1. **Introduction**
Organizing the enormous volumes of data produced in the field of genomics research is one of the biggest problems facing scientists as it continues to advance quickly. Significant challenges arise in the areas of storage, processing, and analysis due to the sheer volume of genomic data, which includes sequences, variants, and annotations. Researchers run the danger of becoming overwhelmed by the amount of information available to them if they don't have good strategies in place.
For genomes research to advance, these issues must be resolved. In addition to guaranteeing data accessibility and integrity, effective big data management opens the door to fresh discoveries and insights. By addressing these challenges, researchers may fully utilize genomic data to propel advancements in disease knowledge, customized medicine, and other vital biotechnological fields. In order to advance our understanding of genetics and its applications in healthcare and other fields, it is imperative that genomics large data management be made more efficient.
2. **Understanding Genomics Big Data**
The term "genomics big data" describes the enormous volumes of genetic data produced by different methods like transcriptomics, epigenetics analysis, and DNA sequencing. This information includes all of an organism's genes, profiles of gene expression, and regulatory components. With its ability to shed light on genetic variances, disease causes, treatment responses, and evolutionary links, genomics big data has a vast application.
Genome sequencing data, which entails figuring out the nucleotide sequence of an organism's entire genome, is one source of big data in genomics. Gene expression patterns in various tissues or environments are captured by transcriptomic data. Changes to the DNA sequence that can affect gene activity without altering the underlying genetic coding are revealed by epigenetic data. Additional sources include metabolomic data, which describes the tiny compounds found in cells, and proteomic data, which identifies the proteins made by an organism.
These many genomics big data sources are essential for developing research in customized medicine, agriculture, evolutionary biology, and environmental science, among other areas. Scientists may decipher intricate biological processes, find illness biomarkers, create tailored treatments, improve crop breeding initiatives, and comprehend how organisms adapt to their surroundings by examining this abundance of data. Big data in genomics has the power to transform our understanding of life at the molecular level and spur technological advancements that will improve both environmental and human health.
3. **Challenges in Genomics Big Data Management**
In genomics, handling large amounts of data comes with many difficulties. The enormous amount of data produced by the processes of genome sequencing, processing, and interpretation makes scalability a significant problem. The constant flood of data may be too much for traditional storage methods to handle, resulting in bottlenecks and sluggish processing speeds.
Big data management in genomics has substantial challenges related to data storage and retrieval. Due to the sheer bulk of genomic datasets, efficient and reasonably priced high-capacity storage systems are needed. For rapid analysis and research results, it is essential to guarantee fast and dependable access to this enormous volume of data.
Given the sensitive nature of genetic data, security and privacy issues are major considerations. Because genetic information is so private, there are moral questions about consent, data ownership, and possible misuse. It is critical to protect this data against security lapses, unwanted access, and accidental disclosure in order to preserve public confidence in genetic research and its uses in healthcare. Strict access controls and cutting-edge encryption techniques are necessary to safeguard genetic data at every stage of its existence.
4. **Technological Solutions for Genomics Big Data Management**
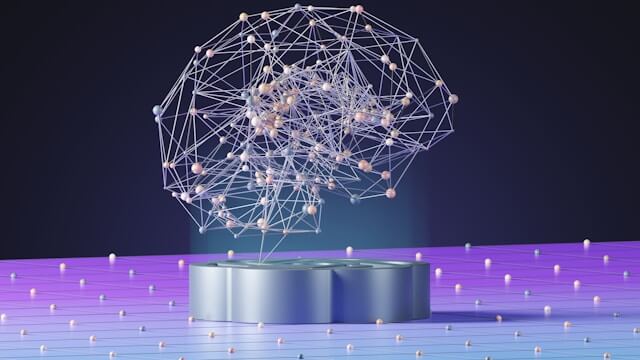
Technological solutions are essential for tackling huge data management difficulties in genomics. In genomics research, cloud computing proves to be a potent instrument for scalable storage solutions. Researchers may effectively store, access, and analyze large volumes of genomic data without worrying about hardware limits by utilizing cloud infrastructure.
Apart from cloud computing, data compression methods provide a another efficient approach to handling huge genomics datasets. With the use of these methods, scientists can preserve the accessibility and integrity of genomic data while lowering the amount of storage needed. Researchers can increase overall data management efficiency, optimize storage resources, and improve data transport speeds by compressing genetic datasets.
Through the investigation of technical solutions like cloud computing and data compression techniques, the genomics community can surmount the obstacles presented by big data and open up novel avenues for research and finding advancement in this swiftly changing field.
5. **Data Integration and Interoperability in Genomics**
Data integration and interoperability are essential components for efficient research and analysis in the field of genomics. By integrating data from several sources, including gene expression profiles, DNA sequences, and clinical data, integrative datasets enable researchers to obtain a more thorough understanding of genetic information. By using an integrated method, scientists can find fresh insights that might not be seen by examining each dataset alone.
