1. Introduction to AI Data Annotation
Data is frequently referred to as the new oil in the digital age. To realize its full potential, raw data must be tagged and annotated; otherwise, it is insufficient. This is where annotation of AI data becomes useful. AI data annotation is the process of giving machine-readable labels to unprocessed input, such as texts, photos, or videos. It is an essential stage in refining the accuracy and performance of machine learning models through training.
AI data annotation is leading the way in transforming essential business operations in a number of different industries. AI-powered annotation tools are being used in a variety of industries, including healthcare and retail, to improve decision-making, expedite processes, and spur innovation. Businesses may automate tedious operations, lower human error, and speed up the development of innovative solutions by utilizing AI for data annotation.
The foundation for developing strong machine learning models that can quickly and accurately assess enormous volumes of data is laid by AI data annotation. It is impossible to overestimate the significance of precise and effective data annotation as firms continue to digitize and use AI technologies. We will go into greater detail about the importance of AI data annotation and examine how it is changing the face of contemporary business operations in this blog article.
2. Importance of Quality Data Annotation in AI
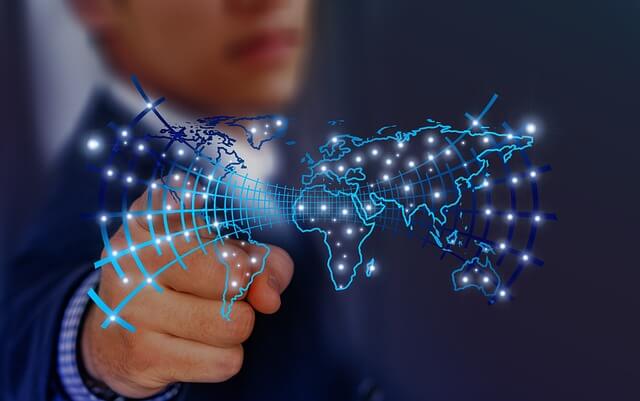
Annotating high-quality data is a vital part of developing AI since it helps train and enhance machine learning models. The process of labeling or tagging data points provides the required context for computers to identify patterns and generate reliable predictions. This is known as data annotation. For AI systems to be reliable and effective in a variety of industries, high-quality annotations are essential.
The effectiveness of AI applications is directly impacted by the precision and applicability of data annotations. Biased models, erroneous forecasts, and untrustworthy decisions might result from inadequate or incorrect annotations. Businesses can improve their AI solutions' accuracy and resilience by investing in high-quality data annotation processes. This will improve results and boost user confidence.
Annotating high-quality data not only enhances model performance but also contributes to the development of more just and moral AI systems. Annotated data contains biases that can spread across machine learning models and provide discriminating results. Therefore, creating AI systems that are impartial and inclusive requires making sure annotations are correct and diverse.
After putting all of this together, we can say that good data annotation improves model accuracy, reliability, and fairness, which is essential for a successful AI deployment. Organizations may unleash the full potential of AI technologies to transform fundamental business processes across industries by giving careful annotation procedures top priority.
3. How AI Data Annotation Impacts Core Business Processes
An important factor in the transformation of key business operations in many different industries is AI data annotation. Organizations may enhance decision-making, expedite processes, and increase accuracy by utilizing AI for data annotation jobs. By using automated annotation methods, businesses can handle massive amounts of data more effectively, cutting down on human error and speeding up the time to insights.
Improved data quality is one important way that AI data annotation affects essential business operations. Businesses may make sure that the labels on their datasets are precise and consistent by using machine learning techniques to tag and annotate data. Better analytics and insights are produced as a result of this enhanced data quality, enabling firms to make defensible decisions.
Annotating AI data facilitates resource optimization for enterprises. By using machine learning models to automate routine annotation chores, firms may free up their team to concentrate on more strategic and complicated duties. This change not only boosts output but also gives workers additional opportunities to add value to the company by working on projects that call for human creativity and intelligence.
Annotating AI data can significantly improve consumer experiences and spur innovation. Businesses are able to create highly customized goods and services that cater to the specific requirements of each individual customer by using precisely annotated data sets. Businesses may conduct personalized marketing campaigns and raise customer satisfaction levels by carefully annotating data to understand consumer preferences.
