1. Introduction
Leveraging the power of data is essential for corporate success in today's data-driven environment. However, a shortage of committed data scientists causes many firms to struggle with utilizing this invaluable resource. In order to enable organizations to extract insights and make educated judgments without the requirement for specialized knowledge, data science technologies are used in this situation. These companies frequently struggle with finding patterns in big datasets, making accurate predictions about the future, and analyzing them. Organizations can overcome these obstacles and discover the potential hidden in their data with the help of data science tools.
2. Data Collection and Cleaning
Without the requirement for a trained data scientist, data science tools are essential in helping organizations with the data collecting and cleaning process. These technologies simplify the overall data collection process by efficiently gathering data from a variety of sources, including databases, websites, and APIs. They assist in cleaning the acquired data by identifying and removing errors, addressing missing values, and guaranteeing consistency in datasets.
The open-source tool Apache Nifi, which automates data flows between systems, Talend Data Fabric, which offers data integration and integrity functionalities, and Trifacta, a platform that specializes in data preparation by streamlining difficult tasks like cleansing and organizing varied datasets, are a few examples of tools that automate data collection and cleaning processes. These solutions improve decision-making processes' accuracy and dependability while helping firms handle their data more effectively.
3. Data Analysis and Visualization
For organizations, data science tools are essential for streamlining the analysis and visualization of large, complicated datasets. Without requiring data science skills, these tools allow people to explore data, spot trends, and extract insightful information. Businesses may simply construct interactive dashboards and visually appealing visualizations that enable intuitive understanding of data patterns by utilizing technologies such as Tableau, Power BI, or Google Data Studio. As a result, decision-makers are better equipped to base their decisions on lucid and succinct visual representations of the facts.
To help organizations extract relevant insights from their information, data science tools for analysis and visualization are mostly used for this purpose. With the help of these tools, users may effectively carry out a variety of analyses, including trend analysis, forecasting, clustering, and outlier detection. Businesses can find hidden patterns in their data by utilizing these technologies, which may not be seen using more conventional techniques. These tools' visualizations make it easier for teams inside an organization to share their findings with one another and make collaborative decision-making based on data-driven insights possible.
In essence, data science tools act as a bridge between raw data and relevant business outcomes by providing sophisticated capabilities for analysis and visualization that enhance the overall comprehension of complicated datasets. By using data-driven insights to drive strategic initiatives and maximize performance, businesses that fully utilize the potential of these technologies can acquire a competitive advantage.
4. Predictive Analytics
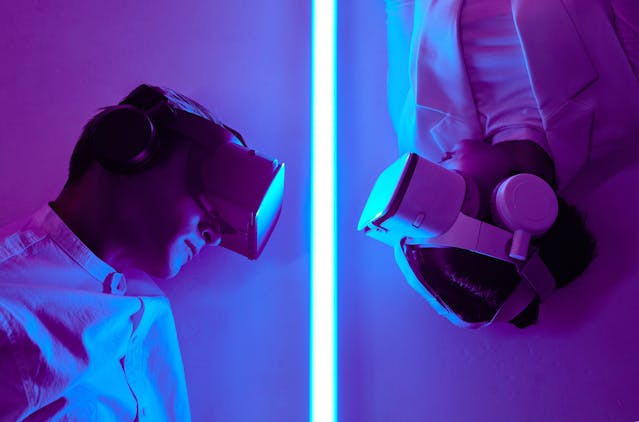
Tools for predictive analytics provide priceless insights to companies of all kinds. With the use of these technologies, businesses may effectively predict future trends and results by utilizing both historical and current data. Predictive models help businesses foresee consumer behavior, spot possible hazards, streamline processes, and make wise decisions.
The potential of predictive analytics technologies to improve marketing strategy is a strong selling point. Businesses can create customized marketing campaigns by grouping customers according to their preferences, purchasing history, and demographic data. Businesses may maximize their promotional efforts and increase consumer engagement and conversion rates by predicting client wants and behaviors.
Predictive analytics technologies are useful for organizations managing risk in the financial sector. Organizations can develop models that effectively identify prospective financial downturns or opportunities by evaluating market trends, historical data, and external factors effecting financial stability. Companies are able to take preemptive measures to reduce risks or take advantage of new market trends because to this foresight.
