1. Introduction
The issues of creating and managing data in huge enterprises are addressed by data mesh, a decentralized approach to data architecture. Instead of centralizing data, it promotes data organization around business domains, which makes it easier for teams to own and manage their data. In today's data-driven world, creating a data mesh is essential for handling complex and diverse data sources with scalability, flexibility, and agility. Organizations may boost team communication, enhance data quality, and make more informed decisions based on trustworthy insights by implementing a data mesh framework.
2. Understanding Data Mesh
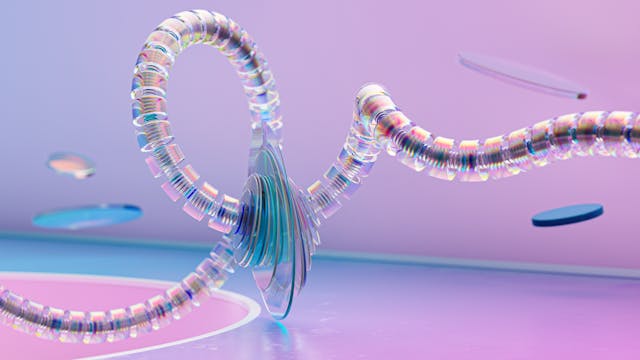
**Understanding Data Mesh**
**Defining what a data mesh is:**
A decentralized method of exchanging and managing data inside an organization is called a data mesh. It comprises dividing conventionally large, centralized data systems into more manageable, distributed domains. These domains, also known as meshes, consist of self-serve data products that contain the processing, storage, and logic required for a particular business function. With the use of common interfaces that other domains can use to access and use this data, this design permits each domain to control and own its own data.
**Explaining its principles and key components:**
Domain-oriented decentralization, product thinking, automation over integration, and federated governance are the fundamental tenets of a data mesh. In domain-oriented decentralization, each domain or team is given the autonomy to manage its own data assets. Data is treated as a product in product thinking, with distinct ownership and value propositions linked to particular business requirements. Building automated procedures for obtaining and utilizing data rather than depending on human involvement is the focus of automation over integration.
Domain-specific data products, self-serve platforms for generating and administering these products, a governance framework guaranteeing adherence to organizational policies and standards across domains, and a federated metadata layer facilitating the identification and comprehension of available data assets are essential elements of a data mesh architecture. Organizations can establish a culture of collaboration and ownership across teams managing varied datasets while improving their data management processes' agility, scalability, and innovation by implementing a data mesh strategy.
3. Challenges with Traditional Data Architectures
Organizations have always managed their data using traditional centralized data architectures. In most of these architectures, all data is processed, stored, and analyzed in a single data lake or warehouse. This method presents a number of difficulties even though it offers advantages in terms of governance and consistency. Scalability is one of the main issues; when data volumes increase dramatically, centralized systems find it difficult to handle the growing demands made on them. Performance problems, bottlenecks, and delays in getting important insights can result from this.
A data mesh design, on the other hand, distributes these duties among several domains or business units within an organization in an effort to decentralize data ownership and processing. Compared to conventional designs, this move from a centralized to a decentralized one has various benefits. Organizations can better extend their data infrastructure and increase flexibility and agility in responding to changing business needs by delegating ownership and processing of data.
Traditional methods sometimes suffer from segregated data structures that make it difficult for employees to collaborate and share insights throughout the company. When faced with a variety of data sources and formats, centralized systems frequently find it difficult to maintain consistency and quality. Users who rely on this data for decision-making may become suspicious as a result, making it difficult to maintain data accuracy and integrity.
It can be difficult for traditional architectures to adapt to new technology or changing business needs since they are inflexible and stiff. This inability to change can stifle creativity and make it more difficult for the company to successfully utilize new trends like machine learning, real-time analytics, and IoT integrations.đź“—
Although traditional centralized data architectures have proven to be effective for enterprises in the past, the demands of the quickly changing digital ecosystem of today are progressively outpacing them. The transition to a data mesh architecture is a viable substitute that tackles numerous obstacles encountered by conventional methods, allowing enterprises to optimize their data assets more efficiently.
