1. Introduction:
The goal of the large subject of computer science known as artificial intelligence (AI) is to build devices or systems that can mimic human intelligence, including learning, problem-solving, and decision-making. A branch of artificial intelligence called machine learning (ML) focuses on creating algorithms that let computers learn from data and make judgments or predictions without explicit programming. Deep Learning (DL) is a subset of machine learning (ML) that models and learns complicated patterns from massive volumes of data by utilizing multi-layered neural networks.
Each of these technologies contributes in a different way to the advancement of computer systems' capabilities. While machine learning (ML) offers methods for processing data including clustering, regression, and classification, artificial intelligence (AI) focuses more broadly on the creation of intelligent systems. Deep learning (DL) uses deep neural networks to uncover complex patterns from large datasets, which makes it an excellent choice for tasks like image identification, natural language processing, and audio recognition. To help readers grasp the differences and applications of AI, ML, and DL in today's technology scene, this blog article will go deeper into their differences.😠
2. Understanding Artificial Intelligence:
Artificial Intelligence (AI) is the term used to describe the modeling of human intelligence processes by machines. Usually, complicated patterns are analyzed using algorithms and large amounts of data. Applications for artificial intelligence (AI) are numerous and include everything from facial recognition software and autonomous cars to virtual assistants like Siri and predictive text on smartphones and medical diagnostic systems. Its extensive use in a variety of industries demonstrates its capacity to completely transform the correct and efficient completion of operations.
Artificial intelligence uses methods like machine learning and deep learning to simulate human cognitive processes. By teaching algorithms to identify patterns in data, machine learning makes it possible for computers to make judgments without the need for explicit programming. Deep learning is a branch of machine learning that processes data in layers using neural networks that are modelled after the structure of the human brain. AI can carry out activities with progressively higher degrees of accuracy and complexity by utilizing various technologies, including speech recognition, image categorization, natural language processing, and more. Artificial Intelligence (AI) aims to mimic human intelligence by continuously enhancing its capacity to learn from data and adjust to novel situations.
3. Exploring Machine Learning:
The goal of machine learning, a branch of artificial intelligence, is to create statistical models and algorithms that let computers perform better at a given task without having to be specifically programmed for it. Because it enables systems to learn from data, identify patterns, make judgments, and get better over time, machine learning is essential to artificial intelligence.
Machine learning algorithms come in a variety of forms, each with its own learning style. Supervised learning is a popular kind in which computers are taught on labeled data sets and then make judgments or predictions based on patterns identified during training. Unsupervised learning relies on finding patterns or links in the data itself to learn from datasets lacking labeled responses. An algorithm can be taught to make judgments through trial and error and to learn from results by receiving positive or negative feedback thanks to reinforcement learning. For complex applications, learning strategies that incorporate elements of supervised and unsupervised approaches are available in the form of semi-supervised and self-supervised approaches. Every kind of machine learning algorithm has a distinct function and a special ability to address a range of problems in a variety of industries.
4. Delving Into Deep Learning:
Deep learning is a form of machine learning that uses artificial neural networks to model and interpret complicated patterns in data. Machines can learn representations of data with numerous degrees of abstraction by training algorithms to analyze input via multiple layers of neural network designs.
Deep learning is essential for advanced artificial intelligence applications like picture and audio recognition, natural language processing, autonomous cars, and more because of its capacity to manage enormous volumes of unstructured data effectively. In many different sectors, deep learning approaches have greatly increased the accuracy and performance of AI systems.
Deep learning is based on neural networks, which process information by simulating the composition and operations of the human brain. These networks are made up of interconnected nodes arranged in layers, each of which processes the input data using a different mathematical operation. From straightforward feedforward neural networks to more complicated structures like convolutional neural networks (CNNs) for image processing or recurrent neural networks (RNNs) for sequential data, deep learning designs can range widely in complexity.
Deep learning architectures employ these neural network structures to automatically extract features from raw data, enabling machines to learn complicated patterns without explicit programming. Deep learning models can make accurate decisions and predictions thanks to the hierarchical representation they have learned, which opens the door for revolutionary developments in AI technology. 🤭
5. Key Differences Between AI, ML, and DL:
Artificial intelligence (AI), machine learning (ML), and deep learning (DL) are all closely related terms in the field of technology, yet they each have distinct scopes and functionalities.
The broadest term for machines that can carry out human-like functions, like language comprehension, object recognition, and decision-making, is artificial intelligence (AI). Within AI, machine learning (ML) is a subfield that focuses on how computers may learn from data without explicit programming. It makes it possible for systems to gain experience and enhance their performance over time. On the other hand, deep learning is a branch of machine learning that makes use of multi-layered neural networks to find intricate patterns in massive volumes of data.
The main distinction between them is the breadth of their approaches: while ML concentrates on creating algorithms that automatically improve performance over time, DL specializes in teaching neural networks to identify patterns in data. AI seeks to build machines that can reason and solve problems similarly to humans. Knowing these differences can make it easier to understand how AI, ML, and DL are used in various technical contexts and their respective constraints.
