1. Introduction:
Big Data has emerged as a key success factor in today's business environment. It alludes to the enormous amount of organized and unstructured data that constantly floods a company. This data is gathered from several sources, including sensors, social media, and commercial transactions. Big Data is significant because it has the ability to provide insightful information that might completely change the way businesses run.
Businesses can make well-informed decisions based on verifiable information rather than conjecture or gut feeling when they successfully leverage big data. Organizations can learn more about customer preferences, market trends, operational efficiencies, and much more by examining this abundance of data. By quickly responding to changing circumstances, businesses may stay competitive in a market that is changing quickly by utilizing big data.
2. Understanding the Basics of Big Data:
Big Data is the term used to describe data sets that are too big and complicated for conventional data processing software to handle efficiently. Big Data is characterized by three key elements: diversity, velocity, and volume.πΆβπ«οΈ
Volume is the term used to describe the massive amount of data that is produced every second by a variety of sources, including corporate applications, social media, and sensors. The pace at which this data is generated and must be processed in real-time or very close to it is referred to as its velocity. Variety refers to the various forms of data that are being gathered, including unstructured, semi-structured, and structured data, which makes it difficult to organize and analyze.
To succeed with Big Data initiatives, organizations must understand and effectively manage these key elements to derive valuable insights and drive informed decisions.
3. Setting Clear Objectives:
Establishing specific goals is a vital cornerstone to successfully leveraging big data. Organizations that lack clear goals run the risk of being lost in a sea of data with no direction or purpose. Having well-defined goals gives you a road map for using Big Data to generate business results and make wise decisions. They assist in concentrating efforts, assets, and analysis on the most important areas that coincide with the strategic priorities of the firm.
Incorporating key stakeholders from throughout the organization is essential when defining clear objectives for Big Data initiatives to guarantee alignment with overarching business goals. SMART stands for precise, measurable, achievable, relevant, and time-bound goals, which make it possible to monitor development and gauge success along the way. Companies can more accurately assess the results of their Big Data initiatives and make necessary strategy adjustments to stay on track by setting these SMART goals.
Organizations can better focus their efforts in data collecting and analysis by having clear objectives. Establishing clear objectives helps organizations avoid becoming overwhelmed by the massive amounts of unstructured data; instead, they can concentrate on gathering the appropriate data sets that are directly related to accomplishing those goals. In addition to saving time and money, this focused strategy raises the possibility of producing insights that may be put into practice and produce significant outcomes.
In summary, establishing well-defined goals is essential to realizing the complete potential of big data in an enterprise. Companies can manage the intricacies of Big Data with purpose and accuracy by adopting a SMART framework for goal-setting, involving key stakeholders in the process, and defining precise goals that are in line with corporate strategies. In today's increasingly data-centric environment, having clear objectives paves the way for leveraging data-driven insights that spur innovation, enhance decision-making procedures, and accelerate corporate success.
4. Data Quality and Governance:
To successfully handle Big Data, two fundamental pillars must be established: data quality and governance. The conclusions obtained by Big Data analysis may be faulty or undermined if data quality is not properly attended to. Data governance guarantees the security, consistency, accuracy, and legal compliance of the data. It establishes guidelines and procedures for the upkeep, use, and management of data during its whole lifecycle. Organizations may rely on the accuracy of their analytical results and make well-informed decisions by upholding strict governance procedures and high standards for data quality. Establishing a strong foundation for efficiently exploiting Big Data to drive corporate growth and innovation involves implementing robust data quality controls in conjunction with effective governance.
5. Advanced Analytics Techniques:
Using advanced analytics methods is essential to realizing big data's full potential. Through the application of techniques such as machine learning and predictive modeling, enterprises are able to get meaningful insights from large datasets. using the help of machine learning algorithms, which can automatically learn from experience and make improvements without the need for explicit programming, firms can find patterns and trends that would not be visible using more conventional analytics techniques.
Another important tool in advanced analytics is predictive modeling, which enables businesses to project future results using historical data. Businesses are able to make proactive decisions by using statistical algorithms and machine learning approaches to predict trends, behaviors, and events. In the end, these sophisticated analytics techniques provide more precise forecasts and a deeper comprehension of data relationships, which leads to well-informed choices and strategic planning.
Adopting sophisticated analytics methods gives firms hoping to capitalize on big data a whole new range of opportunities. The insights gained by machine learning and predictive modeling have the potential to completely transform company processes, whether they are related to finding new market opportunities, streamlining operations, or improving consumer experiences. Businesses may keep ahead of the competition and take advantage of big data's enormous potential by investing in these advanced analytical tools and methods.
In summary, utilizing cutting-edge analytics methods like machine learning and predictive modeling is crucial to succeeding with big data. Organizations can gain a competitive edge, spur innovation, and improve decision-making processes by using these potent technologies to extract actionable insights from complex datasets. By utilizing a strategic combination of technology, expertise, and planning, companies can fully utilize big data and set themselves up for future expansion and prosperity.