In order to ensure interoperability across the various datasets and technologies used in genomics research, standardization efforts are essential. Researchers can more readily access and share genomic data across many platforms and labs by developing common standards for data formats, metadata, and annotations. This helps to improve the reproducibility and reliability of genomics investigations in addition to encouraging collaboration within the scientific community.
In order to progress research efforts, make more accurate analyses possible, and ultimately lead to discoveries that can expand our understanding of genetic pathways and customized medicine, it is imperative that genomics data integration and interoperability be fostered.
6. **Machine Learning Applications in Genomics Big Data Management**
The management of large amounts of data in genomics presents a number of issues that machine learning must solve. Machine learning can help with the fast and accurate analysis of large amounts of genetic data by using complex algorithms and statistical models. It supports the process of pattern recognition, prediction, genetic variation classification, and significant insight extraction from large, complicated datasets by researchers. In genomics, machine learning algorithms that facilitate effective data analysis and interpretation include regression, classification, clustering, and deep learning.
By speeding up research, eliminating human error, and automating monotonous processes, artificial intelligence (AI) has a lot of potential to improve genomic data management procedures. Scientists may combine various datasets, speed up data processing, and find relationships that might not be visible using more conventional methods with AI-powered technologies. Large amounts of genomic data can be used to train AI systems to forecast illness risks, identify possible therapeutic targets, and tailor treatment plans based on the unique genetic profiles of each patient. Precision medicine programs are encouraged to be innovative and resource usage made more efficient by the incorporation of AI technology with genomics.
From all of the above, we can conclude that
The big data management in genomics has great potential to transform the way we study genetic data using machine learning applications. Sophisticated algorithms can be used to find hidden patterns in large datasets, which can lead to important breakthroughs in disease research, customized therapy, and biological knowledge. Researchers can expedite scientific discoveries and enhance global healthcare outcomes by leveraging AI-driven tools and methodologies for genetic data processing and interpretation.
7. **Best Practices for Effective Genomic Data Management**
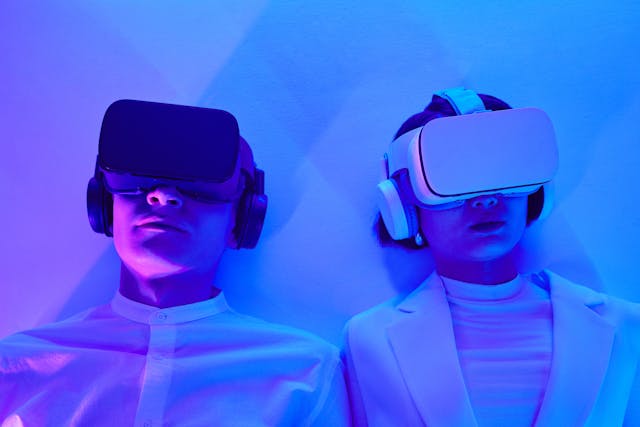
The establishment of strong data governance policies is essential to the efficient administration of genetic data. Organizations can guarantee adherence to regulations and preserve data integrity by establishing unambiguous policies on data collection, storage, and sharing. This comprises describing who can access the data, how to use it, and the procedures for maintaining data quality.
It is imperative to apply tactics that prioritize preserving data accuracy, completeness, and consistency in order to guarantee high-quality genetic data. Frequent validation procedures and audits can aid in the early detection of mistakes or irregularities. By making it easier for others to repeat experiments or studies, using standardized formats for data entry and storage can further improve reproducibility.
Setting up documentation procedures that describe data processing stages, analytical techniques, and any alterations made to the original dataset is crucial in addition to these tactics. This improves transparency in study findings in addition to helping to ensure reproducibility. Organizations may maximize the value gained from their datasets while navigating the complexity of big data by adhering to best practices in genomic data management.
8. **Case Studies: Successful Implementation of Genomic Data Management Strategies**
Effective genomic data management has the potential to be demonstrated by the numerous organizations that have successfully used creative ways for handling massive data in genomics. To manage the enormous volumes of genetic data they produce, the Broad Institute in Cambridge, Massachusetts, for example, has established strong data management procedures and has been at the forefront of genomics research. Advanced storage options, data processing pipelines, and platforms for researcher sharing and cooperation are all part of their methodology.
In a similar vein, businesses such as DNAnexus have developed robust systems that facilitate the safe and effective handling of genetic information. Large genomic datasets may be easily stored, analyzed, and shared with ease thanks to DNAnexus' cloud-based technologies. Many academic institutions and healthcare organizations have been able to expedite their genomes research while maintaining compliance with privacy requirements thanks to this streamlined strategy.
Large-scale genomic data management is now possible thanks to the establishment of top-notch infrastructures by organizations like the European Bioinformatics Institute (EBI). Databases, analysis tools, and training materials are just a few of the services provided by EBI to help researchers all around the world efficiently navigate and utilize big data in genomics. With its state-of-the-art resources and bioinformatics knowledge, EBI has emerged as a major force behind the advancement of genetic research worldwide.😎
These case studies emphasize how crucial it is to put customized methods into place in order to effectively handle big data in genomics. Organizations may fully utilize genomic data to propel ground-breaking discoveries and advances in precision medicine by implementing best practices in data management.
