to make decisions more quickly using real-time data and tailor analysis to meet their own requirements.
But it begs the question of whether this empowerment is a front or not really realized. Self-service data tools provide users some autonomy and flexibility, but they can also make it difficult for them to navigate large, complex datasets, understand outcomes accurately, and maintain data security and compliance. Unintended effects like siloed information ecosystems, where different departments produce isolated studies devoid of comprehensive perspectives, could result from the temptation of independence.
It can be deceptive to assume that every user has the necessary abilities and subject-matter expertise for efficient data analysis. In actuality, not everyone possesses the knowledge and experience to make the best use of these tools or the awareness to identify biases in the interpretation of data. This calls into question the dependability and veracity of insights obtained via self-service platforms, thereby compromising the main goal of making well-informed decisions.
In the pursuit of leveraging data-driven insights, enterprises must critically assess whether self-service solutions actually empower users or unintentionally spread false information and perform ineffective analyses. Realizing the full potential of self-service data while minimizing its drawbacks requires striking a balance between autonomy and accountability, offering sufficient training and assistance, and putting in place strong governance procedures. Through questioning empowering assumptions and proactively addressing its constraints, companies may foster a responsible data usage culture that respects individual liberty and group intelligence.
3. Accessibility vs. Accuracy
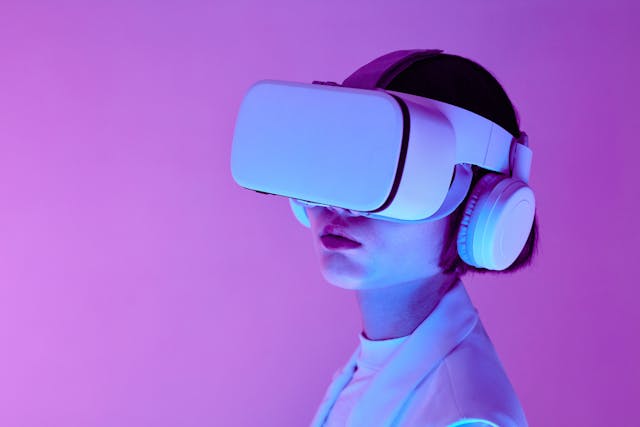
Accuracy and accessibility are crucial trade-offs in the world of self-service data. Although having quick access to data enables consumers to make well-informed decisions, assuring its accuracy can be difficult. Self-service systems face many challenges in maintaining data integrity because of things like inconsistent data sources, human error, and a lack of centralized control. Even though instantaneous data retrieval is convenient, sacrificing accuracy for accessibility might result in expensive mistakes and biased conclusions. For businesses utilizing self-service data solutions, maintaining data accuracy while facilitating easy access is crucial.
4. User Skills and Knowledge
One important premise that is frequently made in the context of self-service data is that users have the necessary abilities to appropriately analyze the data. This presumption, though, has two drawbacks. Self-service tools are meant to empower users, but they also make an assumption about a particular level of data literacy, which isn't always the case. Users who lack a strong foundation in data analysis may misunderstand the data or come to the wrong conclusions.
In order to close the gap between user abilities and the requirements of self-service data, education and training are essential. In addition to providing users with the technical know-how to use self-service capabilities, proper training instills fundamental principles of data literacy. In order to efficiently navigate complicated datasets, ask pertinent questions, and make defensible judgments based on their results, users must be equipped with this knowledge.
Optimizing the advantages of self-service data depends on giving users opportunity for ongoing education. Training courses designed for varying degrees of expertise can boost users' self-assurance in managing data on their own while guaranteeing correctness in analysis and interpretation. Organizations can fully utilize self-service data and cultivate a culture of data-driven decision-making at all organizational levels by funding comprehensive education programs.
5. Data Governance and Control
Self-service data settings require careful consideration of data governance and control. Self-service by its very nature suggests a degree of flexibility and freedom in data access and manipulation, which can give rise to worries about upholding security, compliance, and order. Companies need to find a way to balance giving people self-service options while making sure data is still managed in compliance with laws and regulations.
It is imperative to create explicit policies and procedures regarding data governance in the self-service context in order to successfully handle these risks. This entails establishing procedures for monitoring usage and enforcing compliance, as well as defining roles and duties, data access limits, and data quality measures. Organizations can reduce the risks of unauthorized access, data breaches, and regulatory noncompliance by cultivating an environment of accountability and openness.
