1. Introduction
Businesses are increasingly using data science to make educated decisions and obtain a competitive edge in today's data-driven environment. Data science is the analysis of large, complicated data sets to find important patterns and insights that can inform strategic choices. Businesses can turn raw data into usable information by utilizing methods like machine learning, statistics, and data visualization. This leads to increased customer understanding, enhanced operations, and overall corporate success.
Making decisions based on data has become essential for companies trying to stay competitive in today's fast-paced marketplace. Companies are now embracing advanced analytics and predictive modeling in place of intuition or historical trends alone to predict client requirements, optimize operations, and spot new growth prospects. With the abundance of data available today, companies may use data science to reduce hazards, increase prediction accuracy, and ultimately make more successful decisions by reducing risk.
In the current digital world, integrating data science into corporate operations is not only a trend but also a need. Companies that use data-driven decision making are in a better position to surpass rivals, meet changing customer expectations, and adjust to shifting market dynamics. We will go into more detail about data science's usefulness for companies in this blog article, as well as how this revolutionary area can open up new avenues and promote long-term success in today's corporate environment.
2. Key Components of Data Science
Key Components of Data Science:
The first stage of data science is data collecting, which entails obtaining information from a variety of sources, including social media platforms, databases, and sensors. This procedure guarantees that the data accessible for analysis is reliable and pertinent.
Analysis is where the acquired data is evaluated using statistical tools and algorithms to uncover patterns and trends. This process aids in the extraction of insightful information from the data so that wise business decisions can be made.
Making meaning of the studied data requires interpretation, which entails interpreting the results in terms of corporate objectives or inquiries. It is essential for drawing practical conclusions from the analysis.
In order to make difficult information easier for stakeholders to grasp, visualization involves displaying the interpreted data in graphical or visual representations like charts, graphs, or dashboards. In an organization, good visualization facilitates decision-making and improves communication.
These key components work together seamlessly in data science to transform raw data into meaningful insights that can drive strategic decisions and improve overall business performance.
3. Benefits of Data Science for Business
Businesses can benefit greatly from data science. Improved decision-making procedures are a key benefit. Organizations can get better results by using machine learning algorithms and data analytics to create data-driven decisions that are more fast and accurate.
Businesses may personalize their services and obtain deeper insights into their customers thanks to data science. Businesses can gain a better understanding of their customers' requirements, interests, and habits by studying customer data. This makes it possible to run more focused marketing campaigns, provide better customer service, and eventually win over more devoted customers.
Increasing operational efficiencies is one of data science's other main advantages. Businesses may increase productivity, cut expenses, and optimize processes by deploying automation and predictive analytics tools. As a result, there is a competitive advantage in the market and operations are optimized.
4. Case Studies of Successful Implementation
Numerous companies have used data science to transform their operations and spur expansion. One famous application is Netflix, which employs data science to assess viewer preferences and behavior to offer personalized content, resulting in greater user engagement and retention. Another excellent example is Amazon, which uses data science and predictive analytics to precisely predict customer wants, optimize pricing policies, and improve the whole shopping experience.
Predictive modeling and preventative care programs have helped the massive healthcare organization UnitedHealth Group enhance patient outcomes through the effective application of data science solutions. Uber uses data science to create dynamic pricing models that adjust to traffic and demand patterns, facilitating effective driver-rider matching and optimizing income.
These case studies show how data science has enormous potential to revolutionize sectors and businesses by fostering innovation, strengthening decision-making procedures, and eventually raising overall performance and competitiveness.
5. Challenges in Implementing Data Science
Although integrating data science into an organization can be a game-changer, there are a number of obstacles to overcome. Ensuring the quality of the data being used is a common obstacle. Inaccurate outcomes and incomplete insights can be caused by poor data quality, which can influence decision-making procedures. To reap the greatest rewards from data science, businesses must devote time and money to making sure their data is accurate, consistent, and clean.