Supply chain management has undergone a transformation thanks to predictive analytics tools, which let companies predict demand more precisely. Businesses can minimize expenses related to stockouts and overstocking, manage inventory levels, and improve logistical operations by evaluating past sales data, market trends, and external factors like weather patterns or geopolitical events.
Predictive analytics technologies are essential for enhancing both operational effectiveness and patient outcomes in healthcare settings. Healthcare professionals can identify high-risk patients who might need extra care or treatments by evaluating patient data, medical histories, treatment outcomes, and demographic data. By projecting patient admission rates and bed occupancy levels, predictive models can also assist hospitals in allocating resources as efficiently as possible.
Predictive analytics technologies have been applied in a variety of industries, demonstrating their revolutionary effect on corporate decision-making procedures. Using data-driven insights to spur innovation and growth, predictive analytics enables firms to stay ahead of the curve in a variety of industries, including marketing, finance, supply chain optimization, and healthcare.
5. Automated Reporting
For companies trying to extract insights from their data without hiring data scientists, automated reporting is revolutionary. Reports can be automatically generated using data science tools on the basis of predefined metrics and parameters. These reports can offer insightful information on a range of company topics, including consumer behavior and sales patterns.
Excellent examples of reporting technologies that help expedite the automated reporting process are Tableau, Power BI, and Google Data Studio. With these platforms, users may use drag-and-drop interfaces to produce visually appealing and interactive reports without requiring sophisticated coding or analytical abilities. Businesses may generate reports more quickly, cut down on human error, and make data-driven choices more quickly by automating the process.
Imagine a retail shop utilizing Tableau to automatically generate daily sales data across different product categories. They may evaluate trends over time, visualize sales performance, and pinpoint areas for improvement with a few clicks—all without requiring manual interaction. In this sense, automated reporting enables companies to effectively utilize their data and maintain their competitiveness in the current fast-paced marketplace.
6. Market Segmentation
If companies want to effectively customize their marketing strategy, market segmentation is essential. Businesses can use data science technologies to evaluate enormous volumes of data and divide its clientele into discrete groups according to their preferences, behavior, or demographics. Through this method, organizations can develop marketing strategies that are specifically targeted to appeal to particular consumer demographics. Businesses can gain important information by using these technologies to better understand their clientele and anticipate their demands.
Using data science technologies for market segmentation greatly improves consumer engagement and retention. Businesses can tailor their offerings and messaging to each group's distinct tastes by segmenting their consumer base. The consumer experience is improved by this tailored approach, which raises engagement and loyalty. Businesses may identify high-value consumers and put successful retention strategies in place by using data-driven segmentation. Businesses can maximize their marketing efforts and create enduring client relationships by utilizing data science methods for market segmentation.
7. Sentiment Analysis
Tools for sentiment analysis are essential for helping organizations understand client comments. To determine the attitude underlying the words, these systems comb through enormous volumes of text data from numerous sources, including surveys, social media, and reviews. Businesses can gain important insights into the attitudes, preferences, and pain areas of their customers by studying this data.
Sentiment analysis finds use in many different areas in the current digital era. Understanding client attitudes in e-commerce can help with product development by pointing out characteristics that customers find appealing or areas that want improvement based on feedback that is not so good. Sentiment analysis is used in the hospitality industry to help hotels and restaurants track customer feedback, improve service, and quickly resolve issues. Analyzing patient feedback in the healthcare industry can help improve services for better experiences and results for patients.
Businesses can have a more profound comprehension of the emotions and perspectives of their target audience by utilizing sentiment analysis techniques. This helps businesses to make well-informed decisions that match the expectations and preferences of their customers, which eventually raises customer happiness and loyalty across a range of industries.
8. Text Mining
Data science tools offer a potent capacity called text mining that can be quite advantageous to enterprises. Businesses can use text mining to glean insightful information from vast amounts of textual data, including emails, social media posts, customer reviews, and more. These technologies examine text data using natural language processing techniques to find moods, patterns, and trends that human analysts might not notice right away.
Text mining technologies are a valuable tool for businesses to monitor brand sentiment on social media platforms, uncover developing trends in the industry, better comprehend customer feedback, and discover potential risks or difficulties early on. Businesses can use sentiment analysis to determine how the public feels about their goods and services and use that information to guide actions that will increase consumer satisfaction. Additionally, text mining is essential for automating processes such as classifying support tickets or retrieving pertinent data from unstructured documents.