Ensuring the integrity of data on self-service platforms requires the implementation of strong security measures. To prevent unwanted behaviors, this entails implementing technologies like role-based access restrictions, authentication methods, and encryption. Sensitive information can also be anonymized while maintaining its usefulness for analytics by using data masking techniques. Organizations can take proactive steps to fortify their defenses by identifying vulnerabilities and holes in security policies through regular audits and assessments.
A strategic approach is needed to strike a balance between granting self-service capabilities and retaining control over data. Using policy-driven automation technologies to enforce data governance policies in real-time based on predetermined criteria is one tactic. These solutions can ensure that data usage complies with company standards and legal requirements while streamlining decision-making processes. Offering thorough training courses on data governance best practices enables users to use self-service tools responsibly and make educated judgments.
Furthermore, as I mentioned previously, it is not impossible to resolve the seeming conflict between the ease of self-service and strict data control. Organizations can build a strong framework that guarantees compliance and security without impeding innovation or agility by utilizing technological solutions, defining clear policies, encouraging a culture of responsibility among users, and offering sufficient training. Unlocking the full potential of data-driven decision-making in today's fast-paced digital environment requires embracing the changing self-service data landscape while taking precautions against potential hazards.
6. Impact on Decision-Making
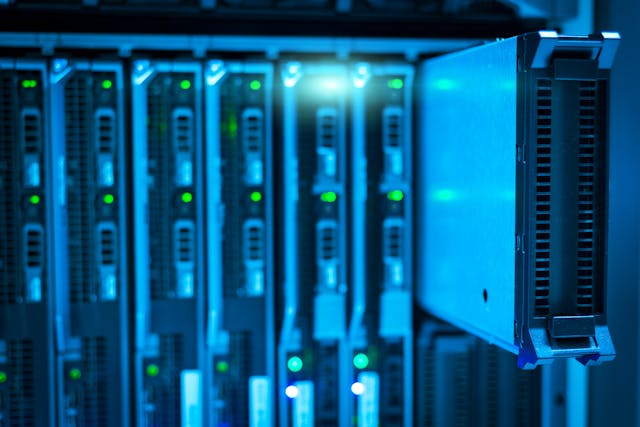
Organizational decision-making processes have undergone a substantial transformation thanks to self-service data technologies. With the use of these technologies, consumers may access, examine, and draw conclusions from data without heavily depending on IT or data experts. Self-service data solutions facilitate agility by providing faster access to information, enabling users to make decisions based on real-time data more quickly.
There are advantages and disadvantages to the decentralization of data access via self-service technologies, though. Decentralization has the potential to enhance creativity and productivity by enabling workers from different departments to examine data on their own and find insights that are pertinent to their positions. This decentralized strategy can promote cooperation and dismantle organizational silos.
However, there can be dangers involved with decentralizing data access. There is a chance that various people or teams will analyze the same dataset inconsistently or inaccurately if there is inadequate control and oversight. As a result, judgments may be made based on inaccurate information and contradicting findings.
In order to reduce the dangers associated with decentralized data access, businesses must set up clear policies, governance frameworks, and training initiatives. Self-service data tools are a great way to democratize data access and speed up decision-making processes. To optimize the beneficial effects of self-service technologies on decision-making in an organization, control and autonomy must be balanced. 🔶
7. Bias and Misinterpretation
When it comes to self-service data analysis, bias might pose a serious risk of producing inaccurate results and decisions. By making erroneous assumptions, selecting particular facts, or misinterpreting information, users may inadvertently induce bias. Organizations can reduce prejudice by enforcing stringent data governance procedures, offering instruction on efficient data analysis methods, promoting cooperation among analysts from various backgrounds, and deploying automated systems that can identify and rectify biases instantly.
Encouraging a culture of critical thinking and skepticism among users performing self-service data analysis is necessary to promote correct interpretations. Biased interpretations can be lessened by encouraging people to challenge their presumptions, validate findings using a variety of sources or viewpoints, and ask peers for comments. Establishing unambiguous protocols for data interpretation and validation can help organizations guarantee the dependability and credibility of insights obtained from self-service analytics. Through proactive measures to remove bias and the promotion of best practices in data analysis, organizations can optimize the benefits of self-service data platforms while reducing the potential for misinterpretation.
8. Collaborative Data Culture
Achieving a balance between stakeholder involvement and self-service capabilities is critical to developing a collaborative data culture. Although self-service solutions enable individuals to access and analyze data on their own, actual effectiveness frequently arises from varied teams working together and pooling their combined wisdom. Through the integration of autonomy and teamwork, businesses can fully utilize their data resources.