The skills gap that many firms have when implementing data science is another important obstacle. A distinct skill set including proficiency in statistics, programming, machine learning, and domain knowledge is needed for data science. It can be expensive and challenging to find professionals with these multidisciplinary talents. To properly close this gap, businesses might need to spend money on external talent acquisition or training for current staff members.
To overcome these obstacles, a calculated strategy that includes investing in staff upskilling programs to create an adept data science team within the company and implementing thorough data governance procedures to uphold data quality standards are needed. Businesses can fully utilize data science to fuel innovation and gain a competitive edge by taking on these difficulties head-on.
6. Data Science Tools and Technologies
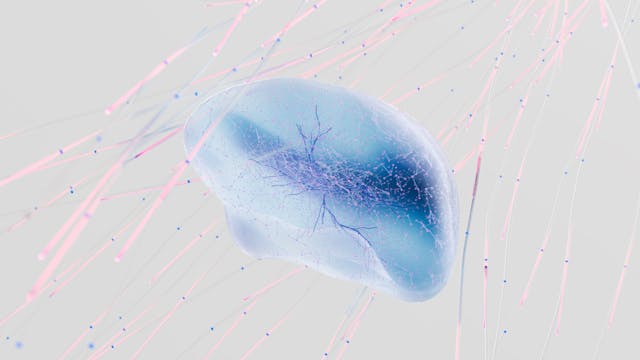
There are several well-liked tools and technologies in the field of data science for business applications that are frequently utilized to extract insights from data. Programming languages like Python and R, which are well-liked and adaptable due to their strong libraries that make activities like data manipulation, statistical analysis, and machine learning easier, are among the most often utilized technologies.
Tools like Tableau, Power BI, and Python's matplotlib are crucial for data visualization and exploration because they help stakeholders comprehend complex findings in a more comprehensible manner. With the use of these technologies, interactive dashboards, charts, and graphs may be created to assist with decision-making based on data-driven insights.
Big data management is made possible by technologies like Spark and Apache Hadoop, which allow distributed processing and storage across computer clusters. These tools are essential for effectively processing and analyzing massive volumes of data, which is especially advantageous for companies working with huge datasets.
Businesses may get a competitive edge in today's data-driven environment by utilizing these well-liked data science tools and technologies to drive innovation, make wise decisions, unearth insightful information, and improve productivity.
7. How to Get Started with Data Science in Your Business
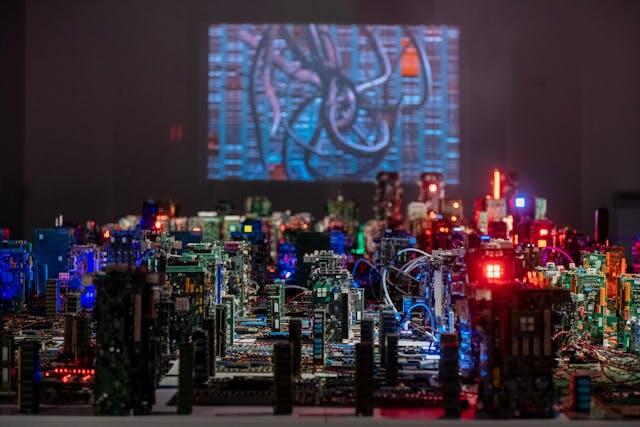
To implement a data-driven culture in your organization effectively, follow these steps to get started with data science:
1. **Teach Your Group:** Start by explaining to your group the value of and potential advantages for the company from data-driven decision-making. Organize workshops or training sessions to acquaint them with fundamental data concepts.
2. Establish definite goals: Identify particular goals and key performance indicators (KPIs) that you wish to enhance through data analysis. Verify that these goals complement your overarching business plan.
3. **Construct Data Structure:** Establish a robust data infrastructure by investing in tools and technology that allow you to collect, store, and analyze data effectively. To handle huge datasets efficiently, think about utilizing cloud services or data management tools.