4. Benefits of Implementing a Data Mesh
There are a number of important advantages to implementing a data mesh framework, which can completely change how businesses handle and use their data. Increased scalability is a significant benefit. A data mesh enables horizontal data infrastructure scalability to effectively manage increasing volumes of information by decentralizing data ownership and governance.
Increased organizational agility is facilitated by a data mesh. Decision-making procedures become more effective and flexible in response to shifting business requirements when domain-oriented teams assume control of their data products. Teams can swiftly adjust to new requirements or insights because to this flexibility, which prevents them from being hampered by centralized bottlenecks.
Increased data accessibility is among a data mesh's most important advantages. Through the dismantling of data silos and the creation of transparent data product interfaces, disparate teams may quickly identify and obtain the pertinent datasets required for analysis or application development. Teams throughout the company may now more effectively utilize insights thanks to the democratization of data, which promotes informed decision-making and innovation at all levels.
5. Key Components of a Data Mesh
Key Components of a Data Mesh
Key elements that are necessary for the structure and operation of a data mesh must be assembled successfully. Domain-oriented decentralized data ownership is one of a data mesh's fundamental ideas. This indicates that the domains or business units in charge of the data inside an organization own and manage the data. This type of decentralized ownership makes each domain accountable for the governance and integrity of its data, resulting in more accurate and dependable insights.
Federated governance is another essential part of a data mesh. The term "federated governance" describes the decentralized decision-making mechanisms that direct the management of data across many domains inside an organization. It guarantees uniformity in norms, regulations, and procedures while granting each domain the freedom to decide what is best for their own requirements. Cohesion and alignment within a data mesh framework are contingent upon striking a balance between decentralized authority and centralized supervision.
A data mesh can effectively scale and adapt to the increasing complexity of contemporary data ecosystems by including these ideas into its design. Domain-oriented decentralized data ownership promotes local accountability and knowledge, which strengthens an organization's culture of making decisions based on data. Federated governance, on the other hand, offers the framework and direction required to guarantee smooth collaboration between many domains while maintaining uniform standards and legal obligations.
To sum up, the key to creating a successful data mesh is to comprehend and put into practice essential elements like federated governance and domain-oriented decentralized data ownership. In addition to enabling specific domains to assume responsibility for their data, these components set explicit standards for cooperation and coordination throughout the enterprise. In today's quickly changing digital landscape, firms may fully utilize their data assets by adopting these concepts.
6. Building Blocks of Data Mesh Architecture
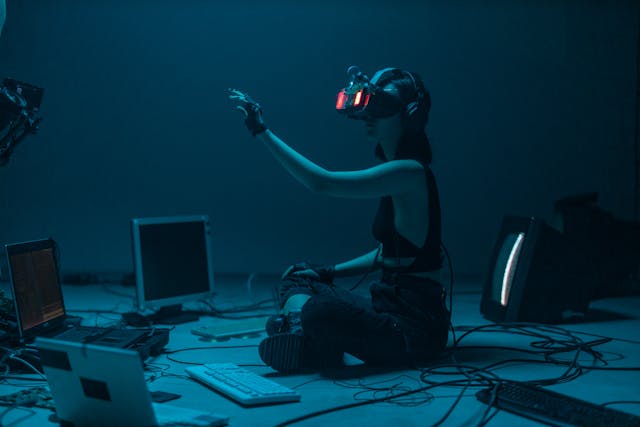
By decentralizing data, a Data Mesh redefines the conventional centralized method in the field of data architecture. The fundamental elements of a data mesh are those that enable enterprises to efficiently handle their data at scale. In order to increase usability and accessibility, domain-driven design—which emphasizes organizing data around business domains—plays a crucial role. Self-serve platforms provide teams with autonomy, making it easier to access data and encouraging departmental collaboration. By considering data as a product and incorporating software engineering concepts into data management, adopting product thinking changes the conversation toward better results. Organizations can utilize Data Mesh architecture to fully utilize their data assets by comprehending these architectural pieces.
7. Implementing a Data Mesh Strategy
To guarantee a seamless transition, implementing a data mesh approach involves careful preparation and implementation. Determining new ownership and governance structures is one of the most important things to think about when implementing a data mesh architecture. This entails creating a culture of data accountability, decentralizing data ownership within specific domain teams, and setting precise rules for data sharing and cooperation.