6. Real-World Applications:
With their cutting-edge applications, artificial intelligence (AI), machine learning (ML), and deep learning (DL) are transforming a number of industries. AI is utilized in healthcare for predictive analytics, which foretells diagnostics and patient admissions. Personalized medicine is aided by machine learning algorithms that analyze patient data and provide customized treatment approaches. Medical scans can help discover diseases like cancer early since DL enables image recognition algorithms. 😷
AI is essential to algorithmic trading in finance, as ML models use market trends to make instantaneous investment decisions. Machine learning techniques are employed by bank fraud detection systems to detect suspicious activity and avert financial crimes. Deep learning is employed for risk assessment by evaluating massive datasets to predict market volatility and optimize investing strategies.
Artificial intelligence (AI) technologies, such computer vision, are crucial to autonomous cars' ability to recognize and detect objects on roads. Self-driving cars can learn from prior experiences and make better decisions when negotiating traffic thanks to machine learning algorithms. In order to improve perceptual abilities and make autonomous vehicles safer and more effective on the roads, deep learning models evaluate complicated sensor data.
These illustrations highlight how the enhanced capabilities of AI, ML, and DL are revolutionizing a variety of industries, including healthcare, banking, and transportation, by increasing productivity, accuracy, and decision-making processes.
7. Advantages and Limitations:
There are several benefits to implementing deep learning (DL), machine learning (ML), and artificial intelligence (AI) technology across a variety of businesses. AI makes it possible to automate, expedite, and accurately complete a variety of jobs, from customer service to data analysis. With machine learning, computers can gradually increase their performance without explicit programming. Large datasets for challenging pattern recognition tasks, such as speech or picture identification, are well-suited for deep learning (DL), a subset of machine learning. These innovations have the power to completely transform commercial operations and offer answers to pressing social issues.
Nevertheless, there are difficulties in putting AI, ML, and DL into practice. The need for large amounts of high-quality data in order to properly train these algorithms is one of their main drawbacks. Acquiring pertinent datasets can pose challenges and incur significant costs, particularly in specialist fields. There are several obstacles to overcome before AI applications may be used ethically, such as bias in algorithmic decision-making or privacy issues with data security. Because of their complexity, these technologies frequently call for specialist knowledge, which not all businesses wishing to implement them will be able to purchase or simply obtain.
Despite these difficulties, when applied wisely, the advantages of using AI, ML, and DL surpass the drawbacks. In the current digital environment, businesses must properly use new technologies while giving careful thought to their unique needs in order to realize their full potential for innovation and success.
8. Future Trends:
Anticipating forward, a number of fascinating developments in the fields of artificial intelligence (AI), machine learning (ML), and deep learning (DL) are reshaping the technological environment. Focusing on Explainable AI is one new trend that seeks to increase transparency and accountability in AI systems by providing a human-understandable explanation for their judgments. Automation powered by AI is poised to transform a number of sectors, including healthcare and finance, by optimizing workflows and boosting productivity.
Federated Learning, a decentralized method that permits several parties to work together on model training without exchanging sensitive data, is anticipated to gain traction in the field of machine learning. Promising uses for this technology can be found in privacy-sensitive industries including finance and healthcare. It is becoming more common for edge devices to incorporate machine learning (ML) techniques, allowing IoT devices to make decisions in real time without relying on cloud infrastructure.
With the investigation of new architectures like Transformers and GANs (Generative Adversarial Networks), deep learning keeps progressing. These models have demonstrated exceptional performance in tasks related to natural language processing and the creation of realistic synthetic data, hence presenting new opportunities in sectors such as fraud detection and content synthesis. Researchers continue to place a high priority on resolving issues with model interpretability and energy economy as DL models get bigger and more intricate.
Future developments in AI, ML, and DL suggest that these technologies will become more accessible to both individuals and enterprises, hence democratizing their use. It is anticipated that multidisciplinary research projects and collaborative research efforts would spur additional innovation, resulting in revolutionary applications across industries and clearing the path for a more intelligent future driven by data-driven choices.😍
9. Ethical Considerations:
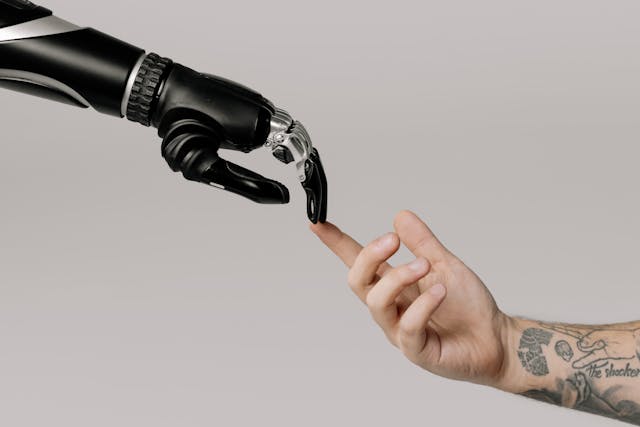
When talking about the application of deep learning (DL), machine learning (ML), and artificial intelligence (AI) technologies, ethical issues are crucial. The increasing integration of these technologies into our daily lives raises important ethical considerations that must be addressed.
