1. Introduction
Data modeling plays a more important role now than it has in the big data era, as information is generated at an unparalleled rate. Data modeling is the process of putting data structures and relationships into a visual format so that large amounts of data can be handled accurately, consistently, and efficiently. Enterprises depend on data modeling to comprehend intricate datasets and get significant insights that propel decision-making procedures in the digital realm.
Building strong databases and analytical systems on top of data modeling enables businesses to efficiently manage and structure their data. Businesses can increase overall operational efficiency, improve data accuracy, and optimize data management procedures by utilizing modeling approaches like dimensional modeling and entity-relationship diagrams. Learning the craft of data modeling is crucial for businesses wishing to strategically use their information assets in a time when data is seen as the new money.
2. Understanding Data Modeling
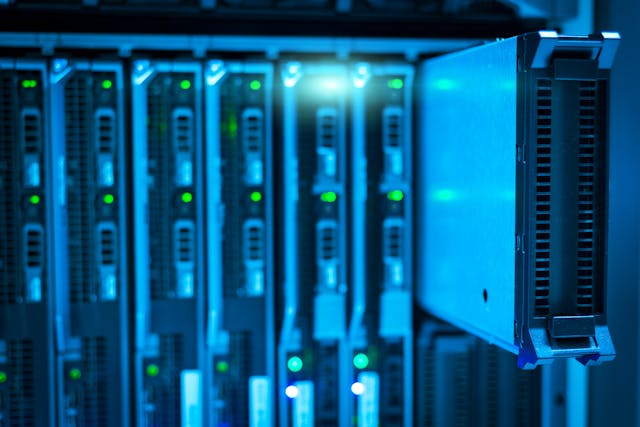
In the era of big data, comprehending data modeling is essential since it helps organize and structure enormous volumes of data. Creating a visual depiction of data structures to show how information is kept, accessed, and handled within an organization's systems is known as data modeling. This guarantees data efficiency, consistency, and integrity across several databases and aids companies in understanding the links between diverse data items.
In big data environments, several types of data models are commonly used to manage complex datasets effectively. These models include conceptual, logical, and physical models.
Conceptual Data Model: This high-level model defines broad concepts and their relationships. It provides a bird's eye view of the entire database without delving into technical details.
Logical Data Model: This model uses certain entities and characteristics to define the relationships between various data components. Its main goal is to define the database's structure without reference to the database management system.
The physical data model is a representation of the logical model that may be used with a particular database management system. Tables, columns, indexes, keys, partitions, and other features are included, and performance is optimized for effective storage and retrieval of huge datasets.
Organizations may efficiently manage their constantly expanding data volumes while maintaining information that is well-structured, readily available, and interoperable across several systems and platforms by utilizing these kinds of models in big data environments.
3. Improved Data Quality
Improving data accuracy and quality is largely dependent on data modeling. Data modeling aids in the standardization and clarification of data definitions by defining the framework that governs how data is arranged and related. This procedure guarantees accuracy, completeness, and consistency of data throughout an organization.
Data modeling helps to enhance the quality of data by pointing out mistakes or inconsistencies in the current database. Data modelers can address problems like duplicate entries, missing values, or improper linkages between several datasets by using this technique. Organizations can rely on more reliable information for their operations by using this technique to clean up the data.
Clearly defined business rules and data validations are established with the use of data modeling. Organizations can make sure that incoming data satisfies particular requirements before it is accepted into the system by specifying these rules inside the model. This proactive strategy aids in upholding high standards for the data that is saved.
Enhanced data quality has a direct effect on how decisions are made inside an organization. Decision-makers are better able to make decisions that result in better outcomes when they have access to trustworthy and dependable data. A retail organization that bases its sales forecasting accuracy on clean historical sales data, for instance, is probably going to make better decisions about inventory management than one that depends on incomplete or inconsistent information.
In a different case, a healthcare provider can provide more individualized treatments and care plans by utilizing precise patient data obtained from well-structured databases. Across a range of sectors and industries, investing in strong data modeling techniques improves decision-making ability.
