1. Introduction
Medical errors are a recurring problem in the healthcare sector, with serious repercussions including patient injury, higher healthcare expenses, and legal repercussions. Error rates in medicine remain concerning despite advancements in technology and protocols. A growing number of professionals are looking to machine learning as a potential solution to reduce medical errors and improve patient safety because they understand how urgent it is to address this problem.
The creation of algorithms for machine learning entails the analysis and learning of intricate data patterns in order to generate predictions or judgments devoid of explicit programming. Machine learning has demonstrated significant promise in the field of healthcare, particularly in the areas of risk factor identification, outcome prediction, treatment plan optimization, and diagnostic accuracy improvement. Through the utilization of artificial intelligence and data analytics, machine learning presents a new method for identifying problems prior to their occurrence or quickly addressing them once they do. We will examine how machine learning technology can significantly lower the rate of medical errors and transform patient care in this blog article.
2. Understanding Medical Errors
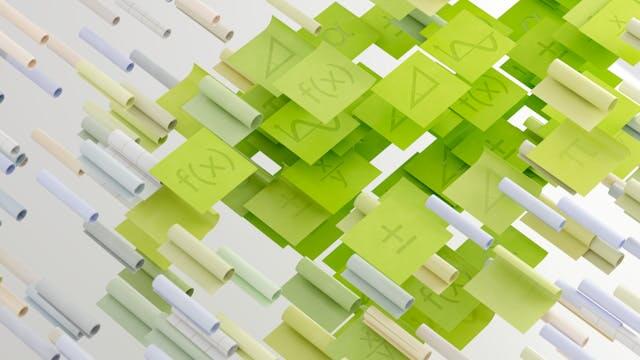
Medical errors are described as avoidable adverse occurrences or mistakes in the provision of healthcare that have the potential to cause patients harm. These mistakes have a major effect on patient outcomes, resulting in longer hospital stays, higher rates of morbidity and mortality, and lower patient quality of life. Beyond the human cost, medical errors cause significant financial losses for the healthcare system in the form of unpaid bills, extra treatments, and missed productivity.
Medication errors (using the incorrect dosage or drug), diagnostic errors (missing or delayed diagnosis), surgical errors (operating at the incorrect spot), communication errors (misinterpreting information), and system failures (absence of protocols or safeguards) are common medical error categories. These mistakes frequently have a number of root reasons, including poor coordination of care, structural problems in healthcare facilities, staff exhaustion or burnout, misunderstanding among healthcare providers, and insufficient training or supervision. It is essential to comprehend these underlying causes in order to put appropriate measures in place that will lower medical errors and enhance patient safety.
3. The Potential of Machine Learning in Healthcare
Massive datasets can be processed and analyzed by machine learning algorithms at speeds and scales much faster than those of a human. Through the effective identification of patterns, anomalies, and correlations in these datasets, machine learning can reveal important information that could otherwise lie hidden. Given the everyday generation of enormous volumes of patient data in the healthcare industry, this skill is extremely important.
Machine learning has demonstrated its potential in a number of applications in healthcare settings. Interpretation of diagnostic imaging is one prominent example. With the use of machine learning models, radiologists may diagnose patients more quickly and accurately by identifying abnormalities in medical images like CT, MRI, and X-rays. Machine learning-powered predictive analytics can predict patient outcomes, enabling healthcare practitioners to take early action to avoid issues.
Personalized medicine relies heavily on machine learning, which analyzes genetic data to customize therapies based on each patient's own genetic composition. This targeted approach lowers the risk of negative medication reactions while simultaneously improving therapeutic efficacy. The healthcare industry has witnessed the successful implementation of machine learning, indicating its potential to transform medical procedures and significantly enhance patient outcomes.
4. Current Challenges in Reducing Medical Errors
A vital objective in the complex world of healthcare is continuing to lower the rate of medical errors. Progress in this area is hampered by a number of factors, such as poor communication between healthcare providers, disjointed healthcare systems that result in subpar care coordination, a lack of standard operating procedures for reporting and analyzing errors, and the complexity of human factors in medical decision-making.
The limitations of traditional approaches to mistake prevention prohibit them from effectively addressing the complex nature of medical errors. Techniques like root cause analysis frequently ignore more significant organizational and procedural flaws in favor of concentrating on individual mistakes as opposed to systemic problems. Due to the reactive character of many traditional techniques, opportunities for proactive preventative actions are missed and interventions are made after errors have already had an impact on patients.
By offering sophisticated analytical tools to spot patterns and trends in medical data that human analysis could miss, machine learning holds promise for resolving these issues. Predictive analytics can assist anticipate possible errors before they occur, enabling healthcare providers to implement targeted interventions and prevent patient damage proactively. It does this by applying machine learning algorithms on massive amounts of healthcare data. Real-time patient data stream monitoring can be facilitated by machine learning techniques, which can also be used to identify abnormalities or departures from established protocols that require rapid attention.
