1. Introduction
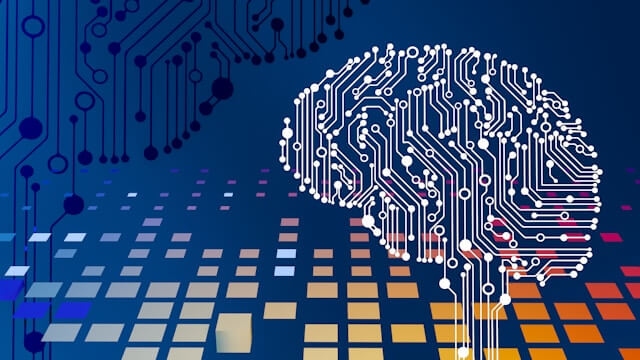
Introduction: Data quality is the foundation of any successful data-driven strategy. Without accurate, reliable data, businesses risk making flawed decisions that could have serious repercussions. Whether you're a small startup or a large corporation, ensuring high data quality should be a top priority to drive business growth and innovation. However, maintaining pristine data can be challenging due to various factors that can degrade its quality over time.
Common Issues with Data Quality:
Your data's quality may be impacted by a number of typical problems. System restrictions or human mistake during data entry are common causes of inaccurate or incomplete data. Another common problem that might cause biased analysis and subpar decision-making is duplicate entries. The issue is exacerbated by inconsistent formatting and out-of-date data, which makes it challenging to draw insightful conclusions from the data. To tackle these obstacles, a methodical strategy and committed work are needed to set up strong data governance procedures and optimize procedures for gathering, storing, and validating data.
2. Understanding Your Data Quality Problem
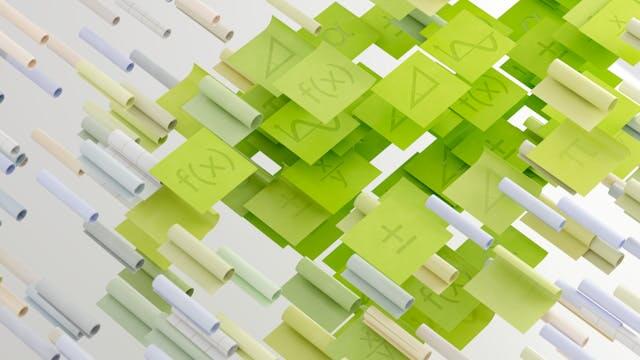
To solve your data quality issue, you must have a thorough understanding of it. Finding the sources of faulty data within your company is a crucial component. This entails examining the locations and methods of data collection, storing, and processing in order to identify any erroneous or inconsistent data-prone places. Human error in data entry, out-of-date systems producing disparities, and problems with database integration are common causes of inaccurate data.
After determining the sources of faulty data, the next stage is to examine how decision-making processes are affected by the faulty data. Incomplete or inaccurate data might result in erroneous conclusions, poor insights, and poor strategic choices. You can see the extent of the issue and identify areas that need to be improved by knowing how these problems affect every level of the decision-making hierarchy in your company. This research is essential for emphasizing how urgent it is to address issues with data integrity and for showing how seriously they affect business performance.
3. Establishing Data Quality Goals
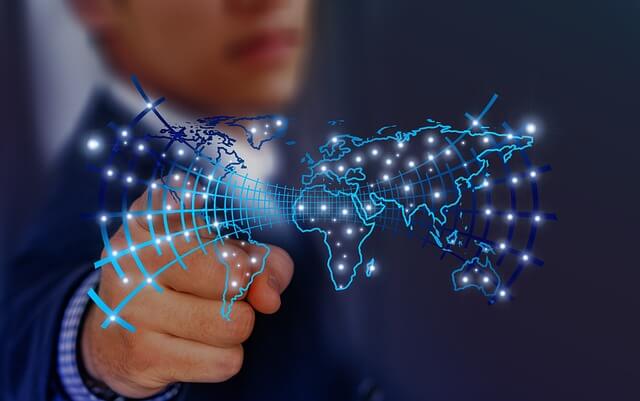
One of the most important steps in fixing data quality problems inside a company is setting targets. A clear road map for evaluating and tracking advancement is provided by defining important metrics for data quality improvement. These metrics might include the data's legitimacy, timeliness, accuracy, consistency, and completeness. Every metric ought to be customized to the unique requirements and difficulties the firm faces.
It's crucial to set reasonable goals for every indicator to make sure gains are quantifiable and attainable. Objectives ought to be time-bound, relevant, quantifiable, achievable, and specific (SMART). For instance, within six months, a company might aim to increase data accuracy from 90% to 95%. Organizations can monitor their progress and hold themselves responsible for attaining improvements in data quality by establishing these specific goals.
