1. Introduction:
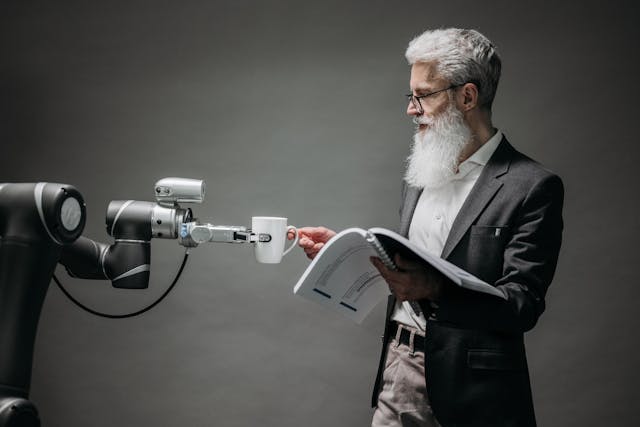
Big data analytics, as it pertains to the healthcare industry, is the act of analyzing vast and intricate datasets that have been collected from several sources. The goal of this study is to find important trends, correlations, and insights that can help with decision-making and enhance patient outcomes. Big data analytics is important to the healthcare industry because it has the ability to transform the way doctors provide care, improve operational effectiveness, forecast epidemics, customize patient care, and ultimately save lives. Instead of only treating patients as they become ill, healthcare professionals can adopt a more proactive and preventive approach to medicine by utilizing the power of data.
2. Challenge 1: Data Security and Privacy Issues:
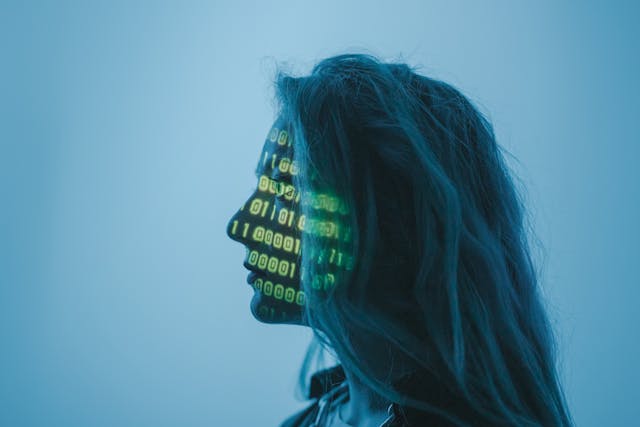
Concerns about privacy and data security are crucial to big data analytics in the healthcare sector. Due to the large quantity of personal data that is gathered, including financial information, medical records, and more, protecting sensitive healthcare data is essential. Any concession in this domain may have dire consequences for people as well as the healthcare system as a whole.
Significant hazards arise from data breaches, which can result in identity theft, insurance fraud, patient confidentiality violations, and harm to healthcare providers' reputations. The disclosure of personal identifiers or medical histories may lead to both monetary loss and negative effects on the health of the patients. It might reduce public confidence in healthcare organizations and obstruct initiatives to use data-driven insights to enhance patient outcomes.
Strong security mechanisms including encryption, access limits, frequent audits, and employee training must be put in place to meet these obstacles and safeguard sensitive data throughout its lifecycle. HIPAA compliance is necessary but insufficient; to counteract the growing risks in today's healthcare environment, a complete strategy incorporating technical improvements and strict rules is required.✉️
3. Challenge 2: Data Integration from Various Sources:
Challenge 2: Data Integration from Various Sources
Currently, one of the biggest obstacles in big data analytics for healthcare is integrating data from many sources. Healthcare institutions frequently handle massive amounts of data coming from wearables, medical devices, electronic health records, and other sources. The format, organization, and degree of detail found in each source can vary, making it difficult to combine all of this data in an efficient manner.📦
When it comes to precise analysis in the healthcare industry, the significance of smooth data integration cannot be emphasized. A comprehensive understanding of patient health, treatment outcomes, and operational efficiency in healthcare settings is made possible by the coherent combination of data. Ineffective integration of different sources can lead to the loss or skewing of important information, which can affect the way decisions are made and how patients turn out.