Because AI data annotation enables businesses to efficiently leverage the value of labeled datasets, it is transforming essential business operations. This technology-driven strategy helps businesses become more competitive in today's digital environment while also improving operational efficiency.
4. Tools and Technologies for Efficient AI Data Annotation
For AI data annotation processes to be effective, tools and technologies are essential. Numerous inventive methods have surfaced to simplify this crucial facet of artificial intelligence advancement. One such solution is the platform Labelbox, which allows teams to work together dynamically and scalable on labeling jobs. Because of its adaptability to multiple data types—such as text, video, and images—it is a preferred option for both organizations and researchers.
Businesses such as Amazon Mechanical Turk provide human intelligence jobs on demand for efficient and economical data labeling. This service gives users access to a big workforce that can quickly annotate large datasets. Advanced algorithms are used by programs like Supervisely to automate annotating chores, minimizing manual work and greatly increasing overall efficiency.
Active learning methods are used with manual annotation on platforms like Explosion AI's Prodigy to continuously optimize the annotation process. By selecting samples for annotation iteratively based on feedback from machine learning models, this integration improves the quality of labeled data. These developments play a key role in retaining the high-quality annotations necessary for reliable model training while speeding up AI development cycles.
The integration of these technologies into AI initiatives has the potential to transform fundamental business procedures by accelerating data labeling assignments while preserving precision and uniformity. The growing array of AI data annotation tools keeps enabling businesses to fully utilize AI across a range of sectors, resulting in unprecedented levels of efficiency and innovation.
5. Challenges Faced in AI Data Annotation and Solutions
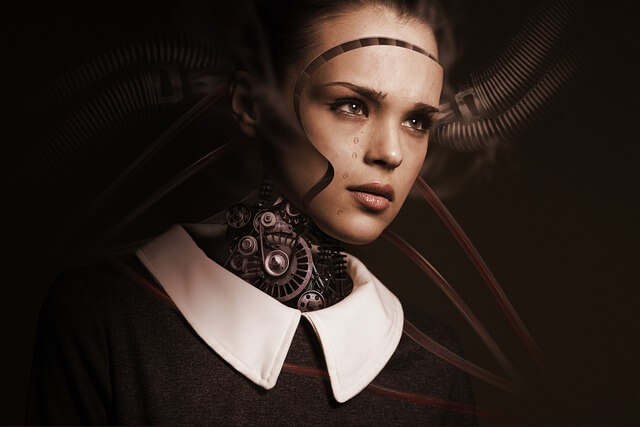
Ensuring quality and accuracy in AI data annotation is one of the main problems. As AI models are trained using annotated data, flaws or inaccuracies can have a substantial effect on the model's performance. Businesses are increasingly using specialized annotation systems with capabilities like automated error detection algorithms, multiple annotator consensus checks, and real-time quality control to lessen this difficulty.
Organizing massive amounts of data that must be annotated in a short amount of time is another difficulty. For companies handling varied data types or complicated datasets, this can be especially intimidating. Choosing scalable annotation solutions that make use of both automation and human annotators can help to expedite the annotation process and guarantee fast turnaround times without sacrificing accuracy.
One major problem in AI data annotation is managing sensitive data while maintaining data privacy and security. To protect data during the annotation workflow, businesses must put strong standards in place. These include stringent access limits, anonymousization methods, and secure storage procedures. Businesses can maintain regulatory compliance and effectively handle these obstacles by cooperating with reputable annotation suppliers who prioritize data security. 🎚
Finally, because unstructured or domain-specific data is complex and lacks common labeling rules, annotating it can be challenging. The task of appropriately annotating such data necessitates domain expertise and specialized knowledge. When dealing with complicated datasets, collaborating with industry experts or utilizing specialized annotation tools catered to certain domains can improve annotation accuracy and maximize model performance.
6. Case Studies: Successful Implementations of AI Data Annotation
Because AI data annotation may improve machine learning algorithms and AI systems, it has revolutionized essential business operations across a wide range of industries. The enormous advantages of AI data annotation are made clear by looking at successful case studies where it has been used successfully.