6. Leveraging Technology Solutions:
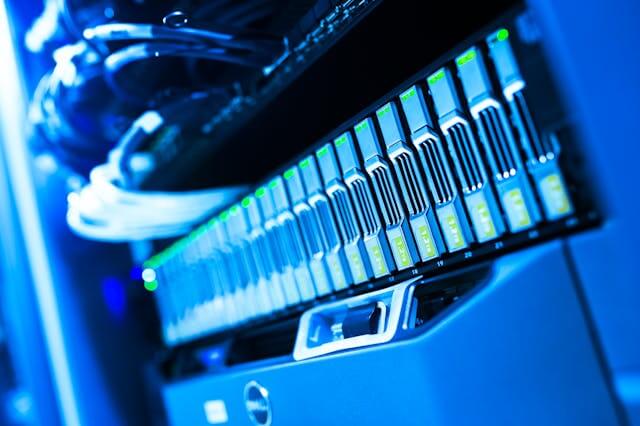
Utilizing technological solutions is essential to managing large amounts of data successfully. The ability to process massive datasets effectively has made tools like Hadoop and Spark indispensable. Hadoop makes scalable and dependable data processing and storage possible with its distributed file system and MapReduce programming methodology. Conversely, Spark's fault tolerance and in-memory processing make it quick and simple to use, which makes it perfect for interactive queries and iterative algorithms.
Due to their ability to provide parallel processing across computer clusters, these technologies are essential in helping to overcome the difficulties presented by large data quantities. They offer the framework required to efficiently glean insightful information from massive volumes of data. Organizations may analyze data more quickly, make wiser decisions, and obtain a competitive edge in the data-driven world of today by judiciously utilizing these technologies.
By incorporating these technologies into current systems, data pipelines may be made more efficient, data quality can be raised, and overall operational effectiveness can be increased. Adopting cutting-edge tools allows for real-time analytics and machine learning applications in addition to speeding up data processing. Using cutting-edge technologies like Hadoop and Spark is essential for organizations to stay ahead in the big data analytics space as they struggle with ever-growing information.
7. Building a Skilled Team:
Putting together a knowledgeable team is essential to any big data project's success. To solve the difficulties of big data, a varied team of engineers, analysts, and data scientists brings together a breadth of experience. Large datasets can yield valuable insights, which data scientists then mine for insights, analysts then use to make decisions, and engineers use to put strong systems for data collecting and analysis into place.
A talented staff guarantees that the project is tackled from several angles, resulting in more thorough solutions. When team members with different skill sets work together, they can generate ideas, find innovative solutions to problems, and promote innovation in the efficient management of large amounts of data. By enhancing one other's strengths and shortcomings, team members can maximize the performance of the group as a whole and bring out the best in each member.
A knowledgeable staff is more suited to handle the constantly changing big data tools and technology landscape. The team's options for upskilling and ongoing learning enable them to stay current with industry trends and innovations. This proactive approach guarantees that the project stays competitive and relevant in a constantly evolving sector, while also improving their capabilities.
A key component of big data success is assembling a talented team of engineers, analysts, and data scientists. Their combined knowledge, cooperation, attitude of constant learning, and flexibility are crucial for overcoming obstacles, spurring creativity, and accomplishing good results in challenging big data initiatives.
8. Ensuring Scalability and Security:
Achieving success with big data projects requires ensuring scalability and security. In order to sustain performance as datasets grow, scaling challenges must be adequately addressed. It is crucial to put in place scalable technology and infrastructure that can manage growing data volumes. Stressing the significance of data security procedures is essential to preventing breaches and unauthorized access to sensitive data.
Organizations should invest in technologies that can process massive amounts of data efficiently, including distributed computing frameworks, to ensure scalability. Effective management of expanding datasets can also be achieved by employing scalable storage systems and cloud services. Through the use of automated scaling mechanisms, which modify resources in response to demand, businesses can maximize productivity without sacrificing effectiveness.
When working with large data, data security needs to be the first focus. Encryption, access limits, and monitoring systems are a few strong security measures that may be put in place to protect data from potential dangers. Mitigating risks associated with processing and storing huge volumes of data requires regular updates to security policies and vulnerability audits.
Scalability and security should be given top priority in big data initiatives so that businesses may guarantee dependable performance while safeguarding important data assets. Long-term success will result from incorporating these essential components into the entire big data strategy, which will also help firms effectively use data-driven insights for innovation and decision-making.
9. Real-world Case Studies:
An essential component of comprehending the real-world application of big data success is real-world case studies. Through exhibiting instances of enterprises that have proficiently utilized big data, we can extract significant knowledge and guidance for our own undertakings. These case studies offer tangible proof of big data's influence on companies in a range of industries in addition to showcasing the technology's potential.
Netflix's use of big data analytics to transform the entertainment sector is one such excellent case study. Using advanced algorithms that examine viewers' tastes and viewing patterns, Netflix offers millions of customers across the globe customized recommendations. This customized strategy has helped Netflix become the industry leader in digital streaming by greatly increasing user satisfaction and retention.