4. Enhanced Data Analysis
In the big data era, data modeling is essential to improving data analysis procedures. Data modeling facilitates the organization and comprehension of complicated data sets by offering a systematic framework, which in turn speeds up the analytical process. By ensuring that analysts have access to relevant and well-organized datasets, organizations can save time and costs by using proper data modeling approaches.
Precise data modeling facilitates more effective handling of substantial amounts of data, resulting in enhanced analytical results. It makes it simple for analysts to find insights, linkages, and patterns in the data, which eventually leads to better decision-making. Case studies from different industries show how paying close attention to the specifics of data modeling produces forecasts that are more accurate and insights that can be put to use.
For example, a retail corporation noticed a considerable boost in the accuracy of their sales forecasting by carefully modeling its client transaction data. Through efficient data modeling techniques and appropriate relational database structure, they were better equipped to recognize consumer preferences and purchasing patterns. This resulted in more sales numbers because to focused marketing campaigns and tailored product suggestions.
Another example involves improved diagnostic capabilities for a healthcare company that applied comprehensive entity-relationship models to patient medical information. Better treatment choices and patient outcomes resulted from the organized presentation of patient data, which made it possible to retrieve pertinent information more quickly throughout the diagnosis process.
These case studies highlight how accurate data modeling, which facilitates effective data processing and perceptive pattern discovery, greatly affects the caliber of analytical outputs. Maximizing the value generated from large datasets requires using strong data modeling approaches in the era of big data and information overload.👡
5. Scalability and Flexibility
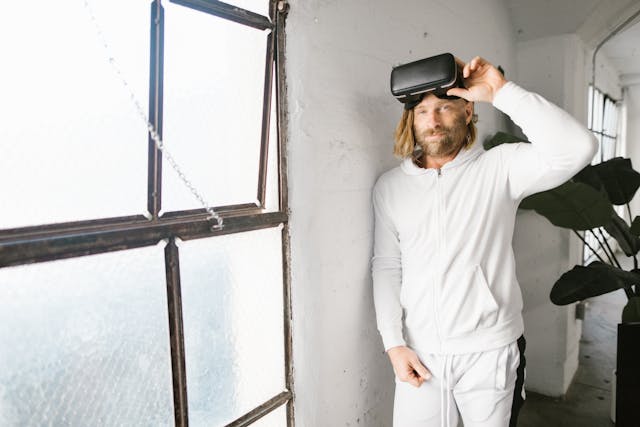
In the era of big data, scalability and flexibility are essential for managing the enormous volumes of data produced. As businesses expand, accurate data modeling is critical to their ability to scale their data infrastructure effectively. Businesses can seamlessly expand as data volume rises by adding additional data sources, fields, or tables to their well-designed data models without affecting already-running systems.💬
The advantages of flexibility become apparent when companies have to adjust to shifting consumer demands and corporate needs. A strong data model offers the flexibility required to make changes efficiently and promptly. Flexible data architecture makes it simple for enterprises to update and adapt their models to new information kinds and format changes, keeping their analytics current and applicable.
In summary, flexibility guarantees that organizations can remain adaptable and flexible in a quickly changing business environment, while scalability, achieved through appropriate data modeling, permits businesses to expand their data operations without difficulty. Accepting these advantages might provide businesses a competitive advantage by enabling them to successfully and efficiently utilize Big Data to its fullest.
6. Cost Efficiency
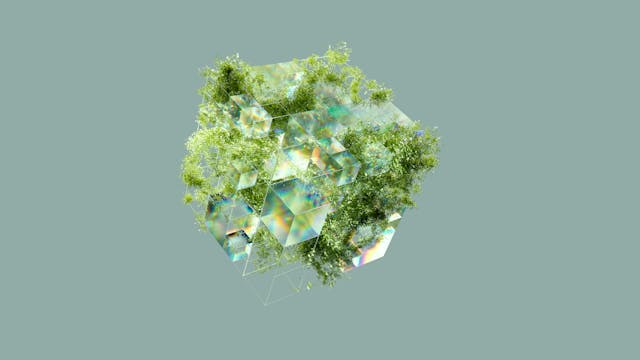
Organizations can save a lot of money in the long run by investing in data modeling. Businesses can boost productivity and save operating costs by streamlining their processes through the implementation of strong data modeling tactics. For example, businesses can reduce waste and overstock by using data modeling to enable precise forecasting and resource management, which lowers procurement costs.