AI data annotation has been essential in enhancing patient care and diagnosis accuracy in the healthcare industry. AI-powered technologies can help medical personnel discover abnormalities like tumors or lesions more precisely by accurately classifying medical images. This improves treatment planning and overall patient outcomes in addition to quickening the diagnosis procedure.
AI data annotation has transformed personalized shopping experiences and product suggestions in e-commerce. AI systems can offer customized product recommendations by analyzing the browsing habits, past purchases, and preferences of customers. This increases consumer engagement and boosts conversion rates. For e-commerce companies, this degree of customisation not only improves the customer experience but also increases revenue.
AI data annotation plays a key role in autonomous vehicle training, helping algorithms identify and react to different roadside items and situations. By carefully identifying traffic signs, obstructions, and pedestrian movements, AI-enabled cars can safely and effectively navigate complex situations. Ensuring the dependability and security of autonomous driving systems depends critically on the precise annotation of enormous volumes of visual data.
AI data annotation has shown to be quite helpful in financial services for risk management and fraud detection. Through the annotation of patterns suggestive of fraudulent activity in transactional data, artificial intelligence algorithms are able to quickly detect suspicious activity and reduce associated risks. This proactive strategy improves compliance controls and fortifies security procedures in addition to protecting financial institutions from fraud.
These case studies show how established business operations are being transformed by AI data annotation in a variety of industries. Organizations can seize new chances for creativity, productivity, and expansion by using annotated data to train complex AI models. The potential to improve core operations is limitless as long as companies stick to this technology-driven approach to data processing and analysis.
7. Future Trends and Innovations in the Field of AI Data Annotation
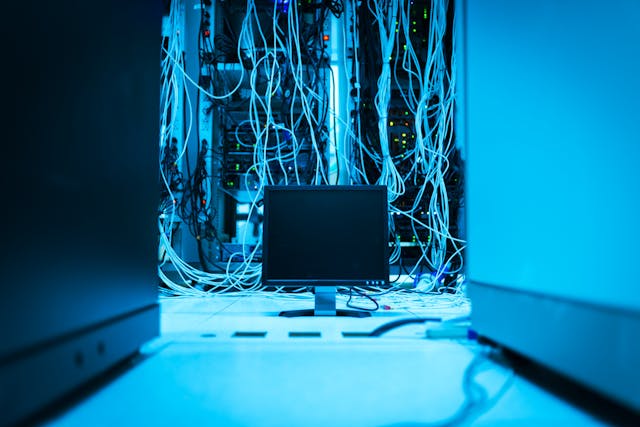
Promising trends and advances in AI data annotation are expected to transform fundamental business operations in the ever-evolving field. The emergence of semi-supervised and self-supervised learning models is one such trend that lessens the need for sizable labeled datasets for training AI systems. Businesses may increase the effectiveness of their AI applications and expedite the data annotation process by utilizing these strategies.
The incorporation of cutting-edge methods into data annotation workflows, such as active learning and reinforcement learning, is another significant breakthrough that lies ahead. With the use of these techniques, AI systems can learn from user feedback iteratively, eventually producing annotations that are more accurate. These methods will become increasingly important as companies look to improve the caliber of their training data in order to guarantee solid and trustworthy AI models.
Dynamic and adaptive data annotation solutions are becoming more and more necessary as real-time insights and personalized experiences become more and more in demand. Businesses may remain ahead of changing consumer tastes and market trends by integrating automatic retraining capabilities and real-time feedback systems into their annotation pipelines. This data annotation agility will spur innovation across industries and enable companies to produce customized solutions on a large scale.
We may anticipate the release of specialized tools and platforms made especially for AI data annotation as AI continues to seep into every facet of corporate operations. Comprehensive capabilities including complex labeling algorithms, collaborative annotation interfaces, and easy integration with current machine learning frameworks will all be available with these tools. Businesses may speed up the creation of AI-powered applications and expedite their data labeling processes by adopting these purpose-built solutions.😐
Businesses all across the world are about to enter a new era of efficiency, accuracy, and scalability thanks to the development of AI data annotation. Organizations may fully utilize artificial intelligence to revolutionize their key business operations and gain a competitive edge in an increasingly digital world by investing in cutting-edge technologies and implementing creative ways to data labeling.