To find patterns, trends, and correlations that can lead to improvements in treatment protocols, personalized medicine, and preventative care initiatives, healthcare practitioners rely on integrated data. Big data analytics has the potential to completely alter healthcare delivery, but this promise will remain unfulfilled in the absence of smooth integration across many sources. The key to obtaining useful insights that might enhance patient care and expedite industry processes is figuring out how to integrate these disparate data streams.
4. Challenge 3: Scalability of Infrastructure:
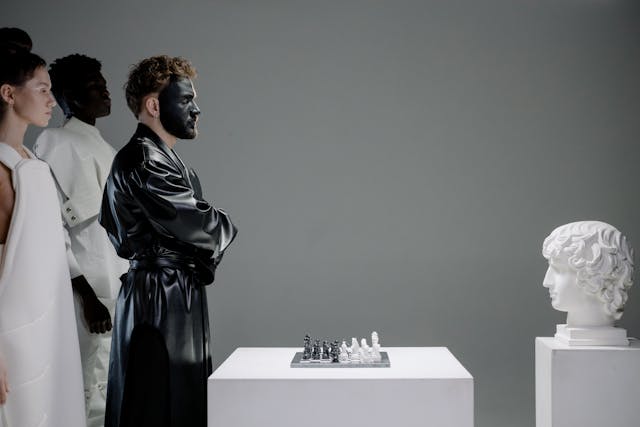
Scalability of infrastructure is the third challenge in big data analytics for healthcare. Every day, the healthcare sector handles enormous amounts of data, thus having a scalable infrastructure is essential. Managing the ever-increasing volume of data effectively without compromising speed or accuracy is a major challenge.
Healthcare companies can use a number of ways to efficiently scale their infrastructure in order to meet this difficulty. Scalable processing and storage capacity can be obtained on-demand through the use of cloud computing services like AWS or Azure, enabling flexibility as data quantities rise. Large datasets can be processed more quickly by using technologies like distributed computing frameworks like Hadoop or Spark, which can assist split workloads over several computers.
Scalability and speed can be improved by using data partitioning techniques to help distribute data over several nodes for parallel processing. Reducing superfluous data amount and increasing overall handling efficiency of big datasets can be achieved by putting into practice a data management strategy that prioritizes data quality and cleaning.
Taking into account everything mentioned above, we can say that guaranteeing infrastructure scalability is essential to overcoming the difficulties presented by big data analytics in the healthcare sector today. Healthcare companies may efficiently grow their infrastructure to meet the needs of handling huge amounts of healthcare data while retaining speed and accuracy in analytics operations by utilizing cloud services, distributed computing frameworks, and smart data management methods.
5. Challenge 4: Data Quality and Accuracy:
The fourth challenge in big data analytics for the healthcare sector is correctness and quality of data. Analytical results can be severely hampered by poor data quality, which can result in incorrect conclusions and choices. Incomplete or inaccurate data can skew forecasts, jeopardize patient treatment, and eventually have an impact on the whole healthcare system. Strict data governance regulations that uphold high standards of data quality throughout the data's lifecycle are one way to address this difficulty.
Organizations can create automated procedures for data cleansing and validation to guarantee data accuracy and dependability. Vital practices include updating and monitoring databases on a regular basis, thoroughly checking them for flaws or inconsistencies, and setting up feedback mechanisms for concerns about data quality. The detection of erroneous data points can be enhanced by utilizing cutting-edge technology such as machine learning algorithms for anomaly detection, which can lead to an overall improvement in data integrity.
It is imperative to cultivate a culture of accountability among stakeholders with respect to data entry and upkeep. Employees can develop a sense of ownership by receiving thorough training on the value of correct data collection and utilization. Ensuring consistency across departments and enhancing overall data accuracy in healthcare analytics can be achieved by working with cross-functional teams to establish standardized methods for data collection, storage, and sharing.