4. Employ Data Professionals: To spearhead your data projects, appoint experts in analytics, business intelligence, or data science. These professionals can assist you in creating prediction models, algorithms, and useful insights from your data.
5. Promote Data Exchange: Encourage teams to share information and insights among departments in order to cultivate a transparent and cooperative culture. Using a cross-functional approach can result in creative ideas and comprehensive solutions based on group knowledge.
6. **Initiate Little:** To show the usefulness of data science inside your company, start with small-scale or pilot projects. Test many theories, track results, and adjust your strategy in light of criticism and the findings.
7. **Monitor Progress:** To gauge the success of your data-driven efforts, keep a close eye on important metrics and performance indicators. To effectively convey progress and show trends within the organization, use dashboards or reporting systems.
By following these steps, you can lay a strong foundation for implementing data science in your business and creating a culture that values evidence-based decision-making for long-term success.
8. Data Privacy and Ethics Considerations
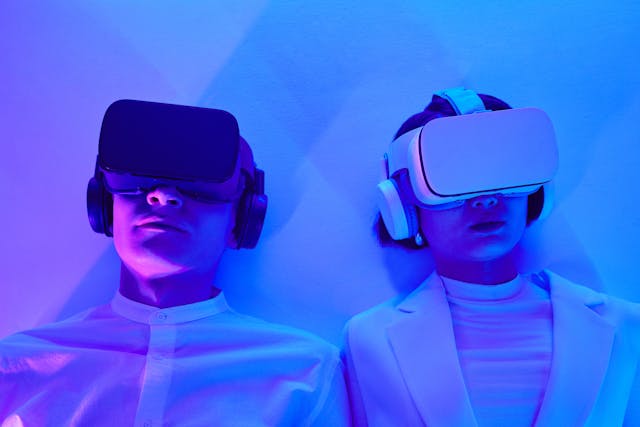
Data privacy and ethics are important issues in the field of data science for corporations. When businesses use data to guide choices, it is critical to make sure that the data is managed properly and morally. Ensuring client privacy, adhering to data security processes, and respecting laws like the GDPR are essential components of maintaining moral principles.
In commercial contexts, using data ethically protects confidential information and fosters consumer trust. Businesses may build enduring relationships with their clients and improve their reputation by putting ethical standards and data privacy first. Following moral principles reduces the possibility of data breaches or misuse, which can have detrimental effects on the business and its stakeholders.
Ensuring that judgments are made with integrity and respect for individual privacy rights is ensured by including ethics into the fundamental framework of data science procedures. Every action, from gathering and evaluating data to implementing algorithms, ought to be dictated by moral standards. In addition to reducing risks, a dedication to data ethics fosters an environment of trust and accountability within a company, opening the door for long-term success in the digital era.
9. Measuring the ROI of Data Science Initiatives
Determining the effectiveness and impact of data science activities on a business necessitates measuring their return on investment (ROI). The ROI of these initiatives can be calculated using a variety of techniques. Comparing the expenses of persons, technology, and other resources needed to accomplish the data science project with the project's benefits is a popular strategy. This could be any number of key performance indicators that show improved results, such as higher revenue, lower expenses, or enhanced productivity.
Using key performance indicators (KPIs) that are closely related to the goals of the data science project is an additional strategy. Businesses can evaluate the value added by comparing the KPIs before and after the project's implementation, based on the data-driven insights that were obtained. In the event that a data science endeavor seeks to enhance marketing initiatives, pertinent key performance indicators may comprise client acquisition expenses, conversion rates, or customer lifetime value.
Measuring the impact of data science projects can be aided by running experiments or A/B testing. Through a comparison of results between groups—one subjected to the data-driven modifications, and another serving as a control group—organizations can explicitly link performance improvements to the use of data science solutions. Isolating the effects of data science from other variables that might affect outcomes creates a more controlled environment for ROI assessment.