Organizations can use data modeling to make well-informed decisions by using insights from structured data. As a result, marketing activities become more focused and less money is wasted on wide-ranging programs that might not have a big impact on sales. Businesses can better adapt their services and maximize sales while lowering marketing expenses by using data modeling approaches to identify client behavior trends.
Organizations can find inefficiencies and bottlenecks in areas like supply chain management by implementing data modeling. Through data-driven insights, firms may optimize logistics processes to save shipping costs and improve overall operational efficiency. By seeing possible problems before they worsen and preventing costly equipment breakdowns, predictive maintenance made possible by data modeling can reduce the cost of replacement and repair.
In summary, firms can gain a strategic edge in streamlining processes and reducing costs in a variety of organizational activities by utilizing data modeling in the big data era.
7. Better Decision-Making
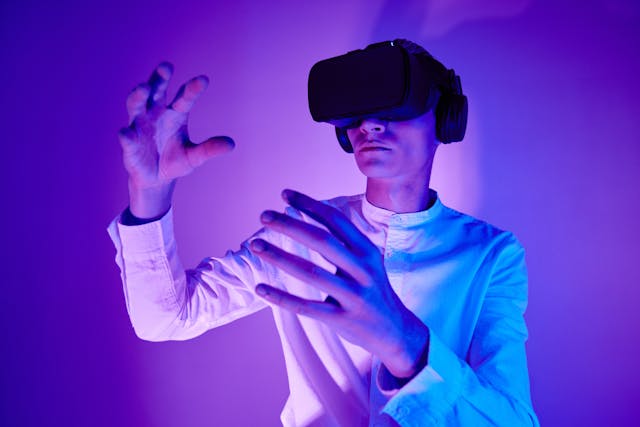
In the era of big data, data modeling is essential for improving decision-making procedures. Organizations can make decisions based on trustworthy insights by using precise and well-structured data models. These models let companies evaluate large, complicated datasets efficiently, which results in more accurate projections and strategic planning.
Businesses have used efficient data modeling to support well-informed decision-making in real-world situations. For example, a retail chain optimized product placement in stores using predictive analytics resulting from strong data models. Because popular items were positioned strategically to draw in the most customers, this resulted in higher sales.
In a similar vein, a financial institution found patterns of fraudulent activity by using advanced modeling tools to evaluate historical data. Early detection of suspicious transactions allowed the business to shield its customers' assets and avert possible losses. These illustrations highlight the revolutionary effect of data modeling in empowering organizations to make more informed decisions based on data-driven insights.
8. Regulatory Compliance
Adherence to standards like as GDPR and HIPAA is crucial in the era of big data. In order to make sure that regulatory requirements are fulfilled, data modeling is vital. By organizing data in accordance with these requirements, companies can quickly locate and retrieve the information required to prove compliance. Sustaining well-organized data models lowers the chance of non-compliance and associated penalties by optimizing sensitive data handling procedures. Strong data modeling improves an organization's overall data governance and security procedures in addition to making regulatory compliance easier.
9. Collaboration and Communication
Effective teamwork and communication are essential for success in the big data domain. Data modeling is essential for promoting communication amongst teams because it offers standardized models that act as a common language for all stakeholders. By guaranteeing that everyone is using and understanding data in the same way, this shared foundation improves collaboration.
Organizations can improve departmental collaboration by implementing standardized data models. For example, marketing teams can make better use of and comprehend customer data that the data analytics team has modeled in a consistent format. This makes decision-making procedures more effective and encourages a unified strategy for leveraging data insights throughout the company. Data modeling facilitates improved communication, which speeds up procedures and increases team efficiency.