9. **Future Trends in Genomic Data Management**
Technology will be crucial in changing the way that genomics data management is done in the future. The development of sophisticated AI and machine learning algorithms to quickly and reliably handle and evaluate enormous volumes of genetic data is one important trend. With the use of these tools, researchers will be able to find intricate patterns in genomic datasets that were previously difficult or time-consuming to find. Solutions for cloud computing, which provide scalable processing and storage for managing genomics data without requiring a sizable on-site infrastructure, will proliferate.
Using blockchain technology to improve data integrity and security in genomics data management is another new trend. Blockchain technology can offer a decentralized, impenetrable method for genetic data storage that promotes stakeholder trust and privacy. Novel techniques like federated learning might become popular as long as genomics research produces ever-larger datasets. Federated learning preserves privacy by enabling several parties to work together on the analysis of dispersed datasets without exchanging sensitive information, all the while utilizing the pooled knowledge to enhance research.
The creation of standards and protocols that are compatible with one another will be essential to the smooth sharing of data and cooperation between different databases and research institutes. These standards will enable more effective global sharing and integration of genomic data by promoting data harmonization and compatibility. Genomic data management will keep pushing the envelope as technology develops, opening doors to fresh perspectives on human health and illness and transforming customized medicine and precision healthcare techniques.
10. **Collaborative Approaches to Addressing Genomic Big Data Challenges**
Working together is essential to solving the intricate problems that come with handling large amounts of genetic data. We can better address these complex problems by using our combined knowledge, resources, and technological capabilities through the development of partnerships among researchers, institutions, and industry. Working together makes it possible to share methods, resources, and data, which speeds up the field of genomics research. By merging different viewpoints and skill sets, collaborations can promote innovation by creating cutting-edge approaches to organizing and interpreting enormous volumes of genetic data. Together, we can discover new information about human health and illness that would not be possible if we worked alone.
11. **Ethical Considerations in Managing Genomic Data**
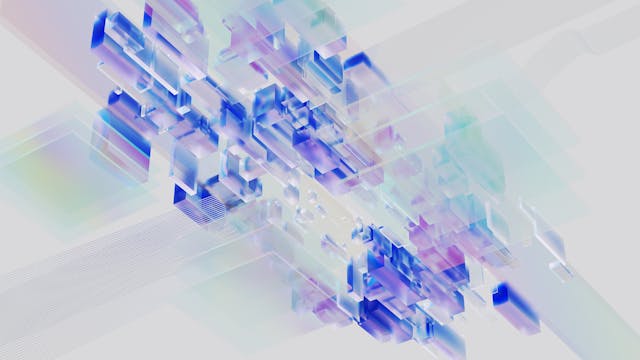
Because our genetic code contains sensitive information, ethical considerations are essential when managing genomic data. Concerns of consent, privacy, and possible abuse are raised while handling such data. Genetic information on an individual can provide insights into behavioral features, ancestry details, and even disease predispositions. Therefore, protecting this data is essential to avoiding discrimination, privacy violations, and moral conundrums.
A number of frameworks have been created to direct ethical procedures for managing genetic data. In these frameworks, the concepts of autonomy, beneficence, non-maleficence, and justice are frequently fundamental. In order to respect people's autonomy, it is necessary to have their informed consent for data collection and usage, making sure they are aware of the intended uses for their genetic information. Using genetic data to its fullest potential while reducing hazards to individuals or communities is known as beneficence. The goal of non-maleficence is to prevent harm from occurring from improper handling or illegal exposure of private genetic information. Lastly, equitable access to resources and benefit sharing from genetic data management are essential components of fostering justice in this field.
Assuring participants and stakeholders that all aspects of the process—from data collection and storage to analysis and sharing—are transparent is essential. The foundational elements of responsible genomic data management include defining precise policies regarding who can access genomic data, how it can be used, and guaranteeing compliance with laws like GDPR and HIPAA.
In addition to defending people's rights, ethical standards in the processing of genetic big data also provide an environment that is favorable to scientific progress without jeopardizing security or privacy. In order to fully utilize the promise of genomics research and prevent unintended repercussions or exploitation of sensitive genetic information, it is imperative to be alert about ethical considerations as technology in this field continues to progress quickly. 😷
12. **Conclusion**
In summary, efficient handling of the enormous volumes of data produced in genomics is essential to improving research results. In genomics research, the use of cutting-edge technologies such as blockchain, AI algorithms, and cloud computing can greatly enhance data exchange, analysis, and storage procedures. Researchers may solve issues with scalability, interoperability, and security in genomic big data management by putting strong data management strategies—like standardized formats, safe storage options, and efficient data sharing protocols—into practice. Accepting these novel approaches would improve researcher collaboration and speed up genomics discoveries by revealing important information from large, complicated datasets. Making effective genomic data management a top priority is essential to maximizing the promise of genomics research and generating significant discoveries that have the potential to transform precision medicine and healthcare.