Biases in AI systems as a result of skewed training data sets are a significant source of worry. These systems have the potential to reinforce and even worsen inequality if they are trained on data that reflects societal biases. Preventing prejudice against specific categories of individuals requires making sure AI systems are impartial, open, and devoid of bias.
The possible effects of AI on employment are another ethical factor to take into account. There are worries about broad job displacement across numerous industries as AI and automation technologies improve. A seamless transition to an AI-driven future depends on finding ways to counteract these consequences, such as by retraining programs or opening up new job opportunities in developing industries.
With the increasing use of AI systems that gather and process vast volumes of data, privacy issues surface. Upholding data protection protocols to secure individuals' personal information is essential to preserving user confidence and trust in enterprises using AI, ML, and DL technologies.
To ensure that AI, ML, and DL technologies serve humanity as a whole, navigating the ethical issues around them demands a careful strategy centered on transparency, justice, privacy protection, and addressing potential social repercussions.
10. Impact on Society:
In a variety of fields, deep learning (DL), machine learning (ML), and artificial intelligence (AI) are transforming civilization. Artificial Intelligence (AI) in education is personalizing learning through adaptive learning platforms that adjust to the demands of each individual student. To improve student results and institutional effectiveness, machine learning is augmenting educational analytics. Deep learning (DL) is enabling intelligent tutoring systems for advanced learning support by processing large amounts of data.
Artificial Intelligence is automating tasks in the employment sector, raising concerns about job displacement but also opening up new opportunities in specialized domains related to AI. In order to efficiently screen candidates, recruitment processes use machine learning algorithms. Predictive analytics for personnel management and operational optimization in sectors like manufacturing and logistics are made possible by DL.📱
Concerns about privacy are growing as AI technologies proliferate. Large volumes of user data are frequently collected by AI-powered apps, which raises concerns about data security and privacy. Strong security measures are required for ML algorithms that handle personal data breaches. Transparent procedures are necessary for DL systems to guarantee the appropriate handling of sensitive data.
Artificial intelligence (AI), machine learning (ML), and deep learning (DL) are revolutionizing various industries and transforming society. However, they also present new issues that call for ethical deliberations and regulatory structures to safeguard people and communities against the possible hazards linked to these formidable technologies.
11. Innovations in AI Research:
Thanks to developments in machine learning and deep learning, artificial intelligence (AI) has advanced significantly in recent years. Numerous industries have seen a change thanks to advances in AI research, which have also opened up fascinating new opportunities. One such innovation is the creation of GPT-3, a language model that can produce text that is human-like on a never-before-seen scale. AlphaFold, an AI system with remarkable prediction accuracy for protein folding, is another noteworthy accomplishment. It has great promise for use in biological research and medication development.
Machine Learning technique developments have made a substantial contribution to the advancement of AI. Reinforcement learning, for example, has made it possible for algorithms to learn complicated behaviors without the need for explicit programming, opening the door to applications like AI systems that can play games and drive themselves. Models trained on one task can now be effectively recycled for new tasks using transfer learning, which saves time and computational resources. The emergence of self-supervised learning techniques has created opportunities for efficiently training models on large volumes of unlabeled data.
In order to push the limits of AI capabilities, Deep Learning—a subset of Machine Learning that focuses on neural networks with several layers—has proved crucial. While recurrent neural networks (RNNs) have proven essential in sequential data analysis applications like speech recognition and natural language processing, convolutional neural networks (CNNs) have achieved notable breakthroughs in picture recognition tasks. The incorporation of attention processes in neural networks has enhanced model interpretability and performance on numerous tasks.
The interplay among Deep Learning, Machine Learning, and Artificial Intelligence is pushing innovation in a variety of domains. These discoveries highlight the revolutionary potential of AI while also emphasizing the necessity of continued research and cooperation. We can expect even more ground-breaking findings as we explore the possibilities of these technologies, which will influence the direction of AI-driven services and applications in the future.
12. Conclusion:
After a summary of the material presented, it is clear that there are differences between machine learning (ML), deep learning, and artificial intelligence (AI). A subset of artificial intelligence (AI), machine learning (ML) is the ability of computers to learn from data without explicit programming. AI is the broad concept of machines accomplishing tasks that traditionally require human intelligence. A kind of machine learning called "Deep Learning" uses multi-layered neural networks that can learn from vast amounts of data.
Deep Learning is a method inside Machine Learning, which is a subset of Artificial Intelligence, which is why both technologies are related. Collectively, they are essential in determining the future of technology. The flexibility of AI drives many industries, the data-driven methodology of ML improves decision-making, and the sophisticated neural networks of Deep Learning provide answers to challenging issues.
Businesses and industries hoping to make the most of these technologies' potential in a constantly changing technological landscape will need to grasp the subtleties of these technologies and how interrelated they are. It's not just about picking one over the other; it's about realizing how AI, ML, and Deep Learning work best together to spur innovation and move us closer to a more intelligent future.🫠