Stressing the importance of teamwork while using data highlights the importance of different viewpoints and levels of experience coming together to achieve common goals. The ability to solve problems is enhanced by collective intelligence, which stimulates creativity and well-informed decision-making. Encouraging open communication channels and establishing a culture that encourages cooperation boosts every stakeholder's contribution to deriving useful insights from data sets.
Success in the ever-changing business environment of today depends not only on individual skills but also on how well they function together in a team environment. Organizations may create a competitive edge based on collective intelligence at its foundation and better insights and more informed decisions by promoting a culture that values autonomy and teamwork in data practices.
9. Tools for Validation and Verification
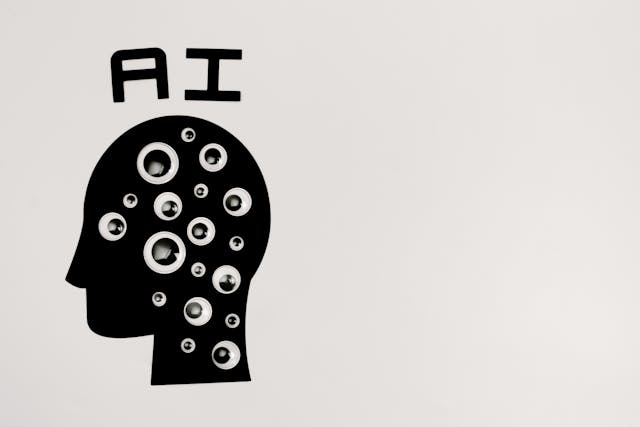
Within the domain of self-service data platforms, validation and verification technologies are essential for maintaining the accuracy of insights obtained from these platforms. These instruments act as protectors of precision and dependability, guaranteeing that conclusions are dependable and genuine.❗️
Data profiling software is one such tool that lets users look at the features and quality of their datasets. Through the process of detecting and resolving anomalies, missing values, and inconsistencies in the data, users may ensure that their analyses remain legitimate.
Metadata management systems are another useful tool for tracking the provenance and source of material. This increases the transparency and reproducibility of analysis processes by allowing users to track back the generation of certain findings.
Algorithms for detecting anomalies can automatically identify odd patterns or outliers in data, warning users of possible mistakes or discrepancies that need more research. These methods improve the reliability of results obtained from self-service data platforms by proactively detecting abnormalities.
These validation and verification technologies essentially serve as gatekeepers for data integrity, strengthening the basis upon which important business decisions are based. Organizations may rely on the dependability and credibility of the insights obtained from self-service data platforms with their help.
10. Transparency in Data Sources
To maintain integrity and reliability, self-service systems must have transparent data sources. Organizations must reveal the sources of their data since users depend on reliable information to make decisions. Make it obvious where the data originates from, how it is gathered, and any changes made to it in order to preserve openness. Provide thorough explanations of the credibility and recentness of data sources so that consumers may determine the applicability of the information. Organizations can gain credibility by being transparent about their data sources and provide users with reliable information.
11. Continuous Improvement and Innovation
Optimizing the utilization of self-service data necessitates constant innovation and improvement. Adopting a continual improvement mindset enables firms to maintain their leadership in the efficient use of self-service data. Organizations can guarantee that their personnel are always at the forefront of data use by supporting continuous training initiatives, updating tools on a regular basis, and cultivating an attitude that welcomes learning from both achievements and setbacks.
Organizations should investigate new technologies like artificial intelligence (AI) and machine learning to automate and streamline data operations in order to reinvent their approach to gaining the most benefits from self-service data. Utilizing sophisticated analytics methods such as natural language processing or predictive modeling can effectively extract insightful information from large datasets. Developing a culture of data-driven decision-making and fostering cross-functional departmental collaboration can inspire creative solutions and tactics for utilizing self-service data in fresh ways.
Through consistent strategy refinement, adoption of technical improvements, and cultivation of a collaborative and learning culture, enterprises may effectively unleash the complete potential of self-service data and pioneer game-changing innovations within their respective sectors.
12. Conclusion: Balancing Autonomy with Responsibility
We can infer from the foregoing that self-service data presents both benefits and drawbacks from the conversation around it. Giving users more autonomy has the potential to boost productivity and creativity, but it also carries some hazards, such erroneous insights and security lapses. Effective use of self-service data requires finding a balance between maintaining organizational accountability and giving users independence.
To manage access controls, guarantee compliance, and keep an eye on data quality, organizations need to put strong governance structures in place. Businesses can encourage an accountable culture and enable users to handle data on their own by offering sufficient training and assistance. To fully profit from self-service data without compromising integrity or security, it is imperative to find this balance.