5. Role of Machine Learning in Preventing Medical Errors
By using cutting-edge algorithms to recognize trends, anticipate possible hazards, and proactively prevent errors, machine learning plays a critical role in preventing medical errors. With the use of this technology, healthcare professionals can quickly and accurately analyze large amounts of data, empowering them to make more educated decisions that will improve patient safety.
Predictive analytics is one way machine learning can help decrease medical errors. Through the examination of patient data, including demographics, test results, and medical history, machine learning models are able to forecast possible dangers for specific patients. These forecasts enable medical personnel to take proactive steps and act before major problems develop.
Healthcare systems can benefit from the integration of machine learning algorithms, which can improve patient safety and streamline procedures. For example, these algorithms can help with medication management by identifying possible drug interactions or mistakes in dosage before patients receive medication. By being proactive, bad outcomes associated with drug problems can be avoided.
Automating the analysis of medical imaging scans, such MRIs and X-rays, is possible with machine learning. These algorithms use image recognition technology to help radiologists find anomalies more quickly and precisely, which can result in prompt patient diagnoses and treatment strategies.
Clinical decision-making can be aided by machine learning systems, which offer real-time insights derived from continuously updated data. These insights support healthcare professionals in staying up to date on evolving patient situations and in making timely, well-informed decisions that reduce errors and enhance results.
To sum up what I've written thus far, incorporating machine learning into healthcare systems has a lot of potential to enhance patient safety through pattern recognition, risk prediction, and proactive error prevention. Through the use of sophisticated algorithms and data analytics tools, machine learning enables medical personnel to provide more individualized care while reducing the risk of medical errors.
6. Ethical and Regulatory Considerations
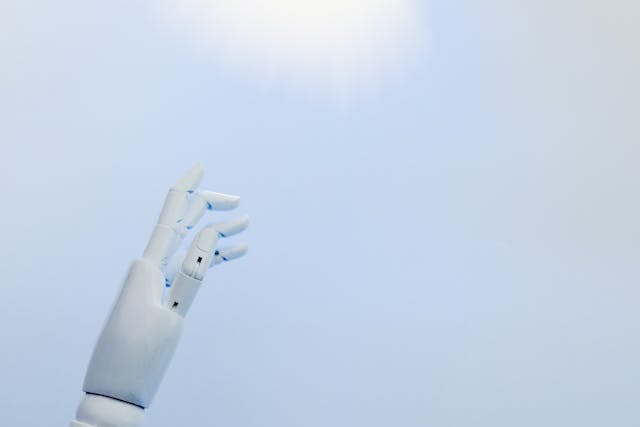
Using machine learning to inform healthcare decisions brings up a number of ethical issues that need to be properly considered. The possible loss of human control and accountability when important choices are left to algorithms is a major source of concern. For consumers and healthcare practitioners to comprehend how these systems reach their decisions, algorithmic decision-making must be transparent.
The problem of bias in machine learning algorithms is another ethical one. These algorithms are trained on preexisting data, which may be biased by nature and produce unfair results, especially in the medical field where decisions that have the capacity to change a person's life are made. It is critical to guarantee justice and equity in algorithmic decision-making processes to prevent escalating already-existing inequalities in the provision of healthcare.
To protect patient safety and privacy of data, the medical industry is governed by strict laws and regulations. A number of nations have begun to enact certain laws pertaining to the application of artificial intelligence (AI) technology in healthcare environments. For example, the FDA in the US has issued guidelines for the development, validation, and regulation of AI-based medical devices.
The use of patient data for machine learning model training is mostly regulated by laws, such as the General Data Protection Regulation (GDPR) in Europe. In order to preserve patient privacy and sustain confidence in AI-driven healthcare solutions, compliance with these standards is imperative. Policymakers are constantly updating rules to keep up with the changing field of AI applications in medicine as a result of the rapid advancement of technology.
7. Implementation Challenges and Solutions
Healthcare organizations encounter difficulties incorporating machine learning into their current infrastructure, primarily because of data silos, interoperability problems, and opposition to change. Comprehensive analysis is impeded by data silos, which limit departmental access to vital information. Different data formats and standards across systems create interoperability difficulties that impede smooth communication and integration. Concerns about job security or a lack of knowledge about the advantages machine learning can provide can be the root of resistance to change.
Interoperability protocols and data standards are two tactics that could help remove these obstacles and improve system-to-system information sharing. Implementing comprehensive training initiatives to educate healthcare personnel on the uses and advantages of machine learning may assist in reducing opposition to the technology. Addressing issues and guaranteeing a seamless integration process also depend on IT departments and clinicians having clear lines of communication.
When addressing the challenges of incorporating machine learning algorithms into healthcare settings, creative solutions are crucial. Healthcare organizations may effectively leverage machine learning to lower medical errors and enhance patient outcomes by encouraging stakeholder engagement, emphasizing data security and quality, and offering continuous support and education.
8. Case Studies: Machine Learning Success Stories
Case studies are effective means of demonstrating how machine learning may enhance healthcare results. One such instance is the work carried out at Massachusetts General Hospital, where patient data was analyzed using machine learning algorithms to find patterns that could indicate which patients were more likely to acquire sepsis. The early identification accuracy of the algorithm resulted in a noteworthy reduction in the death rates related to this illness.