10. Predictive Analytics
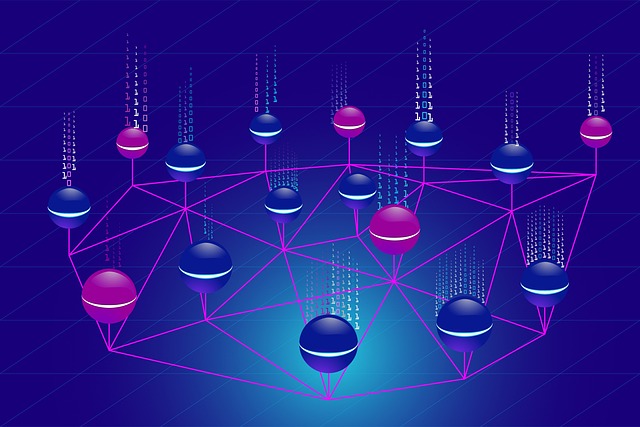
Robust data modeling provides the framework for predictive analytics to flourish. Predictive analytics can reach its maximum potential by using data modeling to create precise and well-defined structures. Effective data modeling approaches shape and improve the underlying datasets, which are crucial for the success of predictive analytics applications. These models provide predictive algorithms the structure they need to precisely predict events and glean insightful information.
In real life, companies use predictive analytics to forecast patterns in past data to predict future behaviors, trends, and events. Predictive analytics is used by e-commerce businesses, for example, to forecast client demand, optimize pricing tactics, and personalize recommendations. The precision and dependability of data models, which guarantee the relevance and integrity of the information being examined, enable these applications.😠
Through the adoption of data modeling as a fundamental component of predictive analytics initiatives, entities can gain significant understanding that facilitates well-informed decision-making and augments strategic planning. In today's data-driven world, investing in strong foundational frameworks through data modeling creates the framework for fully utilizing predictive analytics tools and technology.
11. Security and Privacy
In the big data era of today, the significance of data modeling for security and privacy cannot be overstated. Through the integration of secure design principles into the data modeling process, entities can proficiently protect confidential data from unapproved access and cyber hazards. This all-encompassing strategy guarantees that data is organized to minimize vulnerabilities and lower the likelihood of breaches.
Organizations are under increasing pressure to comply with strict requirements regarding data protection and privacy as a result of the implementation of privacy laws like the California Consumer Privacy Act (CCPA). Organizations can achieve these standards with the use of data modeling, which guarantees that databases are created with privacy in mind. Organizations may better protect user data, adhere to legal requirements, and uphold customer trust by modeling their databases appropriately.
To put it simply, including robust security protocols and privacy considerations into the data modeling process not only helps reduce the risks involved in handling sensitive data, but it also lays the groundwork for establishing reliable customer and stakeholder connections. In a time when data privacy is crucial, this proactive approach not only safeguards priceless data assets but also shows a dedication to moral behavior and legal compliance.
12. Conclusion
Taking into account everything mentioned above, we can say that using strong data modeling techniques has several advantages in the big data era. It simplifies data analysis, strengthens decision-making procedures, raises the standard and consistency of data, supports data governance, helps identify problems early on, and fosters teamwork. Together, these benefits help a business successfully navigate the broad waters of big data while increasing efficiency.🏧
Looking ahead, data modeling will become even more important as big data keeps growing at an exponential rate. The necessity for precise and well-structured data models increases when we consider the essential roles that emerging technologies like artificial intelligence (AI) and machine learning play in data analysis. Organizations will be able to extract valuable insights from their massive information repositories as data modeling will adapt to new kinds of data sources and intricate relationships within datasets.
Data modeling is expected to take on a dynamic and inventive future as it adjusts to the always shifting needs of big data analytics. Organizations may use data to drive strategic decision-making and achieve a competitive edge in today's fast-paced digital market by keeping up with these advancements and investing in strong data modeling practices.🫶