For the company as a whole to support and align with the data quality goals, it is crucial to include essential stakeholders in the process. Through active communication with IT staff, data scientists, business users, and other pertinent stakeholders, businesses can acquire important insights into how different operations and processes are affected by low-quality data. This cooperative method aids in the establishment of significant objectives that target the particular problems faced by various departments or teams.
Any effective data quality program must define the critical measures for improving data quality and set attainable goals. Organizations can significantly raise their data quality standards by implementing a methodical goal-setting process and incorporating important stakeholders.
4. Implementing Data Quality Tools
To effectively handle data quality challenges, it is imperative to implement data quality tools. Your data can be made more accurate and consistent by using data cleansing tools and procedures. These tools assist you in finding and fixing mistakes, discrepancies, and duplicates in your datasets. You may make sure your data is accurate and up to date by using data cleansing technologies, which can facilitate improved decision-making.🗯
Improving data quality also requires automating data validation procedures. In the validation process, automation reduces the possibility of human error while simultaneously saving time. You can manually verify your data integrity on a regular basis by establishing automatic validation methods. This proactive strategy guarantees that your dataset is accurate throughout its lifecycle and aids in the early identification of inconsistencies.
By integrating data quality technologies into your workflow, you may create strong procedures for upholding high standards for data quality. You may increase operational effectiveness, simplify your data management procedures, and eventually produce superior business results by using these tools and practices correctly. Investing in data quality solutions increases stakeholder confidence in the integrity of your organization's data assets and improves the dependability of your information.
5. Creating a Data Quality Framework
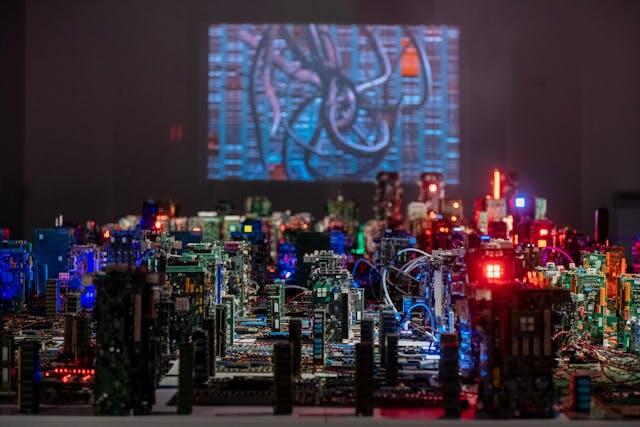
For any company to effectively address data quality challenges, developing a strong foundation for data quality is crucial. Data quality can be continuously maintained and improved over time with the aid of a methodical methodology. Establish specific goals and data quality-related key performance indicators (KPIs) at the outset. This will help you track your progress and give you a strategy for improving the quality of your data.
Setting up roles and duties inside the company is essential to the success of your framework for data quality. Assign particular responsibilities for tracking, evaluating, and enhancing data quality to people or groups. Ascertain precisely who is in charge of data governance, data cleansing, data profiling, and continuous measurement of data quality indicators. You may guarantee accountability and ownership of data quality across the whole organization by designating roles and responsibilities.
In order to successfully deploy a data quality strategy, communication is essential. Make certain that everyone involved in data quality activities is aware of their respective roles and responsibilities. Keeping everyone in sync with the organization's objectives for enhancing data quality can be achieved through regular meetings, training sessions, and updates. In order to foster a culture of shared accountability for data quality and completeness, encourage cooperation across departments.🫥
Review and update your data quality strategy frequently to keep up with evolving business requirements and technological developments. Your organization's strategy for preserving high-quality data should also change as it does. Seek input from relevant parties on a regular basis regarding the success of your data quality initiatives, and remain flexible to adapt as circumstances demand. You can make sure that your data quality strategy is still applicable and useful in the long run by continuing to be flexible and adaptable.
Taking into account everything mentioned above, we can say that building a strong framework for data quality entails defining defined roles and duties inside the company and constructing a methodical approach to preserving high-quality data. You may tackle current data quality concerns and avoid new ones by clearly defining roles, creating clear objectives, encouraging collaboration, and updating your framework often. Make it a priority to invest in the data quality framework of your company, as it is the cornerstone of both well-informed decision-making and overall business success.
6. Data Governance Best Practices
Data quality must be maintained by data governance. Organizations may guarantee compliance with legislation like GDPR by adhering to best practices. Protecting sensitive data requires putting strong rules for data protection and access into place.😸
Companies must set explicit policies about who can access data, how it should be used, and what security precautions must be taken to prevent breaches in order to maintain data integrity. It is recommended to implement routine audits and monitoring systems to monitor compliance and pinpoint opportunities for enhancement.
Businesses can reduce risks related to poor data quality, protect sensitive information, and foster trust with stakeholders and customers by incorporating data governance best practices into their operations. Setting data governance as a top priority guarantees organizational preparedness in an increasingly regulated digital environment while also improving overall data quality.