Constructing self-serve data infrastructure is another essential step. Organizations may empower their domain teams to effectively exploit data for their unique needs by putting in place tools that make it simple for them to find, access, and exchange data. This entails establishing reliable data pipelines, tools for categorization, and systems for quality control in order to promote autonomy while preserving centralized management.
For implementation to be successful, addressing cultural change is just as important as technical factors. Establishing a culture of continuous learning and improvement, fostering information sharing and teamwork, and cultivating trust within teams are the main priorities for organizations. Building cross-functional relationships and embracing transparency in decision-making are crucial elements of a healthy data mesh ecosystem.
Defining clear metrics for measuring success, overcoming siloed mindsets within teams, ensuring data quality and consistency across domains, scalability issues as the organization grows, and resistance to change from traditional centralized approaches are some of the challenges associated with implementing a data mesh strategy. Strong team communication channels, sufficient training and assistance for upskilling staff, frequent evaluations of the system's efficacy, and strategy revisions based on feedback and insights gained along the way are all critical to overcoming these obstacles.
The best practices for a successful implementation include testing the new strategy in small-scale pilot projects before progressively expanding it throughout the entire organization. Getting important stakeholders involved early in the process can assist ensure alignment and buy-in toward shared objectives. Organizations can make educated decisions for continuous development and proactive course correction by routinely comparing performance measurements and KPIs to specified objectives. đź–Ť
A change in perspective is necessary to adopt a data mesh strategy that preserves control over security and compliance standards while democratizing data access. Organizations can position themselves to succeed in developing a contemporary, flexible data architecture that satisfies the changing demands of the complicated business environment of today by painstakingly adhering to these guidelines and tackling obstacles head-on while keeping best practices in mind.
8. Real-world Examples of Data Mesh Deployments
It is clear from looking at actual data mesh deployments that a variety of businesses in a range of sectors have effectively adopted this cutting-edge method of data management. A big online retailer that used a data mesh to democratize and consolidate access to its enormous amount of consumer data is the subject of one such case study. The organization was able to improve its customer insights and provide more individualized shopping experiences by dismantling data silos and giving cross-functional teams self-serve data capabilities.
Another striking example comes from a top financial institution that used a data mesh to improve the efficiency of its data operations and facilitate better decision-making. By introducing domain-oriented data products and decentralized governance frameworks, the company was able to enhance data quality, encourage cooperation amongst various business divisions, and eventually attain increased flexibility in reacting to market fluctuations.
The significance of encouraging an organizational culture of data adoption and collaboration is one of the most important lessons to be drawn from these implementations. Technological developments alone won't be enough to ensure the success of data mesh deployments; a mentality change that values decentralized ownership of data assets and encourages data literacy at all organizational levels is also necessary. Stakeholder engagement and effective communication are essential for securing support and promoting the data mesh framework's successful deployment.
When implementing a data mesh, it is imperative that enterprises give scalability and flexibility first priority when designing their architecture. Businesses may guarantee long-term sustainability and adaptation in a constantly changing technology world by developing modular, reusable data components that are easily expanded across numerous business units or use cases.
These actual cases demonstrate the revolutionary effect that a well-implemented data mesh strategy can have on an organization's capacity to realize the full value of its data assets. By adopting a decentralized data management strategy and cultivating a collaborative and innovative culture, enterprises can surmount the conventional obstacles linked to centralized, monolithic systems and also provide a path towards sustained expansion and prosperity in the digital era.
9. Tools and Technologies for Data Mesh
Using the appropriate tools and technologies is essential to the development and upkeep of a data mesh ecosystem. A data mesh approach's requirement for distributed architecture and metadata management can be supported by a wide range of platforms and technologies.
A data mesh's potential benefits can only be fully realized with the help of platforms that make distributed architectural management possible. These platforms offer infrastructure for data sharing, processing, and storage as well as aid in orchestrating data pipelines across multiple domains. Examples include cloud-based platforms like AWS, Google Cloud, or Azure for scalable infrastructure, Apache Kafka for real-time data streaming, and Apache Hadoop for distributed storage and processing.