Text mining enables companies to extract valuable insights from the abundance of information concealed in large volumes of textual data, thereby facilitating strategic decision-making and optimizing operational efficacy. Businesses can obtain a competitive advantage in the data-driven business environment of today by adding text mining capabilities to their toolkit of data science capabilities.
9. Fraud Detection
Tools for data science are essential for supporting fraud detection systems in companies. Through the use of sophisticated algorithms and machine learning models, these technologies are able to go through enormous volumes of data in order to find abnormalities or irregular patterns that can point to fraud. They are able to continuously monitor data in real-time and promptly notify organizations when they identify questionable activity.
The capacity of data science tools to adapt and learn from new fraud trends is a significant benefit when employing them for fraud detection. By adapting to new risks, these solutions help firms stay one step ahead of fraudsters. Through automation of the fraud case flagging process, organizations can drastically cut down on the time and resources needed for manual investigations.
Through the use of predictive analytics, data science tools give organizations a proactive way to combat fraud. These technologies estimate possible hazards and assist organizations in implementing preventive steps to lessen the effect of fraudulent activity by examining previous data and trends. In addition to improving security, using data science methods for fraud detection also protects a company's finances and reputation.
10. Customer Behavior Analysis
The utilization of data science techniques is essential for firms to thoroughly and precisely analyze consumer behavior trends. By using these capabilities, firms can acquire significant information into client preferences, purchase behaviors, and interactions. Businesses can effectively modify their tactics and make decisions that resonate with their target audience by having a thorough understanding of these characteristics.
Businesses can gain a competitive advantage by analyzing customer behavior, which highlights patterns and trends that might otherwise go missed. Businesses may improve customer experience, increase engagement, and personalize marketing campaigns by using data science tools to segment customers based on their activities. Businesses can use this type of analysis to predict client demands, pinpoint areas for development, and create campaigns that are relevant to particular customer segments.
Businesses can better satisfy customer needs by optimizing their offerings by having a thorough grasp of how customers engage with their products or services. Organizations may track client journeys, identify purchase process pain areas, and improve user experience overall with the use of data science tools. With this kind of understanding, companies can make data-driven decisions that improve customer happiness and loyalty and build lasting relationships with their customers.
11. Recommendations Engine
Data-driven recommendation engines are essential for increasing revenue and enhancing customer happiness for companies. By evaluating user behavior and preferences, these technologies can deliver personalized recommendations that boost the possibilities of conversion. Consider the colossus of e-commerce, Amazon. Their advanced recommendation system makes product recommendations based on user behavior and browsing history, which increases sales and improves user engagement.❠️
Recommendation engines are used by entertainment services such as Netflix to make personalized movie and TV show recommendations based on user viewing preferences. Overall subscriber satisfaction and audience retention have grown dramatically as a result of this. Similar to this, data-driven recommendation algorithms are used by music streaming services like Spotify to construct playlists based on listener history and likes, providing customers with a personalized music experience that keeps them interested.
Recommendation engines are used by businesses such as Flipboard in the online news and content consumption space to tailor article suggestions according to readers' interests. This increases traffic and ad income for publishers in addition to improving user engagement. Data science-powered recommendation engines have shown to be extremely useful tools for improving customer experiences and accelerating business growth in a variety of industries.
12. Conclusion
After putting everything above together, we can say that even companies without professional data scientists can profit greatly from using data science technologies. Businesses may make wise judgments, streamline processes, and get insightful information from their data by utilizing these technologies. In today's data-driven business environment, this transformative potential not only increases overall competitiveness and strategic decision-making, but also operational efficiency.
By adopting data science technologies that enable them to realize the full potential of their data assets, businesses can acquire a competitive advantage. By integrating these tools into routine business activities, one may optimize workflows, spot patterns, reduce risks, and seize opportunities that might otherwise go missed. The potential of data science tools to democratize data analytics and deliver useful information that promotes corporate success is what makes them valuable.
Adopting data science tools necessitates a calculated approach to incorporating these potent resources into current workflows rather than a specialized team of data scientists. Businesses may use data-driven insights to stay creative, adaptable, and ahead of the curve in a market that is becoming more and more competitive by putting the correct tools in place. Businesses may create the conditions for long-term success and sustainable growth by utilizing data science tools to improve operational efficiency and make well-informed decisions.