Amazon's use of big data to improve customer experience is another excellent example. Amazon optimizes its product recommendations, pricing tactics, and inventory management by evaluating massive volumes of data on user behavior, preferences, and purchase habits. The remarkable success of Amazon as an e-commerce behemoth can be attributed in part to its deliberate exploitation of big data.
The transformational power of big data may be fascinatingly observed in the healthcare industry. Big data analytics has been effectively used by businesses like IBM Watson Health to enhance medical research, illness diagnosis, and patient care. IBM Watson Health helps medical professionals make better judgments and create individualized treatment plans by evaluating vast amounts of research data and patient information.
These actual case studies highlight the enormous potential that big data has when used wisely by businesses. They demonstrate how, in today's data-driven economy, insights from large datasets may spur innovation, improve operational effectiveness, and ultimately result in competitive advantages. Businesses can gain important insights into leveraging big data to drive their own growth and success in an increasingly connected world by examining these success stories.
10. Forecasting Future Trends:
Businesses hoping to keep ahead in this ever changing environment must predict future trends in big data. Organizations can gain a competitive advantage by anticipating changes and taking proactive measures to adapt by keeping a watch on developing technologies and processes. To fully exploit Big Data, it is essential to comprehend how new advances in artificial intelligence, machine learning, cloud computing, and data analytics will affect data collection, analysis, and use.
Companies with the ability to accurately predict emerging patterns in big data are better able to streamline processes, find fresh growth prospects, and make well-informed decisions. By keeping up with the latest developments in real-time data processing, prescriptive analytics, and predictive analytics, businesses may adjust their tactics to suit shifting consumer tastes and market demands. By adopting a progressive stance towards Big Data, companies may utilize data-driven insights to foster creativity and long-term prosperity.
Accurately anticipating events and finding significant patterns from the continually growing volume and variety of data requires a mastery of predictive modeling techniques. Innovative technologies such as blockchain, natural language processing (NLP), and Internet of Things (IoT) sensors can help firms better comprehend market dynamics, consumer behavior, and industry trends, and improve their predictive skills. Businesses can stay flexible and strong in the face of uncertainty by adjusting to these new developments.
As I mentioned earlier, a key to succeeding in the data-driven economy of today is predicting future trends in big data. Through innovation adoption, utilization of sophisticated analytics instruments, and proactive industry advancement, enterprises may fully unleash the possibilities of Big Data to stimulate expansion, enhance decision-making, and secure a competitive edge. In an environment where information genuinely is power, those that predict change proactively and adjust quickly will prosper.
11. Conclusion:
Based on the aforementioned information, we can infer that a strategic approach incorporating multiple essential components is necessary to achieve success with Big Data projects. For the data being examined to be accurate and reliable, data quality and governance are crucial. Large volumes of data may be efficiently processed and analyzed by enterprises thanks to scalable infrastructure and sophisticated analytics tools. Effective Big Data leveraging requires a culture that values data-driven decision-making and encourages cross-team cooperation.
For Big Data projects to be successful, it is essential to have individuals with the necessary skills to work with sophisticated data sets and technologies. Setting data security and compliance as a top priority aids in protecting sensitive data in an increasingly digital environment. Organizations will be able to maintain their ability to use Big Data for strategic benefit if they embrace innovation and remain flexible in response to changing technological landscapes.
Companies may optimize the benefits of their Big Data efforts and achieve significant commercial outcomes by concentrating on these essential components: talent, organizational culture, security, innovation, and agility; data quality; infrastructure; analytics capabilities; and organizational culture. Recall that leveraging big data to foster innovation within your company and make educated decisions is just as important to success as having a large volume of data.
12. Call to Action:
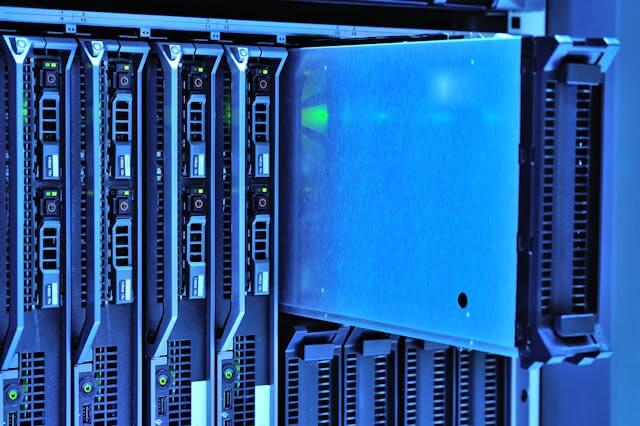
We may infer from all of the above that giving these eight essential components top priority is what will determine if big data initiatives succeed. Organizations may fully utilize their data assets by concentrating on data quality, scalability, security, talent, analytics tools, collaboration, alignment with business objectives, and continual development. Now is the moment to act. Put these tactics into practice right now to improve your data-driven decision-making and maintain your competitive edge in this rapidly changing digital environment. Don't delay; doing so will determine your organization's future success.