6. Challenge 5: Regulatory Compliance and Governance:
Today's fifth challenge in big data analytics for the healthcare sector is governance and regulatory compliance. The sensitive nature of healthcare data presents complex issues for healthcare companies. Compliance is essential to any data analytics project in this industry since regulatory organizations impose stringent requirements to protect patient privacy and data security.
The Health Insurance Portability and Accountability Act is one of the most well-known laws (HIPAA). HIPAA regulates the use, storage, and exchange of sensitive patient data and establishes requirements for its protection. Healthcare professionals who violate HIPAA may face harsh penalties, such as fines and legal repercussions. Therefore, a major obstacle for healthcare firms using big data analytics is making sure that HIPAA requirements are followed.
Healthcare data management is further complicated by additional requirements, such as the General Data Protection Regulation (GDPR) in European nations. These rules mandate that businesses secure express consent before processing patient data and maintain openness regarding the collection and use of patient data. One of the biggest challenges facing the business today is finding a balance between using big data analytics to improve healthcare outcomes and following strict regulatory requirements.
7. Challenge 6: Skill Shortage and Training Needs:
Lack of skills and training requirements is a major issue in the field of big data analytics in healthcare. Professionals need to have specific skills like data science, programming, statistical analysis, and domain knowledge in healthcare to effectively use big data. Finding someone with this particular combination of skills can be difficult, though.
A significant obstacle is the dearth of experts with a thorough understanding of data analytics and healthcare operations. Because of its interdisciplinary nature, this subject requires a unique set of abilities that are difficult to come by. The skill sets needed are always changing due to the quick progress of technology, which makes it challenging for firms to stay up to date with the newest technologies and trends in the market.
Even in cases where qualified personnel are available, standardized training programs designed to address the particular requirements of big data analytics in healthcare are frequently lacking. It could be necessary for current employees to retrain or upskill in order to meet the needs of this changing environment. Businesses have difficulty offering opportunities for ongoing education and motivating staff members to advance their data analytics skills.
A comprehensive approach that prioritizes cooperation between academic institutions, industry stakeholders, and healthcare organizations is needed to address these issues. Through focused training initiatives and collaborative partnerships that encourage knowledge exchange and skill enhancement, the healthcare sector can cultivate a strong workforce capable of utilizing big data to enhance patient outcomes and streamline operations.
8. Challenge 7: Real-time Data Analysis:
**Challenge 7: Real-time Data Analysis**
In the medical field, real-time data analysis is essential to improving patient care. Real-time analytics may save lives by allowing medical personnel to rapidly access and evaluate data, which can result in more prompt actions and a decrease in errors. Continuous data monitoring of patients enables early identification of significant changes in their health status, enabling medical professionals to act quickly and administer the necessary therapies.
For healthcare companies, however, streaming data analysis and processing pose serious obstacles. The sheer amount of data created in real time is a significant problem that makes it challenging to manage and quickly extract insightful information. Another challenge is guaranteeing the consistency and correctness of real-time data since delays or irregularities could affect the way decisions are made.
Preserving the confidentiality and security of private, sensitive real-time health information is critical, but it can be challenging to comply with stringent standards like HIPAA while processing data quickly. In order to overcome these obstacles and fully realize the potential of real-time data analysis in revolutionizing healthcare delivery paradigms, it will be necessary to put advanced technologies like stream processing systems, machine learning algorithms for real-time predictive analytics, and strong cybersecurity measures in place.
9. Challenge 8: Interoperability Among Systems:
Challenge No. 8: Cooperation One major obstacle to the healthcare industry's use of big data analytics is among systems. One challenge is the variety of systems employed in healthcare facilities, many of which are ineffective in communicating with one another. The absence of interoperability results in data silos, impeding both effective data analysis and patient treatment that is comprehensive.