Tools for managing metadata are vital parts of a data mesh because they assist in organizing and controlling metadata from various sources throughout the network. These technologies assist in identifying data lineage, quality, ownership, and usage across decentralized domains. To guarantee data integrity and compliance, well-known metadata management technologies like Apache Atlas, Collibra, or Alation offer features for metadata discovery, classification, and visualization.
Organizations implementing a data mesh may also make use of technologies like Kubernetes for container orchestration, Apache Spark for big data processing, or Presto for federated querying across multiple datasets, in addition to platforms for managing distributed architecture and metadata management tools. Businesses may efficiently construct and maintain a robust data mesh architecture that improves their analytics capabilities while encouraging agility and autonomy across teams by choosing the appropriate combination of technologies suited to their particular needs.
10. Measuring Success: KPIs for Data Mesh Adoption
Measuring success is essential when putting a Data Mesh approach into practice. Key Performance Indicators (KPIs) are essential instruments for monitoring this strategy's efficacy. It is important to keep a tight eye on metrics that show more data democratization, shorter time to insights, better operational efficiency, and better data quality.
1. **Data Quality Metrics**: Metrics like data completeness, accuracy, consistency, and reliability may be tracked to see how well the Data Mesh approach is improving data quality throughout the company.
2. **Time to Insights**: The effectiveness of the Data Mesh implementation may be determined by tracking how quickly data is converted into insightful knowledge. A shorter time to insights implies that data is moving through the system more easily.
3. **Operational Efficiency**: Metrics such as infrastructure expenses, resource usage, and overall operational performance can be tracked to assess if the Data Mesh approach is assisting the company in attaining operational efficiencies.
4. **Data Democratization**: Metrics pertaining to the extent to which various teams and departments access and use data can be used to determine the degree of democratization that can be attained with a Data Mesh method.
Organizations can assess the success of their Data Mesh endeavor and take well-informed decisions to improve their data architecture for better outcomes by establishing KPIs that concentrate on these areas.
11. Future Trends in Data Management: The Role of Data Mesh
The idea of a Data Mesh has the potential to completely change how businesses manage their data assets in the future. The decentralization of data ownership and governance resulting from this novel method is expected to influence the future generation of data architecture. Forecasts suggest that Data Mesh will keep gaining popularity as businesses hunt for more effective and scalable solutions to handle the increasing amounts of data they produce.
The growing usage of data mesh across a range of industries is one important trend that is anticipated in its evolution. As organizations see the benefits of democratizing data access and processing capabilities, they are likely to welcome this paradigm shift. By enabling individual teams within a company to take control of their data domains, democratization is expected to accelerate innovation and increase agility.
Businesses' approaches to maintaining and utilizing their data assets are likely to change as Data Mesh adoption increases. More decentralized methods that adhere to the tenets of a data mesh may eventually replace conventional centralized ones. This change could lead to improved communication between various departments and teams within a business, dismantling organizational silos and promoting a culture of data-driven decision-making.
The way businesses organize and use their data assets is changing dramatically, as evidenced by the continuous growth of Data Mesh. Organizations may position themselves for more agility, scalability, and creativity in a world that is becoming more and more data-driven by adopting this new strategy. Based on the aforementioned information, we can draw the conclusion that comprehending and putting into practice a Data Mesh strategy may be essential to realizing the full potential of data inside a company and influencing the direction of data management procedures in the future.
12. Conclusion
In summary, a data mesh is a paradigm shift in data architecture that prioritizes self-serve capabilities, decentralization, and domain-centricity. When constructing a data mesh, organizations ought to prioritize dismantling data silos, enabling domain teams, encouraging data reuse and discovery, and utilizing scalable infrastructure. The data ecosystem of a business may become more innovative, autonomous, and flexible as a result of this strategy.
In the long run, adopting a data mesh can have a significant impact on how businesses handle and use their data in the future. Businesses may better handle the complexity of today's data challenges by using this creative strategy, which helps them maintain their competitiveness in a world that is becoming more and more data-driven. Adopting a data mesh structure can put organizations in a position to fully utilize their data assets and produce significant commercial results as the volume and variety of data keep increasing.