Another noteworthy example study is from Stanford University, where researchers analyzed retinal scans using machine learning to identify diabetic retinopathy. This novel strategy made it possible to diagnose the disease more quickly and accurately, which improved the prognosis of diabetes patients undergoing therapy.
The significance of high-quality data input, ongoing algorithm improvement, and interdisciplinary cooperation between data scientists and healthcare practitioners are among the lessons acquired from these successful deployments. Healthcare organizations may greatly improve patient care and safety while also lowering medical errors by utilizing machine learning to its full potential.
9. Future Directions and Opportunities
There is a great deal of room for technological progress in investigating potential future directions and prospects in using machine learning to remedy medical mistake difficulties. The creation of increasingly complex algorithms that are better able to anticipate and stop possible mistakes before they happen is one promising direction. Improved predictive analytics may offer real-time information to medical personnel, allowing for proactive risk-reduction measures.
Advanced AI technologies like deep learning and natural language processing (NLP) could greatly enhance therapy recommendations and diagnostic accuracy as the field develops. Through process simplification, a decrease in human error, and eventually improved patient outcomes, these advances have the potential to completely transform the healthcare industry.
In terms of medical error prevention, new developments in AI-driven healthcare are expected to have a significant impact. Wearable technology and Internet of Things (IoT) integration may allow for ongoing patient monitoring, which would make it easier to identify abnormalities early and take preventative action. Big data analytics and machine learning algorithms work together to reveal trends hidden in large datasets, which can result in more individualized treatment plans catered to the needs of each patient.
Prioritizing patient safety and privacy while embracing these cutting-edge technologies appropriately is essential as we traverse the convergence of machine learning and healthcare. We can harness the transformative power of artificial intelligence (AI) to generate substantial progress in decreasing medical errors and improving the delivery of high-quality healthcare by promoting collaborations between data scientists, policymakers, and healthcare practitioners.
10. Collaborative Approaches: Human-Machine Partnership
Promoting collaboration techniques between healthcare practitioners and AI systems stands out as a crucial answer in tackling the rising medical error rate problems. By highlighting the importance of this collaboration between humans and machines, we can greatly improve mistake avoidance techniques. By fusing the computing power, data processing capabilities, and pattern recognition abilities of AI systems with the empathy, creativity, and critical thinking skills of healthcare professionals, this partnership can maximize the strengths of both sides.
The mutually beneficial association between humans and machines presents significant opportunities for enhancing the quality of medical care. Healthcare practitioners can use AI technologies to quickly evaluate large amounts of data and find insights that could be difficult for people to find on their own. Together with AI technologies, clinicians may make better decisions that result in more accurate diagnoses, individualized treatment regimens, and increased patient safety.
Through process streamlining within healthcare settings, this collaboration can lessen administrative workloads for medical staff, freeing them up to concentrate more on providing direct patient care. AI systems can be useful tools that complement human abilities rather than taking their place with efficient cooperation. A future in which medical errors are reduced, patient outcomes are maximized, and total healthcare quality is raised to unprecedented levels is made possible by the cooperation of human knowledge and technological efficiency.
11. Cost-Benefit Analysis: Impact on Healthcare Economics
The financial aspects of the healthcare sector will be significantly impacted by the use of machine learning in the field to reduce medical errors. Healthcare practitioners may be able to reduce expenditures related to avoidable errors, incorrect diagnoses, and needless procedures by utilizing AI technologies. Completing a comprehensive cost-benefit analysis is essential to comprehending how these technologies will affect your bottom line.
Examining the cost-saving possibilities shows that healthcare organizations have a lot of room to cut costs overall, enhance patient outcomes, and streamline operations. By lowering mistake rates, increasing productivity, and optimizing resource allocation, the initial investment made in integrating machine learning technologies can yield significant long-term savings when the return on investment is assessed. In addition to being highly persuasive, the financial advantages of applying AI to healthcare settings are crucial for long-term cost control and high-quality patient care.
12. Conclusion:
In summary, machine learning has the potential to reduce high rates of medical errors because of its capacity to evaluate large volumes of data and spot patterns that people might miss. Patient safety can be greatly increased by incorporating machine learning techniques into healthcare settings, even though they are not a perfect answer to every mistake.
It is imperative that authorities make investments in AI research and applications in the healthcare industry. Important measures to think about include creating explicit rules for the integration of machine learning systems, protecting data privacy and security, and encouraging openness in algorithmic decision-making.
Rather than replacing human expertise, healthcare providers should embrace AI technologies as tools to improve their clinical practice. Maximizing the advantages of machine learning for error reduction will require staff training on how to utilize these tools effectively and ongoing support.🗓
Through the creation of reliable machine learning models customized for certain healthcare scenarios, researchers play a critical role in the advancement of the discipline. Innovative solutions to specific problems in various medical specializations can be developed through partnerships between data scientists, doctors, and legislators. Stakeholders may use AI to establish a safer and more effective healthcare system for everyone by cooperating.