7. Continuous Monitoring and Improvement
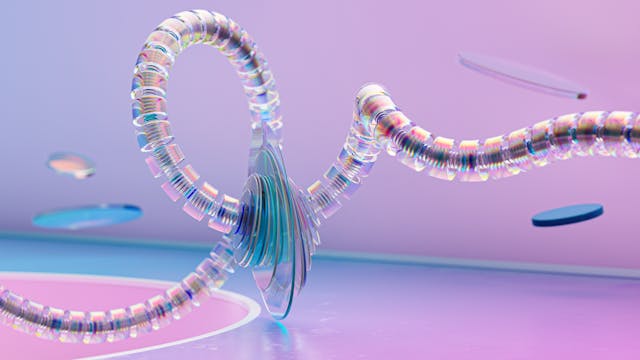
An organization's ability to address problems with data quality requires constant monitoring and development. Establishing routine data quality audits and inspections guarantees that any disparities or inconsistencies are quickly found and fixed. Through the use of uniform monitoring procedures, companies can uphold a high level of data integrity.
Enhancing processes iteratively in response to audit findings is essential for long-term improvement of data quality. Organizations can identify reoccurring problems or patterns influencing data quality by analyzing audit results. With time, more accurate and dependable data will result from their focused enhancements of current processes or systems made possible by this knowledge.
To sum up what I've written so far, you should always be proactive in resolving data quality issues by integrating continuous monitoring and improvement activities into your data management approach. Organizations may improve their data's overall correctness and dependability, which will help them make better decisions and achieve better business results. This can be achieved by implementing regular audits and using the insights from these evaluations to improve internal procedures.
8. Training and Awareness Programs
Putting training and awareness initiatives into place is essential to effectively addressing problems with data quality. Ensuring that employees comprehend the significance of data quality and their part in preserving it is mostly dependent on education. Organizations may enable their personnel to input accurate and dependable data by offering thorough training on data entry best practices.
Emphasizing the effects of low-quality data on decision-making procedures, company operations, and overall organizational performance should be the main goal of training sessions. The importance of data completeness, correctness, consistency, and timeliness cannot be overstated. Employees can understand the practical repercussions of data inaccuracies and inconsistencies through case studies and real-life examples. 💽
Providing helpful advice on recommended practices for data input can greatly enhance the caliber of information entered into systems. Standardized formats, validation guidelines, mistake detection methods, and the need of frequent updates should all be included in training. Organizations may reduce errors at the point of entry and improve overall data quality across all systems and processes by providing staff with these resources and training.
Putting money into education and awareness campaigns shows a dedication to excellence in data management and ongoing progress. Organizations may reduce the risks associated with low-quality data and provide a foundation for improved decision-making by cultivating a culture that emphasizes accuracy and dependability in data processing.
9. Case Studies of Successful Data Quality Fixes
Real-world case studies provide essential insights into how businesses effectively address data quality concerns in the field of data management. A prominent instance is the case of Company X, a global enterprise battling errors in their client list. Through the application of rigorous validation procedures and the acquisition of data cleansing instruments, Company X successfully addressed errors and improved the general quality of their data. Better client relations and more focused marketing initiatives resulted from this.
The story of Startup Y, a tech company having problems with duplicate information in their inventory database, is another motivational case study. Startup Y achieved considerable cost reductions, increased productivity, and streamlined operations with the implementation of deduplication software and frequent audits. This success story emphasizes how crucial proactive data quality measures are to stopping problems before they get worse.
A financial institution's response to reporting errors resulting from mismatched data formats is demonstrated in Case Study Z. Report accuracy and compliance levels improved significantly for the organization after they standardized their data input processes and staff training initiatives. This is an example of how thorough data governance procedures can reduce the dangers brought on by inadequate data quality.
The aforementioned case studies highlight the significant impact that giving data quality first priority may have on an organization's operations. Businesses can obtain important insights into best practices for successfully addressing data quality concerns and improving decision-making processes across many departments by taking note of these successful case studies.
10. Measuring Success and ROI
Understanding the value your data quality initiatives offer to your business requires measuring their success and return on investment (ROI). An assessment of the effects of enhanced data quality on company results include examining key performance indicators (KPIs) that are impacted by data quality, including revenue growth, operational efficiency, and customer happiness.
Take into account the expenses associated with starting and continuing these activities as well as the results they produce when calculating the return on investment for your data quality programs. These advantages can include decreased mistakes, better decision-making based on trustworthy data, more productivity as a result of streamlined procedures, and increased client trust, which raises acquisition and retention rates.
Prior to putting data quality measures into action, define precise metrics in order to monitor progress effectively. Monitor how KPIs evolve over time and contrast them with benchmarks established before data quality was improved. Conducting regular assessments can help you gauge the efficacy of your efforts and make adjustments as needed to continue creating excellent outcomes for your organization.