When assessing the return on investment (ROI) of data science activities, qualitative input from stakeholders inside the company can be used in addition to quantitative indicators. Employee perceptions of the changes brought about by these programs can offer important insights into areas for improvement or unanticipated advantages that may not be captured by traditional measurements. A thorough understanding of the value of data science for your company may be obtained by combining quantitative and qualitative methods, which also enables you to make well-informed decisions about future data science investments.
10. Future Trends in Data Science for Businesses
Staying ahead of emerging trends is essential for long-term success in the ever changing field of data science for organizations. By integrating artificial intelligence (AI), businesses can improve decision-making, automate procedures, and obtain insightful data. Organizations may forecast trends, anticipate customer behavior, and optimize strategy with the help of predictive analytics. Companies that implement effective big data management are able to efficiently handle, analyze, and use enormous amounts of data for strategic decision-making. Businesses may better traverse the complexity of today's market scenario and promote sustainable growth by adopting these data science future trends.
11. Building a Data Science Team or Partnering with Experts
Effectively utilizing data in your organization requires assembling a strong data science team. When considering developing an in-house data science team, it's vital to outline your needs and understand the skill sets required. Seek candidates with programming, machine learning, statistics, and industry-specific domain knowledge.
Alternatively, by utilizing specific skills and knowledge, collaborating with other specialists might expedite your data science endeavors. Prior to selecting a partner, make sure your project goals are well-defined and evaluate the experience and performance history of possible agencies or candidates. Successful partnerships depend on cooperation and communication, so make sure your goals are aligned from the start.
Building a culture that prioritizes data-driven decision-making is crucial, regardless of whether you choose to work with an internal team or outside specialists. Fostering a culture of ongoing education and creativity inside the group or alliance will result in significant breakthroughs and solutions that will support the expansion of your company. Recall that the human skill utilizing data science's power determines its effectiveness, not only the technologies employed.
12. Conclusion
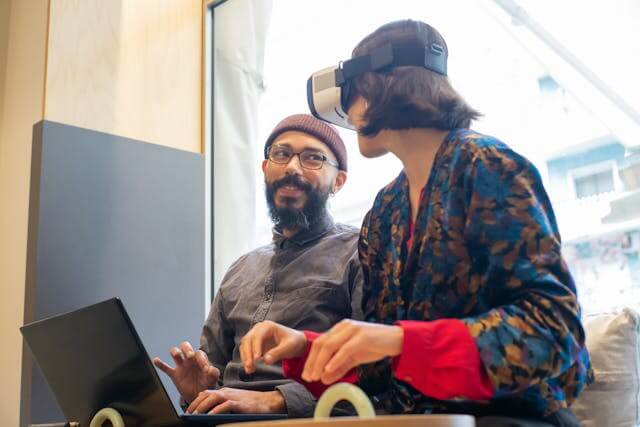
In summary, data science has shown to be incredibly beneficial for companies operating in the current digital era. Companies may increase operational efficiency, improve decision-making, gain a deeper understanding of their clients, foster innovation, and spur growth by utilizing data. Across all industries, the capacity to glean insightful information from massive datasets has transformed business, allowing companies to remain flexible and competitive in a market that is changing quickly.
Businesses can use data science to improve customer experiences, anticipate market trends, streamline operations, reduce risks, and find new revenue streams in addition to personalizing services. Companies can use data science to obtain a noticeable competitive advantage and put themselves in a better position for long-term success if they have the necessary resources and knowledge in place. In today's competitive corporate world, adopting data-driven initiatives is imperative, not optional. 👌
In essence, the effectiveness of data science resides in its power to turn raw information into usable insight that drives strategic decision-making and stimulates innovation. Future developments and innovations that influence the direction of trade in a variety of industries should be anticipated as more companies become aware of the revolutionary potential of data science and make the investment necessary to develop strong analytical skills. A more intricate and interwoven global economy will not only support those who successfully use data, but also reward those who do so.