It is imperative to develop uniform data formats and protocols across various systems in order to tackle this difficulty. Interoperability can be increased by creating application programming interfaces (APIs) that make it simple for systems to share and integrate data. Encouraging healthcare providers to make investments in interoperability-supporting technologies will also facilitate smooth platform-to-platform connectivity.
Smooth data transmission across various healthcare systems can be facilitated by encouraging the implementation of Health Level Seven International (HL7) standards for electronic health records. Overcoming this barrier and maximizing the advantages of big data analytics in enhancing patient outcomes and operational efficiencies within the sector would require highlighting the significance of interoperability in healthcare IT infrastructure design and compliance with laws.
10. Challenge 9: Ethical Use of Patient Data:
The ethical use of patient data is the ninth challenge in big data analytics for healthcare. In order to complete this challenge, you must analyze the moral ramifications of using confidential medical data for analysis. Establishing precise policies and procedures that guarantee patient data handling privacy, confidentiality, and permission is essential to resolving this problem. It is imperative for healthcare institutions to give patients' consent explicit permission for each specific usage and to be transparent with them about how their data will be used.
Anonymization techniques, which remove personal identifiers from datasets while preserving the ability to do meaningful analysis, are one option to respect ethical standards while taking use of big data insights. Healthcare providers can safeguard patient privacy and confidentiality while gaining important insights from huge datasets by de-identifying data. Patient data can be protected from breaches and unwanted access by putting strict security measures in place like encryption.
To ensure the ethical use of patient data, healthcare companies must cultivate a culture of responsibility and responsible data stewardship. This entails educating employees on appropriate data handling procedures, carrying out frequent audits to check for adherence to privacy laws, and setting up channels for reporting any possible breaches or improper use of patient data. Healthcare organizations can successfully manage the challenges of using patient data responsibly while fostering innovation and enhancing treatment outcomes by integrating ethics and compliance into the fundamentals of big data analytics techniques.
11. Challenge 10: Cost Management for Big Data Projects:
Challenge 10: Cost Management for Big Data Projects
Financial difficulties may arise when implementing big data analytics in the healthcare sector for a number of reasons, including expensive infrastructure, data storage, and specialized human resource needs. Organizations can take into consideration a number of affordable solutions to help relieve these difficulties. Prioritizing the adoption of cloud-based and open-source software, which frequently have reduced upfront costs and scalability based on consumption, is one strategy. By spending money on training current employees in data analytics, you can lessen your dependency on outside resources and save money on employing expert workers.
During big data projects, scope creep and needless spending can be avoided by setting clear project goals and a well-defined strategy. By effectively managing massive volumes of data, scalable systems like Hadoop and Spark can help reduce processing expenses. Collaborating on joint projects with academic institutions or research groups is another important component. This approach can give cost-sharing options in addition to access to specialist expertise.
In summary, while cost management is still a major obstacle for big data projects in the healthcare industry, organizations can effectively manage their financial resources while utilizing data analytics to improve patient outcomes and operational efficiencies by adopting a strategic approach that combines cost-effective technologies, internal skill development, and external partnerships.🙂
12. Conclusion:
Taking into account everything mentioned above, we can say that the healthcare sector has a lot of obstacles when it comes to putting big data analytics into practice today. These obstacles include concerns about data security and privacy, integrating different data sources, ensuring data quality, complying with regulations, and dealing with resistance from stakeholders. To fully utilize big data in healthcare and improve patient outcomes, several challenges must be overcome.
Healthcare companies can unleash the revolutionary power of big data analytics by tackling these issues head-on with strong data governance frameworks, cutting-edge analytics technology, staff training programs, and cultivating a culture of data-driven decision-making. Large-scale healthcare data can provide insights that can be used to improve patient care through more individualized therapies, better diagnosis, faster processes, and better resource allocation.📉
To effectively negotiate the difficult terrain of big data analytics, stakeholders in the healthcare sector must work closely with technological specialists and regulatory organizations. By doing this, the sector can overcome these obstacles and open the door to a time when data-driven innovation transforms the delivery of healthcare and improves patient outcomes everywhere.